AI and Declassification: Will LLMs Bring More Transparency—or Less?
.png?sfvrsn=efa9577c_5)
Published by The Lawfare Institute
in Cooperation With
The U.S. government keeps too many secrets. Even the intelligence community thinks so. In remarks last year at the University of Texas, Director of National Intelligence Avril Haines observed that “classified information, whether properly or not, has increased significantly over the last 50 years.” Overclassification, she lamented, “undermines the basic trust that the public has in its government.”
Enter AI. Large language models (LLMs) can already “read” and interpret text with impressive fluency. Transparency experts hope that LLMs will help overtaxed officials review, redact, and declassify documents much faster than with human review alone. Pilot initiatives within the government have shown promising results.
But for advocates of greater transparency, LLMs carry underappreciated risks. U.S. adversaries like China and Russia have large language models too. And they are likely training LLMs to learn from U.S. disclosures.
It is thus far from certain that AI will be a net positive for transparency. Instead of solving overclassification, AI could force the intelligence community to declassify more cautiously. Indeed, our adversaries’ use of LLMs may require policymakers to rethink how the U.S. government declassifies information for public release.
Two caveats before we begin.
First: This piece describes (with concern) how adversaries might use AI to exploit our declassifications. It does so in the hope of spurring discussion about an issue that has not yet garnered public awareness. Out of an abundance of caution, I invited government officials to read this piece before publication.
Second: I have often argued for, and during my time in government worked to provide, greater official transparency about intelligence programs. This piece is not a call to curtail transparency in the interest of security. Rather, it aspires to sharpen our thinking about how best to reconcile transparency and security in an age when our adversaries use large language models to penetrate our secrets.
The Promise: AI as a Solution for Overclassification and the Digital “Tsunami”
Overclassification is not a new problem. In 1956, when the modern security-state was in its infancy, a Defense Department commission fretted that the system of secrecy was “ overloaded.” Four decades later, Sen. Daniel Patrick Moynihan lamented the incentive for workers in the intelligence bureaucracy to overclassify: “For the grunts,” he wrote, “the rule is stamp, stamp, stamp.”
Executive branch classification rules seek to counter that tendency by requiring that information be reviewed for declassification after 25 years. Meeting the 25-year target, however, depends on agencies’ having enough people to review material as it ripens for release. Often, they do not.
Digitization now threatens to swamp a system that is already maxed out. The Public Interest Declassification Board (PIDB), a federal advisory committee, has warned of a “tsunami of digital information” in the years ahead, as a glut of email and other digital artifacts created in the early 2000s edges toward the 25-year threshold.
The numbers are daunting: In a 2020 report, the board contrasted the 250 terabytes of digital information held in the Barack Obama Presidential Library with a mere 4 terabytes in the William J. Clinton Presidential Library. Unless our capacity to declassify information scales comparably, it will soon be overwhelmed.
Here, as in many other fields, AI has emerged as the great hope. At a 2023 conference co-hosted by my organization, the Strauss Center for International Security and Law, panelists, including PIDB members and AI experts, discussed whether AI could be “used like a spell check” to “assist with classification,” such as by highlighting potential redactions for human review. Since then, the PIDB has continued to investigate the possible benefits of AI to support human declassifiers. Congress endorsed that idea in the Sensible Classification Act of 2023, which required the executive branch to “research a technology-based solution utilizing machine learning and artificial intelligence to support efficient and effective systems for classification and declassification.”
Director of National Intelligence Haines has also made this a priority. In remarks last year at UT-Austin, she shared that the intelligence community is “experimenting with artificial intelligence and machine learning tools … to automatically identify records for declassification.”
Few expect AI to replace human declassifiers altogether. The decisions are too subtle and the stakes too high: Errors can endanger human sources or burn technical accesses.
Yet AI could make human reviewers much more productive. AI could tag potential classified material and propose redactions for a human reviewer to consider. It could also recommend portion markings and highlight material that it recognizes as having been previously declassified elsewhere.
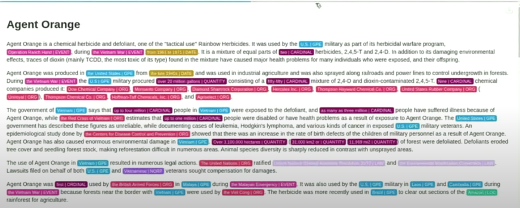
Depiction of AI tagging, presented by Dr. Michael Brundage (University of Maryland, Applied Research Laboratory for Intelligence and Security) and John D. Smith (Office of the Secretary of Defense) at the Public Interest Declassification Board’s June 2024 hearing.
Some agencies are already moving in this direction. The State Department, for example, has trained its own models “on past decisions by human reviewers.” It now uses those models to largely automate the process of reviewing cables for public release.
The results appear impressive. Using AI, the department reviewed its 1998 cables (which hit the 25-year threshold in 2023) in record time. State’s AI tool has now reviewed more than 450,000 cables in total.
The Problem: Adversaries Can Do It Too
There’s a problem, however: U.S. adversaries have large language models too. And the type of “thinking” that large language models do may be well suited to puzzling out secrets from the redacted documents that we release.
Guess the Missing Word
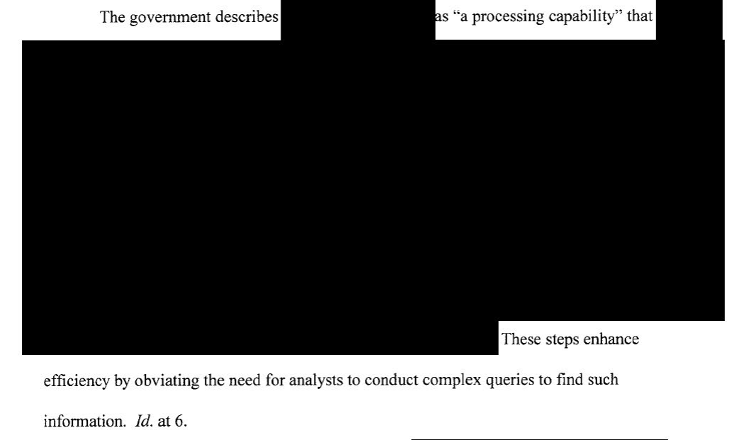
Screen capture from the Foreign Intelligence Surveillance Court’s April 2023 opinion approving the government’s annual “certifications” under Section 702 of the Foreign Intelligence Surveillance Act.
What lurks behind those black boxes? China, Russia, and other U.S. foes (perhaps even some allies) would love to know. But if they can’t know, in the platonic sense, the next best option is to infer with high confidence.
And that is what large language models do: infer. Computer scientist Stephen Wolfram explains that a large language model like GPT-4 is “always fundamentally trying to … produce a ‘reasonable continuation’ of whatever text it’s got so far.” At bottom, the model is guessing—or, to put it more charitably, inferring—the next block of text based on the context and on statistical relationships in the training data.
Essentially, it’s a game of “guess the missing word.”
Humans do the same thing, of course. An experienced analyst can hazard an educated guess about what a redaction masks. But LLMs and humans working together may be able to do it much better, for reasons of both skill and scale.
First, LLMs may be especially well suited to the task of puzzling out secrets from a large corpus of documents. Machine-learning algorithms work by identifying and then applying patterns in training data—including latent patterns that humans might miss, even with traditional statistical methods. During the training process, those patterns become embedded in the model as weights, which then influence the model’s output. If U.S. officials have unconscious, subtle tendencies in how they write about certain programs or how they redact classified descriptions of those programs, LLMs can be used to detect and exploit those tendencies.
Here, LLMs’ lack of common sense can be an asset. Unconstrained by personal experience or institutional culture, the models follow the data where it leads, even to counterintuitive results. Perhaps classified documents describing one technology tend to employ certain, seemingly innocuous adjectives, or to cite certain types of sources. LLMs could correctly attribute meaning to a small clue that humans would overlook.
Lacking common sense has downsides too, of course. “Hallucinations” remain an obstacle to adopting language models in many business contexts. But a human analyst supervising the LLM can always discard bad guesses. A few gems will make the exercise well worth the while.
The second advantage is scale. Uncloaking American redactions can be fruitful, but adversaries must weigh that against other tasks that can be assigned to their limited cadres of analysts familiar with the U.S. target. An LLM trained to puzzle over our declassified materials might resolve that resource dilemma. Teaming with AI could allow fewer analysts to squeeze more insight out of far more U.S. documents.
GPT for the GRU?
It sounds workable in theory. But how good would an adversary LLM’s guesses be in practice?
Unfortunately, they might be quite good. Our principal adversaries have access to plenty of U.S. intelligence documents, which they could use to fine-tune an open-source model or their own proprietary LLM. Their sources include:
- Snowden documents. Edward Snowden “stole 1.5 million sensitive documents,” which the Russian and Chinese governments may have taken during Snowden’s peregrinations from Hong Kong to Moscow. Other Snowden documents were posted online by journalists.
- Classified documents stolen by human spies or hackers working for adversary intelligence services.
- Authorized releases of materials declassified by the intelligence community.
- Unclassified information, including unclassified descriptions of classified programs and high-quality, open-source studies of U.S. intelligence capabilities.
- Adversaries’ internal assessments of U.S. intelligence and defense capabilities.
In short, there’s plenty to work with. A capable U.S. adversary with the necessary hardware could fine-tune an open-source model on a sizable corpus of documents related to U.S. security and defense capabilities. From there, the adversary could use the model in different ways to unlock insight in redacted or unclassified releases. As expert analysts read redacted documents, a co-pilot tool could prompt the analyst with the most probable options for what lurks behind redaction blocks, allowing the analyst to benefit from the LLM’s powers while layering his or her own common sense and experience on top. Or the model could be queried for insights across the full corpus of documents.
Perhaps most guesses would be wrong. But human analysts would vet the output and investigate promising suggestions further. Even a few nuggets of insight would justify the endeavor.
Declassification in an Era of Adversary LLMs
Our adversaries are not fools. They scour each intelligence leak or authorized release for new insight into U.S. government capabilities. We can reasonably assume that their AI efforts are already beyond the rudimentary steps described here. This has several implications for the future of declassification.
Redacting Original Documents: More Transparency, More Trust, More Risk
Certain aspects of how the U.S. government redacts documents today make U.S. adversaries’ task easier. Typically, the intelligence community begins with the original document, identifying redactions needed to make the text unclassified and then superimposing empty boxes over the redacted text.
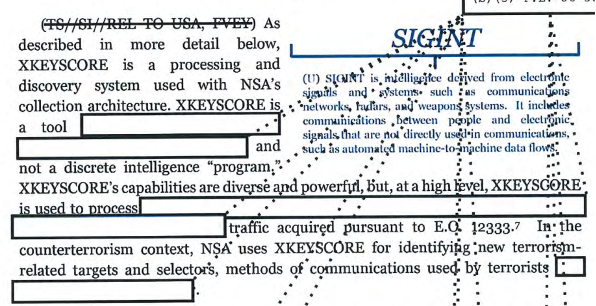
Screen capture from declassified Privacy and Civil Liberties Oversight Board report on NSA’s XKEYSCORE capability.
Showing the original document makes transparency more credible. The public can see what, and how much, remains concealed. The reader sees ground truth, even if it is partial.
The problem is that black-box redactions help U.S. adversaries, perhaps considerably. The redacted text is surrounded by semantic and substantive context. And the size of the box provides useful metadata: the length (even, potentially, the number of characters) of the text behind. The document thus provides both fodder to train the adversary’s LLM and rich context to help it make more accurate inferences.
Comparisons between redacted and unredacted versions could be especially fruitful for adversaries’ LLMs. This would be possible if a document were released first in redacted form and then later re-released with fewer or no redactions. Alternatively, an adversary could compare purloined classified documents with redacted versions released through authorized processes. In the words of one AI expert I consulted while researching this piece, the “ground truth” from such comparisons would be “gold” for hostile attempts to infer U.S. secrets using an LLM.
The speed with which models are improving creates yet another conundrum. Even if something seems safe to release today, there is no telling what an adversary may be able to tease out using next year’s AI capabilities. AI models are making “startlingly rapid” progress. As with quantum-proof encryption, the challenge is to ensure that today’s secrets will be safe from tomorrow’s technology.
What, If Anything, Can We Do About It?
In the U.S., trust is the bedrock on which all national security powers ultimately rest. Unfortunately, polls show that trust in intelligence agencies is declining overall and sharply splintering along partisan lines. This year’s rough ride for the government to obtain a mere two-year reauthorization of the Foreign Intelligence Surveillance Act’s Section 702 shows the political effects of lost trust. Since the Church Committee and Watergate, we have accepted some diminution in operational security to secure public trust and ensure democratic accountability. AI or not, that fundamental bargain cannot change now.
Therefore, a deeply misguided way to “solve” this problem would be to dramatically reduce the amount of transparency that we provide into U.S. intelligence programs. This would limit the amount of insight available to our adversaries, but at an intolerable cost to public trust.
Firm prescriptions will likely be embarrassed by how the technology develops. Still, there are actions we can take now to avoid a harsher reckoning later.
1. Begin Resetting Expectations
The first step is accepting that there is a problem. That means recognizing the possibility that existing declassification processes may have to be adjusted to protect secrets in an era of proliferated LLMs.
Surely the intelligence community is aware of this emerging risk to its secrets, but Congress and the public should also be brought up to speed. If existing declassification practices are to change, the government will bear the burden of explaining why and persuading skeptics. To start this process, the congressional intelligence committees could hold hearings on the risk that adversary LLMs pose to U.S. secrets and LLMs’ potential implications for our approach to declassification and transparency.
2. Research Defensive Technologies
Today’s declassification processes were built to shield redacted materials from human prying—not from LLMs. To declassify with confidence today, agencies will have to stress-test proposed releases against attempts by LLMs to infer redacted text or to intuit secrets across a corpus of releases. That means simulating what adversaries can do with this technology. And that depends, in turn, not merely on the power of their models but on what data about our programs they will use to fine-tune their models and supply them with context. Technical experts in the intelligence community should already be tackling this task.
3. Consider Changing How We Redact New Documents
Agencies could also make guessing harder by adjusting how they redact. Black boxes on original documents hide the redacted text itself but still provide an adversary with useful information about what is concealed. Most prosaically, the boxes indicate the length of the redaction (even, depending on the font, the exact character count).
A practice known as “square-bracket redaction” at least takes that clue away. Instead, the reader sees a bracketed phrase describing the nature of the redacted text at a high level of generality. A report by Canada’s National Security and Intelligence Committee of Parliamentarians (NSICOP) describes this technique:
Where information could not simply be removed without affecting the readability of the document, the Committee revised the document to summarize the information that was removed. Those sections are marked with three asterisks at the beginning and the end of the summary, and the summary is enclosed by square brackets[.]
Unlike black boxes, the length of the bracketed phrase does not betray the length of the original, classified description.
The NSICOP and another Canadian body, the National Security and Intelligence Review Agency (NSIRA), have pioneered the use of square-bracket redaction in their public reports. These examples drawn from NSIRA’s 2022 Annual Report illustrate the technique (“CSE” refers to the Communications Security Establishment, Canada’s counterpart to the U.S. National Security Agency):
8. NSIRA finds that CSE has not developed policies and procedures to govern its participation in [*specific activity*].
8. NSIRA finds that, in its [*a specific document*], CSE did not always provide clarity pertaining to foreign intelligence missions.
There is a catch, of course: Unlike traditional black-box redactions, the public does not see the “original” document. Rather, it sees a slightly different version, with the redacted sections removed altogether and the bracketed summaries inserted in their place. Square-bracket redaction also works only for new documents, not historical releases.
How square-bracket redactions are implemented would be key in preserving credibility and trust. For judicial opinions, independent oversight reports, and congressional reports, the text of the square-bracket summaries should be drafted by the entity that wrote the underlying product, not the agency conducting the classification review. The reviewing agency must, of course, ultimately verify that the replacement text is indeed unclassified. But having the court, agency, or congressional committee confirm that the replacement text faithfully reflects the underlying document will make square-bracket redactions more credible.
4. Release Much Less?
Other potential responses would more radically alter what and how we declassify. These measures would be fraught in an era of fragile public trust, even if they would marginally reduce the insight available to U.S. adversaries. Still, they are worth mentioning given uncertainty about how AI will evolve, and what countermeasures government agencies might seek to employ, in the years ahead.
One option is that the government could simply release less. The more relevant data our adversaries have, the more accurate their AI models will be. And not every intelligence-related release serves equally important public interests. Hypothetically, instead of presuming that everything should be released eventually, we could adopt the “sharp delineation” proposed by former Director of Central Intelligence William Colby: “Politically significant information needed for public decision-making” would be released, while “the technical detail not essential to such decisions” need not be.
The problem is deciding what goes in which category. The few in the know might be tempted to place edge cases in the “technical detail” category. It is thus hard to imagine this line holding for long.
Alternatively, agencies could release unclassified summaries of important documents, instead of redacted versions of the documents themselves. In criminal prosecutions, the Classified Information Procedures Act permits the government to provide a defendant with a summary of relevant classified information, instead of disclosing it, as long as the summary will “provide the defendant with substantially the same ability to make his defense.”
In theory, this would give the public the gist of a document while depriving the adversary of useful grist for its LLM training. In practice, however, mere summaries would inspire little trust, instead fueling public calls for the government to reveal more.
The Biden administration has said that it plans to release revisions to the executive orders governing classified information before the end of this year. It is unclear whether or how those revisions will address the potential threat from adversary LLMs.
***
AI gives us new power to find hidden signals. It unmasks patterns that would be missed by human senses or intuitions.
Of course, this was true of earlier security technologies too. Wiretaps, hidden microphones, satellites, and fingerprints all revealed secrets that were once obscured. But AI differs from these earlier technologies in two important ways.
First, unlike a satellite passing overhead, we don’t yet know how to hide our secrets from AI. A large language model’s outputs are inherently unpredictable and depend on many factors that may not be known to the defenders. At best, we can simulate the adversary’s attacks to ascertain whether our secrets are safe. That is hard to do and provides limited assurance at best.
Second, AI can potentially unlock secrets created in the past. That means that materials we create now must withstand models that may be much more capable very soon.
AI could well herald faster declassification, greater openness, and thus more public trust. But U.S. adversaries have their own plans. Time, and the models themselves, will tell.