Artificial Intelligence Research Needs Responsible Publication Norms
After nearly a year of suspense and controversy, any day now the team of artificial intelligence (AI) researchers at OpenAI will release the full and final version of GPT-2, a language model that can “generate coherent paragraphs and perform rudimentary reading comprehension, machine translation, question answering, and summarization—all without task-specific training.” When OpenAI first unveiled the program in February, it was capable of impressive feats: Given a two-sentence prompt about unicorns living in the Andes Mountains, for example, the program
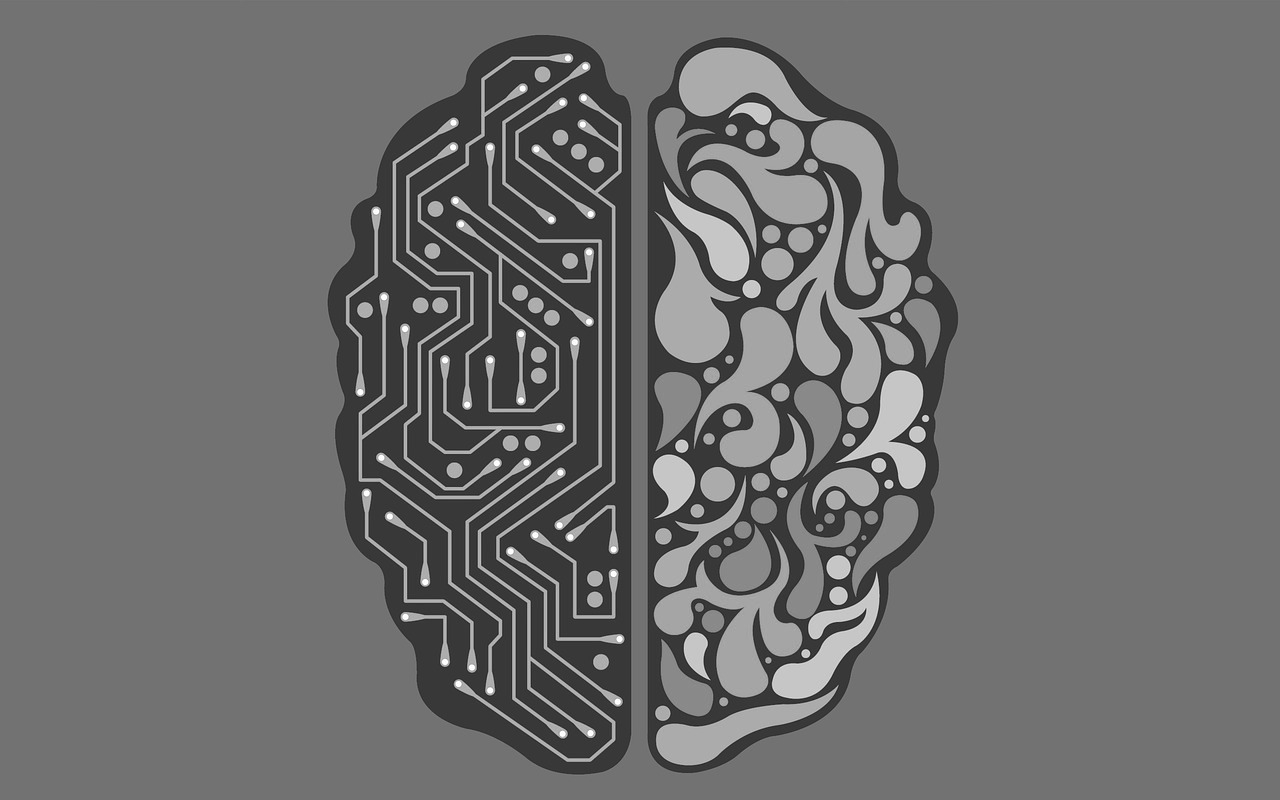
Published by The Lawfare Institute
in Cooperation With
After nearly a year of suspense and controversy, any day now the team of artificial intelligence (AI) researchers at OpenAI will release the full and final version of GPT-2, a language model that can “generate coherent paragraphs and perform rudimentary reading comprehension, machine translation, question answering, and summarization—all without task-specific training.” When OpenAI first unveiled the program in February, it was capable of impressive feats: Given a two-sentence prompt about unicorns living in the Andes Mountains, for example, the program produced a coherent nine-paragraph news article. At the time, the technical achievement was newsworthy—but it was how OpenAI chose to release the new technology that really caused a firestorm.
There is a prevailing norm of openness in the machine learning research community, consciously created by early giants in the field: Advances are expected to be shared, so that they can be evaluated and so that the entire field advances. However, in February, OpenAI opted for a more limited release due to concerns that the program could be used to generate misleading news articles; impersonate people online; or automate the production of abusive, fake or spam content. Accordingly, the company shared a small, 117M version along with sampling code but announced that it would not share key elements of the dataset, training code or model weights.
While some observers appreciated OpenAI’s caution, many were disappointed. One group of commentators accused the organization of fear-mongering and exaggerating the dangers of the technology to garner attention; others suggested that the company had betrayed its core mission and should rename itself “ClosedAI.” In May, OpenAI released a larger, 345M version of the model and announced that it would share 762M and 1.5B versions with limited partners who were also working on developing countermeasures to malicious uses. Again, some applauded. Others remained unimpressed.
Regardless of whether GPT-2 was dangerous enough to withhold, OpenAI’s publication strategy spurred a much-needed interdisciplinary conversation about principles and strategies for determining when it is appropriate to restrict access to AI research. In the past eight months, OpenAI’s efforts as a norm entrepreneur have already influenced others: The Partnership on AI hosted an event to discuss the tension between open research and precautionary concerns. The company Hugging Face limited its release of a social chatbot, described how the system could be misused, and suggested means of identifying others’ misleading chatbots. When researchers with the University of Washington and the Allen Institute for AI Research revealed GROVER, a GPT-2-style language model, they decided against releasing a large version of the model—a decision based in part on OpenAI’s argument. Similarly, AI startup AI21 Labs released a 345M version of their neural text generator, on the grounds that it was “equivalent in size to the publicly released versions of Grover and GPT-2.” “Curious hacker” Connor Leahy independently replicated OpenAI’s unreleased 1.5B GPT-2 and planned to publicly release it—but then decided against doing so precisely to help forge responsible release norms.
The GPT-2 case sparked a spirited debate within the AI community on whether OpenAI made the right call. Now, the task is to extrapolate out from the GPT-2 case study and develop consensus around responsible AI publication norms.
A Growing Risk
Like all technological advances, AI has benefits and drawbacks. Image analysis can speed up medical diagnoses, but it can also misdiagnose individuals belonging to populations less well-represented in the dataset. Deep fakes—computer-generated realistic video or audio—allow for new kinds of artistic expression, but they also can be used maliciously to create blackmail material, sway elections or falsely dispel concerns about a leader’s health (or the “well-being” of disappeared individuals). Algorithms can assist with financial trading or navigation, but unanticipated errors can cause economic havoc and airplane crashes.
It is heartening that AI researchers are working to better understand the range of harms. Some researchers are delineating different kinds of potential accidents and their associated risks. Some are identifying risks from malicious actors, ranging from individuals engaging in criminal activity and harassment, to industry exploiting users, to states and others engaged in social and political disruption. Still others are focused on how AI may create long-term, less obvious “structural risks”—shifts to social, political and economic structures that have negative effects—such as destabilizing the nuclear deterrence regime. Many AI governance principles suggest that researchers attempt to minimize and mitigate these risks throughout the AI lifecycle. Meanwhile, in the wake of AI advances, multistate and supranational bodies, individual states, industry actors, professional organizations and civil society groups have been churning out ethical principles for AI governance.
Still, there is no agreement about AI researchers’ publication obligations. Of all the new ethical guidelines, only a few entities explicitly acknowledge that there may be times when limited release is appropriate. The Malicious Use of AI Report, OpenAI’s Charter and the EU High Level Expert Group on Artificial Intelligence’s “Trustworthy AI Assessment List” all discuss situations where limited publishing is preferable. Meanwhile, individual researchers have also advocated for calculating DREAD scores—which weigh the potential damage, attack reliability, ease of exploit, scope of affected users and ease of discovery—when designing machine learning systems and outlined questions to consider before publishing.
At the time, Stephen Merity, a machine learning researcher, commented on the OpenAI controversy: “None of us have any consensus on what we’re doing when it comes to responsible disclosure.... This should be concerning for us all, in and out of the field.”
What Factors Should Be Considered?
OpenAI modeled one approach to implementing a responsible publication strategy. The company fostered a public debate by discussing and providing evidence of their program’s capabilities, describing what they were and were not releasing, and listing their concerns about potential misuses. By participating in a limited information-sharing regime with trusted participants, OpenAI minimized one problem of closed publication—that it systematically advantages larger research institutions at the expense of smaller ones, risking consolidated control of technological advances.
However, OpenAI was concerned primarily with risks from intentional misuses and implicit bias (such as GPT-2’s tendency to associate males and he/him pronouns with the term “criminal”). In the future, other AI researchers should consider a wider range of factors in weighing obligations for responsible publication. More complete publication norms for AI would weigh and balance a broader range of factors, possibly including the following:
- Source of Harm. What are the potential accidents? Intentional misuses? Structural risks and other indirect sources of harm? Other negative externalities?
- Harmed Subject. Who might be harmed? Individuals, groups or societies? Members of vulnerable or disadvantaged populations? Other living entities? The environment? Social, economic or political structures?
- Type. What form might the harm take? Would it be to physical or mental health? To human rights? To economic stability? To the environment? Will the harm be particularized or diffuse?
- Magnitude. What might be the damage potential? How many entities are likely to be harmed? Can the kind of harm be measured quantitatively? If not, how to avoid underestimating it?
- Likelihood. What is the likelihood of this harm occurring? For would-be malicious actors, what resources are required?
- Time Frame. When might this harm be likely to occur? Will there be time to raise awareness, develop responses or otherwise ward off the worst effects?
- Permanence. Might the harm be fleeting or long lasting?
- Response. Is there a means of limiting discovered harm? Can the kind of harm be reversed or corrected? Are there means of insuring against the potential damage?
- Information and Power Asymmetries. Are harmed entities likely to learn of the injury? Be able to trace it to the source? Report it? Have an effective route of redress? Be well-represented? Be compensated or otherwise have the harm remedied?
- Opportunity Costs. Given that not sharing research may create harms, what benefits or opportunities might be foregone by not publishing?
One critical structural question is which entity should be weighing the potential risks of a technology against its potential benefits. The team of researchers? The research institution? An independent board, inside or outside of the institution? A private or government entity? A domestic or international organization? As different institutions will have different strengths and weaknesses, there may be a need for multiple structures to evaluate different kinds of harms.
While there will always be sector-specific concerns, there is no need to reinvent the wheel. Nuclear, life sciences, cryptography and other researchers working on potentially dangerous technologies have long been discussing and developing responsible release norms that AI researchers can consider in crafting their own.
The Pretext Problem
Granted, responsible publication norms may be used to support pretextual claims: An entity might overstate security concerns or other risks to justify self-interested nondisclosure. But open research can take many forms—including, as Nick Bostrom observed, “openness about science, source code, data, safety techniques, or about the capabilities, expectations, goals, plans, and governance structure of an AI project.” Without sharing everything, researchers can be transparent about what a system can do and their reasons for nondisclosure, so that others can weigh the commercial and security benefits of the technology against the credibility of the concerns. When proffered reasons don’t pass the laugh test, the researchers can be publicly critiqued—and, in the process, the community publication norms will be further defined, refined and internalized.
Ultimately, all norms enjoy only limited compliance. There will always be researchers who do not engage in good-faith assessments, just as there are now researchers who do not openly share their work. But encouraging the entire AI research community to consider the risks of their research—to regularly engage in “Black Mirror” scenario-building exercises to the point that the process becomes second nature—would itself be a valuable advance.
Pandora’s Box Paralysis
Additionally, there is the Pandora’s Box problem: Research can always be released at a later date—but, once released, it cannot be reined in. Meanwhile, it is impossible to accurately predict how AI might adaptively evolve or be misused. The irreversibility of disclosure and the unknowability of potential harms suggest favoring nondisclosure, but adherence to a strong version of the precautionary principle may transform it into a paralyzing principle, chilling the development and spread of socially beneficial technologies.
Weighing the benefits of openness against responsible disclosure is no easy task. As is often the case when balancing competing social goals, there will rarely be a clear-cut answer. Precisely because political and market incentives may place undue weight on the scale in favor of immediate, concrete or concentrated benefits over long-term, abstract or diffuse risks, we need to create shared ex ante principles—and, eventually, institutional structures to implement and further develop them. While it will be impossible to fully predict the future, researchers can at least increase the likelihood that these evaluations will be based on considered reasoning rather than (possibly unconscious) self-interested intuitions.
Incentivizing Adoption
Responsible publication norms could be integrated into the AI research process in various ways, ranging from voluntary implementation to more formal requirements. The EU Expert Group is currently testing a “Trustworthy AI Assessment List,” which requires researchers to evaluate various kinds of risks posed by their system. Alternatively, researchers might engage in “algorithmic impact assessments” to consider a broader range of potential harms, as Andrew Selbst has proposed for predictive policing companies and AINow has discussed in its policy framework. Some researchers are proposing leveraging the peer review process to encourage such considerations, which will help promote norm internalization. And, of course, law can be used indirectly to incentivize safety by creating liability for tech-enabled harms for states, industry and individuals.
Robert Heinlein has observed, “The answer to any question starting, ‘Why don’t they—’ is almost always, ‘Money.’” In thinking about how best to incentivize norm adoption, it is important to recall that regulations can shape technological development by creating carrots as well as sticks. For example, tax incentives can foster research in particular areas, and government contracts and philanthropic research grants could condition awards on thorough risk assessments.
Even though OpenAI will soon be releasing the full model of GPT-2, it is critical that the conversation around thoughtful release continues, informally and formally, until the AI research community develops shared responsible publication norms.
Thanks to Hannah Bloch-Wehba, Miles Brundage, Jack Clark, Evan Selinger and Ram Shankar for conversations that informed this piece. This publication was made possible (in part) by a grant to the Center for a New American Security from Carnegie Corporation of New York. The statements made and views expressed are solely the responsibility of the author.