Beyond Big Tech: The Revolutionary Potential of State Data Commons
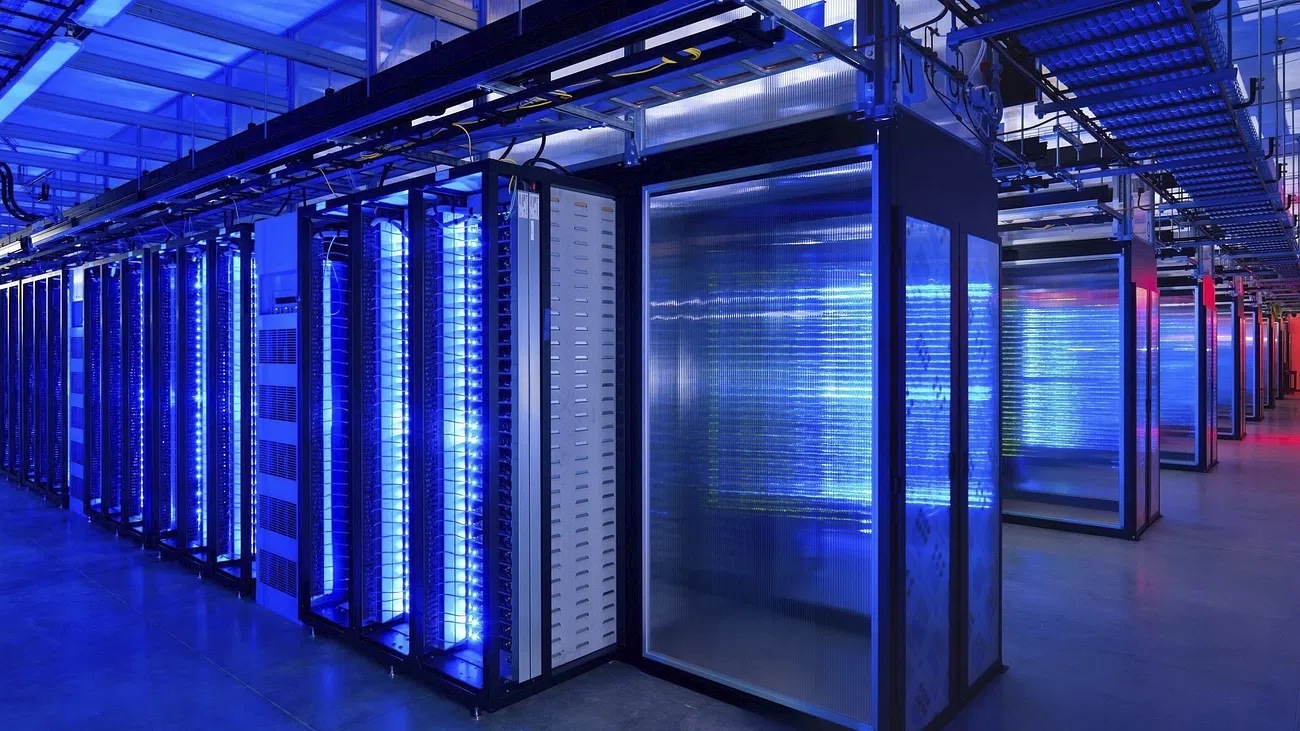
Published by The Lawfare Institute
in Cooperation With
The Department of Government Efficiency’s (DOGE’s) use of artificial intelligence (AI) has sparked widespread concerns about further integrating new tools into essential government services. Likewise, the State Department’s plans to use AI to revoke visas from foreign nationals deemed “pro-Hamas” has raised public concerns. This general rush to apply an AI hammer to anything that looks remotely like a nail risks pounding a message into the minds of many Americans: AI is bad.
If that message becomes too entrenched, Americans may uniformly disapprove of even responsible uses of AI to improve government services. That may set up an unfortunate paradigm: a private sector that continues to develop AI at a breakneck pace and a public sector unable to leverage these powerful tools to enhance education, health care, and other vital services. This technological gap threatens not just America’s competitive edge against strategic rivals but, more fundamentally, the capacity of the federal government and state governments to improve the lives of Americans across the country.
The primary challenge to realizing AI’s potential to improve governance and the delivery of public goods is not technical—AI capabilities appear to advance daily—but rather institutional and social. Success in deploying AI for public benefit hinges on three critical factors: public acceptance of AI in sensitive domains, such as public health emergencies; regulatory permission for such deployments; and, perhaps most crucially, access to the high-quality data needed to develop these tools. This last factor, while less discussed in public discourse, may prove the most decisive in determining whether AI serves all Americans rather than only a privileged few.
Consider the current landscape of AI development. Major tech companies and well-funded labs can draw upon vast proprietary datasets to train their models. But what about the startup developing AI tools to identify early warning signs of student struggle in rural school districts? Or the nonprofit seeking to create language models that understand regional health care dialects and customs? These smaller, mission-driven organizations often lack access to the rich, contextual data needed to create tools truly responsive to local needs and circumstances.
Recent controversies over web scraping have only exacerbated this data drought. A 2024 report by MIT Technology Review and data provider Snowflake found that 78 percent of businesses surveyed lacked the data foundations needed to take advantage of AI deployments, a finding that has been confirmed repeatedly by other research. Data that was available to OpenAI during its initial training runs is no longer available to aspiring competitors as website owners seek to prevent their content from being used for AI training. Although some AI developers have turned to synthetic data—which is artificially generated rather than collected from real-world sources—as an alternative, questions remain about whether artificial data can capture the nuanced patterns of human behavior and needs that are required for public-service AI. The result is a growing gap between AI’s theoretical potential to serve the public good and the practical ability of startups, small business, and public institutions to realize that potential.
The Promise of State-Led Data Commons
A solution may lie in an old idea adapted for the AI age: the commons. Specifically, state-managed data commons that invite individuals to voluntarily share information for the explicit purpose of improving public services through AI development. A number of data commons are already in operation for research purposes, and this approach offers several distinct advantages over current data collection and sharing practices.
First, states already serve as custodians of vast amounts of sensitive information in education, health care, and other domains ripe for AI innovation. They have existing relationships with residents and established (if imperfect) protocols for data security and privacy protection. More importantly, states possess something that private companies often lack: a clear public-service mandate and direct accountability to their community.
Consider Utah as a potential pioneer of this approach. The state combines several advantageous factors: high levels of social trust, a thriving health care technology sector, and a tradition of innovative public-private partnerships. A Utah Data Commons could invite residents to share health information beyond what’s already in state databases, with the explicit understanding that this data would be used to develop AI tools for improving health care delivery in the state.
Local companies could access this data under strict conditions, including robust security requirements and commitments to develop tools specifically for Utah’s communities. The resulting innovations might include AI systems that predict health complications in underserved rural areas or tools that help coordinate care across the state’s unique geographic challenges.
The Data Commons should also adhere to data protection best practices including restrictions on data access, use, and retention. Implementation of robust cybersecurity measures by the Commons will also be essential to both earning and maintaining residents’ trust.
Technical and Security Considerations
The path to establishing effective state data commons is fraught with technical challenges. Recent years have shown that state governments, despite their experience handling sensitive data, are not immune to security breaches. The 2019 ransomware attacks on multiple state and local governments serve as a sobering reminder of the risks involved in centralizing valuable data.
To address these concerns, any state data commons initiative must incorporate multiple layers of protection. Participation should be strictly voluntary, with individuals maintaining granular control over what data they share and for what purposes. Access to the commons must be contingent on meeting rigorous security standards. Organizations seeking to use the data should be required to carry data breach insurance, submit to regular third-party security audits, and implement zero-trust architecture principles. The technical infrastructure must enforce strict data minimization and purpose-limitation requirements.
These technical safeguards must be complemented by legal frameworks that establish clear liability for breaches and misuse. The commons should operate under a model of progressive security requirements—the more sensitive the data accessed, the more stringent the security standards that must be met. At the same time, states could maintain oversight over commercial users to ensure that their practices meet standards for data security and data privacy.
Economic Sustainability and Incentive Structures
Creating and maintaining secure data commons requires substantial investment. While federal grants can provide initial funding, long-term sustainability demands a more complex economic model. One promising approach involves negotiating value-sharing agreements with organizations that benefit from the commons.
Companies developing AI products using commons data might commit to sharing a percentage of future revenues with the state. This could take the form of direct profit-sharing or commitments to provide services to state institutions at reduced rates. Such arrangements would create a virtuous cycle, where successful AI innovations generate resources to maintain and expand the commons.
States could implement tiered-access models, where basic research access comes at minimal cost while commercial applications require more substantial contributions. This approach would balance the need to support public-interest research with the reality of maintaining expensive infrastructure.
The Trust Deficit Challenge
Perhaps the most formidable obstacle to establishing state data commons is the current crisis of trust in government institutions. Recent surveys indicate that public trust in state governments, while generally higher than federal trust levels, varies significantly by region and has declined overall in the past decade.
This trust deficit creates a chicken-and-egg problem: Effective AI tools for public services require broad participation in data commons, but such participation requires trust in government data management. Breaking this cycle requires a new approach to government-individual relationships in the digital age.
States might consider establishing independent oversight boards for their data commons, building mandates for transparency, accountability, and independence from partisan interventions. These boards would include privacy advocates and civil rights organizations, technical experts from academia and industry, community representatives, and public health and education professionals. Through regular public reporting on commons impacts—specifically highlighting how shared data leads to improved services—these oversight bodies could help build and maintain public trust while preventing administrative misuse of sensitive data.
The Federal Role
While states should lead in establishing data commons, the federal government can play a crucial catalytic role. Beyond providing initial funding through conditional grants, federal agencies could establish minimum security and privacy standards for state data commons, facilitate cross-state data sharing where appropriate, provide technical assistance and best practices, and create incentives for AI developers to work with state commons.
Federal support should respect state autonomy while encouraging innovation. States that choose not to participate in data commons initiatives may find themselves at a growing disadvantage as AI tools become more central to effective public-service delivery. For the states that initially opt out, it seems likely that constituents will demand the creation of a commons after seeing the positive use cases in other states. The alternative—allowing local businesses to stagnate and seeing startups flock to other jurisdictions—seems politically untenable over the long run given each state’s interest in the economic dynamism made possible by a vibrant AI ecosystem.
Looking Forward
The success of state-led data commons could reshape the landscape of AI development in America. Rather than relying solely on large tech companies or federal initiatives, this approach would create a network of local innovation ecosystems, each responsive to its community’s needs and preferences.
Returning to the Utah example, imagine a future where the state’s data commons has enabled the development of AI tools that provide early intervention in mental health crises, optimize emergency response in remote areas, support personalized learning in K-12 education, and coordinate care for chronic conditions across providers. Such successes would provide a model for other states and demonstrate how thoughtful data sharing can drive meaningful improvements in public services.
The path to establishing effective state data commons will not be straight or simple. It requires careful balancing of competing interests, robust technical infrastructure, and new models of public-private collaboration. Yet the potential rewards—AI tools that truly serve all Americans, not just those in tech hubs or wealthy areas—make this challenge worth pursuing.
The alternative—allowing AI development for public services to be driven solely by commercial interests and available data—risks creating new forms of digital inequality. By establishing state data commons, policymakers can help ensure that AI’s benefits extend to all communities, advancing the promise of technology while preserving democratic values and local autonomy.