A Financial Primer on a New International AI Organization
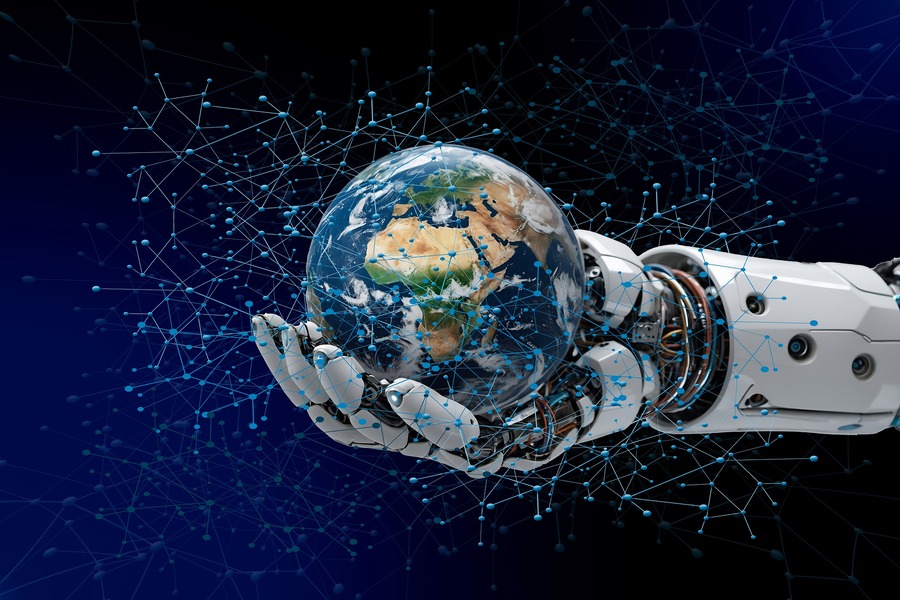
Published by The Lawfare Institute
in Cooperation With
The idea that global artificial intelligence (AI) challenges require global solutions has sparked numerous proposals by researchers, policymakers, and industry leaders for a new international AI organization. However, these proposals often neglect a crucial element: funding. With some proposals calling for a total budget exceeding $100 billion, resolving questions of funding should be a top priority.
International organizations (IOs) live and die by their funding mechanism. An organization’s donors greatly influence its mission, while the reliability of its funding affects its ability to plan long-term projects and pursue that mission independently of its donors’ interests. Consider the World Health Organization (WHO): Over 75 percent of its annual budget comes from voluntary contributions, which can be withdrawn at any time. This financial uncertainty has led to criticisms that the WHO is more focused on pleasing donors than protecting global health. Without a solid funding plan, even the most enthusiastic supporters of a new AI IO—whether it is focused on governance, research, or benefits sharing—will struggle to act. Or worse, they will act on unfunded proposals, wasting both financial and political capital.
Effective financial planning is therefore necessary to create a successful international organization for AI. To this end, I review the budgets of 20 existing IOs to estimate the cost of an AI IO based on the function it performs and offer five principles for designing a funding mechanism:
The method for calculating mandatory assessment amounts should align the incentives of the AI IO and its members.
The funding mechanism should offer members flexibility if they are unable to pay their full membership fees, while still authorizing penalties to aid the IO with enforcement.
An AI IO should be able to perform its core functions if all voluntary contributions were withdrawn.
An AI IO should implement formal policies for handling earmarked voluntary contributions.
An AI IO should defray the cost of administering programs through self-funding activities.
How Much Would a New International AI Organization Cost?
The budgets of existing international organizations—though imperfect proxies for one focused on AI—can serve as a general reference for estimating the cost of a new international AI organization. Based on a review of 20 prominent IOs, the potential range of costs is vast. The Intergovernmental Panel on Climate Change (IPCC) has a $10 million annual budget, while member states spend $3 billion each year on the International Space Station (ISS). Why is the range so broad? IOs performing different functions have different requirements: An international AI organization gathering information on the state of AI development would have substantially fewer expenses than one promoting joint research collaborations. Table 1 lists recent annual budgets of 20 IOs, separating them into six categories based on function (as suggested by AI researchers Maas and Villalabos), as well as an estimated annual cost of an international AI organization according to its function.
These figures illustrate just how widely the cost of an AI IO might vary based on its function. Considering the substantive differences between an AI IO and existing IOs performing the same function is relevant to policymakers for two main reasons. First, it helps highlight when the budgets of existing IOs are a useful reference for an IO focused on AI. Second, it helps identify when a lack of funding is a barrier to an IO’s success: We too easily assume that an IO would be more successful if only it had more resources, overlooking other barriers, such as lack of political will or concerns that sensitive information will be leaked.
Scientific consensus-building (~$5M-$50M): There is currently little scientific consensus about the state of AI research, development, and risks. An international AI organization akin to the IPCC, as Kevin Frazier has argued, could help build scientific consensus by aggregating and standardizing scientific knowledge. However, since AI capabilities (and risks) have been evolving more rapidly than climate research, AI-related reports would need to be updated more frequently than IPCC reports, which are published every five to seven years. Consequently, the IPCC’s volunteer model, which climate experts already describe as “intense, stressful, and unsustainable,” is less viable for an AI IO, which will therefore need a larger budget to pay experts for their contributions. Additionally, unlike climate change forecasts, which are supported by sophisticated models of the global environment, predictions about the future of AI development are highly variable and subjective. If an international AI organization struggles to predict future AI capabilities and risks, the epistemic difficulty of the task suggests that more funding would not be the solution.
Political coordination (~$50M-$500M): Due to the geopolitical importance of AI, countries may resist ceding decision-making authority to a centralized IO, impeding efforts to coordinate standards-setting and policymaking. This difficulty explains why some countries have opted for softer collaboration through a decentralized network of AI safety institutes (AISIs), which share information with each other unconstrained by a consensus requirement. If countries disagree about an AI organization’s priorities, mandate, or size, they can unilaterally decide what the organization should focus on and how much funding to provide it. For example, the U.K. AISI, with a budget of £100 million (~$132 million), does everything from setting standards to awarding research grants, while the U.S. AISI, whose budget is $10 million, focuses more narrowly on model evaluations. This decentralized approach to AI global governance, however, currently lacks formal coordination procedures, limiting its effectiveness among countries that do not already collaborate. Introducing these coordination procedures would require overcoming geopolitical barriers, which additional funding is unlikely to help accomplish.
Standards enforcement (~$50M-$500M): The IAEA is the most cited example of an existing IO that develops and enforces standards. Yet unlike nuclear weapons, which only a few countries build and possess, AI models are being developed by many different companies, research organizations, and governments, making it much harder to enforce standards and verify compliance. There are just too many actors involved. This problem could be resolved by targeting regulations at the most capable foundation models, which are developed by only around 40 of the world’s most highly resourced companies—but this kind of regulation might simply advantage incumbents by raising the barriers to entry for new companies aiming to build advanced AI models, stifling competition. Alternatively, designing regulations for the hardware needed to create AI, what researchers have called “compute governance,” would also make regulation and enforcement more feasible, but the extreme concentration of the AI hardware supply chain among the Japanese, Dutch, and Americans disincentivizes these countries from ceding much control to a multilateral IO. As a result, political feasibility likely represents a larger barrier to this type of IO’s success than funding. Why would a nation—especially one worried about the geopolitical importance of AI—defer to an IAEA for AI when it and a few allies can set standards and write export controls?
Systemic risk management and crisis response ($100M-$1B): The recent CrowdStrike outage demonstrates how technologies can be so embedded within the global system that they are systemically important. As AI models are integrated into critical private and public systems, an AI IO may need to hold widely adopted models to stricter standards, especially in terms of reliability, to avoid a CrowdStrike-style outage. Advanced AI systems may also create other types of risks, such as facilitating large-scale cyberattacks or the creation of sophisticated bioweapons. Responding to such a wide range of potential harms likely requires sector-specific solutions (e.g., WHO has more baseline expertise for responding to global health crises), so some funding should still be allocated toward existing IOs.
Joint research initiatives ($1B-$10B): AI computing resources and talent are in such high demand that even countries working together might be priced out of certain initiatives. A goal of matching the private sector in computing resources and infrastructure just might not be achievable, especially with tech giants planning to spend hundreds of billions of dollars on new AI data centers. A narrower goal, however, of providing enough funding to allow researchers outside of industry to produce cutting-edge research is far more realistic: The estimated budget for the United States’s National AI Research Resource (NAIRR) is $2.6 billion spread over six years, while researchers at the Allen Institute for AI (AI2) produce cutting-edge research and spend only $30 million each year on computing resources. Alternatively, an AI IO might exclusively fund research on the risks of AI. This narrower research initiative would likely cost less than a full-scale “CERN for AI” because researching AI risks often requires securing only enough computing resources to test and fine-tune existing models, rather than enough to train a foundation model from scratch.
Benefits sharing ($1B-$10B): With global health, sharing benefits often requires purchasing expensive medical supplies (i.e., vaccines and diagnostic equipment), which explains the multibillion-dollar budgets of GAVI and the Global Fund. By contrast, AI benefits sharing can be much more cost efficient, ranging from subsidizing access to the most advanced models to lightly fine-tuning models for local contexts. Yet a benefits-sharing AI IO will face additional hurdles related to proprietary information and infrastructure. For the former, AI companies worry that other companies will use access to their models to train competitors and are reluctant to share too much with third parties. For the latter, some regions will require additional infrastructure, such as reliable internet access, to realize the full benefits of AI. Investing in such infrastructure would quickly drive up the total costs of a project.
How Should We Fund a New International AI Organization?
An IO’s budget comes from three main types of funding: mandatory assessments from members, voluntary contributions from government or non-state actors, and self-funding activities. Each source comes with trade-offs: Mandatory assessments provide stability but are hard to secure. Voluntary contributions offer flexibility but risk donor influence. Self-generated revenue can help but rarely covers all costs. I consider these trade-offs and offer five principles for designing an effective funding structure for a new international AI organization.
Principle 1: The method for calculating mandatory assessment amounts should align the incentives of the AI IO and its members.
Mandatory assessments, however valuable they may be from an IO’s perspective, are politically unpopular because members dislike recurrent expenses outside their direct control. Generating buy-in will require clearly demonstrating an IO’s value by calculating assessments in a way that aligns the incentives of the members and the IO. The funding structures of the IMO and WTO provide two good examples of incentive alignment. The IMO calculates assessments based on the tonnage of a member state’s merchant fleet, while the WTO uses the amount of a member’s international trade relative to other members to calculate assessments. In both cases, the more a member benefits from its involvement in an organization, the more it is expected to contribute financially. An AI IO should have a similar aim, adjusting contributions using criteria relevant to the organization’s function: For example, a joint research initiative might calculate contributions according to the number of researchers or papers published by researchers from each member country. Under this system, Italy, whose citizens account for 16.1 percent of all CERN personnel, would contribute more than Germany, whose citizens account for 9.3 percent of CERN personnel, even though Germany’s gross domestic product is larger. If implemented, this funding mechanism would also need to cap members’ assessed contributions and develop meritocratic hiring processes to avoid gamesmanship by members. An AI IO might disentangle these funding obligations from voting rights to promote broader representation from developing countries. For example, CERN adopts a “one member, one vote” model, regardless of the amount of member states’ contributions.
Principle 2: An AI IO’s funding mechanism should offer members flexibility if they are unable to pay their full membership fees, while still authorizing penalties to aid the IO with enforcement.
In 2011, during its sovereign debt crisis, Greece was faced with either defunding its domestic research institutes or reneging on its membership fees for CERN. Choosing the former would have effectively halted research within the country, while choosing the latter would have undermined important cross-border research collaborations. CERN negotiated a new payment plan that temporarily suspended Greece’s membership fees, allowing it to continue funding domestic research institutes without being expelled from CERN. An AI IO must have enough financial cushion to offer members flexibility if they cannot pay their full membership fees. Otherwise, it risks disenfranchising poorer countries whose input is essential to the broader legitimacy of the organization.
But offering payment flexibility does not mean letting members pay whenever they want. An IO must also have sufficient enforcement capabilities to incentivize members to either pay or negotiate some payment plan. These mechanisms typically involve an IO withholding some privilege of IO membership, such as suspending voting rights (as the United Nations did with Lebanon in 2023) or pausing benefits like technical assistance (as the ITU does for nonpayment of membership fees). Depending on its function, an IO for AI’s enforcement mechanisms might include suspending access to scientific reports, political meetings, model evaluations, emergency assistance, compute resources, or any other benefits provided by the AI IO.
Principle 3: An AI IO should be able to perform its core functions if all voluntary contributions were withdrawn.
In recent decades, voluntary contributions have accounted for an ever growing share of IOs’ budgets, with some being entirely or almost entirely funded by such contributions. For example, 95 percent of the International Organization for Migration (IOM)’s 2024 budget came from voluntary contributions. Although these contributions, much like decentralized AI safety institutes, provide members with flexibility if they disagree about the proper size and focus of an IO, overreliance on contributions that depend on continued donor approval risks paralyzing an organization. For example, some observers felt the WHO was too slow to declare the coronavirus pandemic a global health emergency out of fear that it would upset China and lose the country’s voluntary contributions. An AI IO, in particular, needs a reliable source of funding that doesn’t depend on members’ continued goodwill because it will likely need to make decisions that upset its members. Considering the geopolitical importance of AI and geopolitical tensions between the U.S. and China, any decision that appears to shift the balance of power in one country’s favor will likely upset the other. If an AI IO does not maintain enough financial independence through mandatory assessments and self-funding activities, it risks giving members that it is very likely to upset the power to disrupt its core functions. This is a recipe for creating a paralyzed, ineffective organization. Voluntary contributions, however, are more acceptable for funding peripheral functions like one-off reports, which are unrelated to an AI IO’s existence and thus less likely to unduly influence its decisions.
Principle 4: An AI IO should implement formal policies for handling earmarked voluntary contributions.
Earmarked voluntary contributions raise two additional concerns. First, earmarked funding may reduce an IO’s efficiency by increasing its administrative burdens. Employees must spend more time raising funds, maintaining donor relations, and reporting back to donors on the status of their donations. Second, earmarked funding may allow members to bypass formal governance mechanisms. For example, members will sometimes condition funds on them not being used to assist countries upon which the donor has imposed sanctions. An AI IO can mitigate these disadvantages through formal rules like the ones proposed by Kristina Daugirdas that govern the acceptance of voluntary contribution. She recommended (a) requiring projects to be funded by a minimum number of donors, (b) capping the percentage of a program’s budget that can come from any single donor, and (c) predefining the types of funding restrictions that are acceptable—a general restriction that donated funds be used only for vaccines might be acceptable, while a more specific restriction that such funds be used to purchase coronavirus vaccines manufactured in the donor’s country might not be.
Principle 5: An AI IO should defray the cost of administering its programs through self-funding activities.
IOs sometimes reduce the financial burden placed on members by raising money through their own activities. The ISO, for example, received nearly a third of its revenue in 2023 from royalties collected on the sale of standards by its members. Similarly, 20.9 percent of the ITU’s budget in 2024 came from cost-recovery activities like publication sales and radiocommunication filing fees. As an extraordinary, though not technically international, example, the U.S. Federal Reserve is entirely self-funded by interest collected on assets owned through its Open Market Operations. An AI IO should similarly supplement its budget through self-funding activities, though the exact form of these efforts will depend on the AI IO’s function. For example, an AI IO that enforces standards might charge for audits. The U.S. Chamber of Commerce estimates that AI audits can cost companies hundreds of thousands of dollars. Although this may sound expensive, publicly traded companies currently spend an average of $2.2 million on compliance with the Sarbanes-Oxley Act’s financial reporting requirements. For the 45 AI companies with the resources to build their own foundation models, several hundred thousand dollars will not be prohibitively costly. If member states passed laws requiring all leading AI companies to be audited by the AI IO, it could generate $10M (~45 companies x ~$200K) each year through fees.
***
This is only the beginning of a much-needed discussion of how to implement a new international AI organization. More research into the functions not currently being performed by existing public or private organizations is still needed. Entire articles could (and should) be written about how to fund an AI IO in each of the six categories discussed. For now, it’s clear that without a solid funding plan that can support its success, a new AI IO will merely fragment the international ecosystem and dilute funding for AI-related organizations. As policymakers scramble to regulate AI, the question of funding may determine whether this technology is shaped for the benefit of all, or for a wealthy few.