Congress Should Preempt State AI Safety Legislation
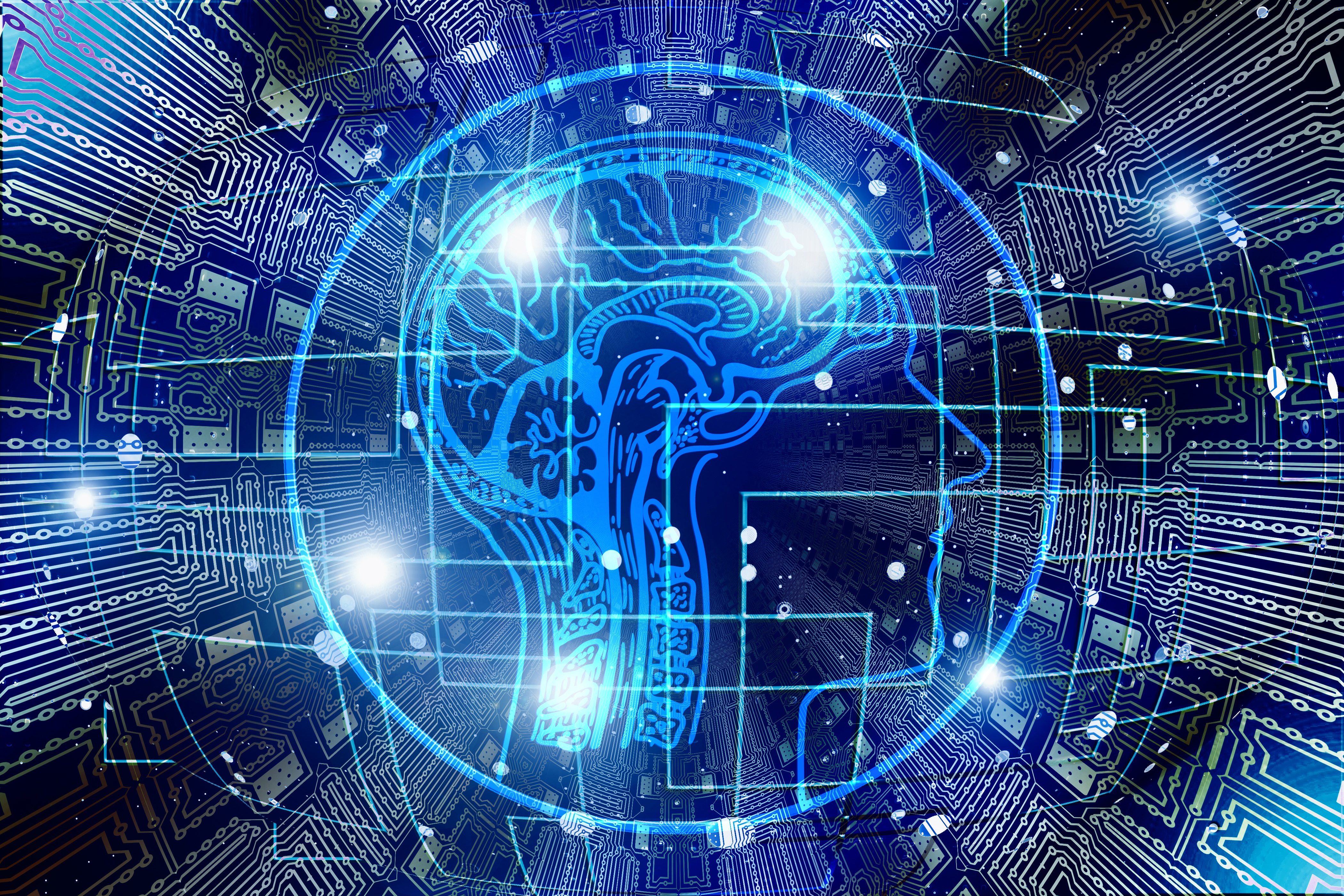
Published by The Lawfare Institute
in Cooperation With
As Congress debates how to regulate artificial intelligence (AI)—or whether to regulate it at all—state legislatures are pushing their own proposals. In the 2024 legislative season, states have considered hundreds of potential bills related to AI. Many of these are minor: creating statewide committees to oversee AI implementation within state agencies, for example, or clarifying that existing statutes apply to AI.
Some are farther-reaching and directly regulate the use of AI. Colorado, for example, passed a law that will require many businesses (including non-AI companies) to conduct “algorithmic impact assessments” for racial, gender, political, and other bias if they want to use AI for commerce in the state. A similar bill came close to passing in the Connecticut legislature but failed amid tech industry opposition.
Some of the proposed state bills are broader still in that they regulate the development of AI models, rather than just their deployment. Because regulation of model development in any one state is likely to have implications for AI development nationwide, Congress should take the lead by preempting legislation of this kind.
A good example of model-based regulation at the state level is California State Sen. Scott Wiener’s SB 1047, the Safe and Secure Frontier Artificial Intelligence Act. The bill raises many questions, but perhaps the most fundamental one is whether legislation such as SB 1047 is the legitimate purview of state governments in the first place.
The bill would create the Frontier Model Division, a new regulator within California’s Department of Technology. Developers of models trained with more than a certain (large) amount of computing power and that cost more than $100 million to train would be required to “provide reasonable assurance,” under oath, that their models are unable to cause $500 million in damage to critical infrastructure within the state or lead to a mass-casualty event involving chemical, biological, radiological, or nuclear weapons. Wiener introduced the bill in February, and it passed the state Senate last month; it is currently under discussion in the California Assembly.
SB 1047 raises many questions and has drawn numerous critics, some of whom think it is too weak, but most of whom worry that it goes too far, ranging from the largest AI labs (OpenAI, Anthropic, and DeepMind), AI startups, venture capitalists, academic researchers, and other tech-adjacent civil society groups. How, many wonder, will developers of frontier AI models be comfortable making the promise SB 1047 forces them to make?
For example, what does it mean for an AI model to “cause” $500 million in damage—if a Russian speaker uses ChatGPT to write a phishing email against an American power plant, did OpenAI “cause” that damage? The bill only specifies that a given hazardous capability must be “significantly more difficult to cause” without access to the AI model in question, but this creates further uncertainty. It is not a given that an attack of the kind envisioned by SB 1047 would leave behind detailed logs of what the attacker prompted the AI model to do. This could create a murky legal situation: If it is known—or suspected—that an AI model was used in an attack, but unclear precisely how, would a jury or judge conclude that it is likely that the model enabled capabilities that would have been “significantly more difficult” to obtain without it? And why single out AI—should Apple have to certify that every MacBook it sells will be impossible to use for a similarly dangerous capability? These are just some of the many uncertainties and ambiguities critics have identified in SB 1047.
One concern is institutional capacity: Can the California government—facing a $60 billion budget deficit and state-government pay scales—recruit the personnel it would need to staff an agency that would oversee some of the most complex technology ever developed by humankind? How can state legislators like Wiener, lacking access to classified intelligence and other national security information, weigh the difficult trade-offs between safety regulation and the hobbling of a geopolitically crucial industry?
A second risk of state-based AI safety regulation is the patchwork it could create. Frontier generative AI models are distributed on the internet and intended to serve as many customers as possible—that is the only way to justify the immense capital investment in computing infrastructure required to make them. To develop AI to its fullest, America needs a coherent, unified national strategy. We will need to answer basic questions such as whether we should regulate AI at the model layer (which is what SB 1047 aims to do), or instead (or in addition) police illicit applications or conduct enabled by AI (which is the direction the U.S. Senate’s AI roadmap suggested). Because these and many other questions involve the entire nation, they should be answered through discussion and debate at the national level. Premature regulation does not just risk stifling innovation and adoption of AI throughout the economy; it also risks permanently placing the U.S. on the wrong path, structurally weakening our capacity in a field of technology that virtually all observers agree could define the coming decades.
The third—and we think most fundamental—problem with state-based regulation is that a particular state’s views on the appropriate risk trade-off for AI may not be representative of the country as a whole. This is especially problematic in the case of California, where most of the AI labs are located and which thus could unilaterally make decisions about this technology on behalf of all Americans, the majority of whom don’t have a say in California’s legislative process.
It’s true that, if one is convinced that there is a substantial risk of near-term catastrophic risk from AI systems, and that the benefits of preventing that risk outweigh the costs of delaying the development of welfare-improving AI systems, then SB 1047 and laws like it are a good bet. But the problem is that this begs the question of what the actual risk is and what the appropriate risk-reward calculation should be. Our point is not to argue that the “doomers” are necessarily wrong (though we do take a more optimistic view) but, rather, that states are the wrong level to make these decisions.
Of course, since the launch of ChatGPT in November 2022, this exact argument about existential risk has played out in the media and the halls of Congress. Majority Leader Chuck Schumer notably asked participants in the Senate’s 2023 AI Insight Fora for their “p(doom),” a term used within the AI existential risk community to refer to one’s probability that AI causes human extinction or other catastrophic consequences.
Yet just a year later, the Senate’s bipartisan working group on AI—chaired by Schumer—released a roadmap advocating that the federal government spend $32 billion on AI-related research and prioritizing the “enforcement of existing laws” with respect to AI. The roadmap comparatively deprioritizes a model-based regulatory regime to combat existential and catastrophic risks, merely directing Senate committees to “develop an analytical framework that specifies what circumstances would warrant a requirement of pre-deployment evaluation of AI models.” This, coupled with both major-party presidential candidates choosing not to advocate for model-based safety regulations, suggests that congressional action on this issue may be a deliberate choice rather than the result of partisan gridlock or incompetence.
Thus, we believe that Congress should consider preempting state regulation of the development of AI models. Preemption of a general-purpose technology such as AI is challenging; therefore, a targeted approach is required to do it properly. There are many AI-related policy areas where states can legitimately enforce their own policy preferences as to the use of AI. For example, states could reasonably differ on whether they want AI systems being used in job searches or customer services or what liability regimes should apply to AI end users. In such areas the U.S. can leverage its “laboratories of democracy” to experiment with different approaches to unsolved regulatory problems in parallel—a luxury many countries do not have.
But like many general-purpose technologies, AI models themselves are better regulated at the federal level. We do not have different electrical standards—different kinds of electrical outlets, for example—in different states. Smartphone makers do not need to meet different technical standards for cellular connectivity in different states. Instead, these standards are codified at the national level.
Regulating technologies at the state level could create barriers to interstate commerce. And even if one state’s standards ended up as the de facto national standard (as occurred with California’s emissions regulations for cars), there’s no guarantee that those standards would be optimal in terms of the U.S.’s overall national competitiveness in AI. Nor do we think it is sufficient for Congress to keep the possibility of federal preemption in reserve, in case state safety regulations on model development become too onerous. Path dependency is a real thing in the development of technology, especially in capital-intensive fields like AI, where investment decisions are made years in advance. Given AI’s exponential trajectory, even a few-years delay could have massive consequences on the U.S.’s AI competitiveness.
Achieving a similar outcome for AI policy will require a careful balance, reserving certain avenues for AI regulation to the federal government. Broadly speaking, there are three approaches one can take to AI policy:
- Model-level regulation: Formal oversight, mandatory standards or safety procedures, and/or regulatory preapproval for frontier AI models, akin to SB 1047 and several federal proposals. In practice, many proposed liability regimes also fall into this category.
- Use-level regulation: Regulations for each anticipated downstream use of AI—in classrooms, in police departments, in insurance companies, in pharmaceutical labs, in household appliances, etc. This is the direction the European Union has chosen.
- Conduct-level regulation: A broadly technology-neutral approach, relying on existing laws to establish the conduct and standards we wish to see in the world, including in the use of AI. Laws can be updated to the extent that existing law is overly burdensome or does not anticipate certain new crimes enabled by AI.
Federal preemption of the kind we propose should focus, to the greatest extent possible, on preempting state laws in the first category. No one of the regulatory approaches is, on its own, likely to be sufficient. Nor can clear lines always be drawn between each. This means that any preemption law—even a targeted one—is likely to restrict some use- or conduct-level regulation when those approaches bleed into model-level regulation. It is hard to avoid this trade-off altogether, but policymakers should be aware of it nonetheless.
Preempting state-based AI model regulation would mean preventing states from passing laws such as licensing regimes for models or model developers, mandatory technical or safety standards for AI, or, in most cases, imposing liability on AI companies for user misconduct. To be clear, we do not necessarily oppose laws of this kind at the federal level—we are merely suggesting that such laws would excessively hinder AI development.
How might preemption of this kind be achieved? We suggest three distinct strategies.
Preempting Laws on Model Distribution
Many of the potential model-level laws described above would impose a particularly harsh burden on open-weight AI models. These are AI models whose weights—the set of billions or even trillions of numbers that define the model’s capabilities and behavior—are available for free on the internet. Open-weight models are the norm in the academic community and, until recently, within the top AI labs as well. Today, only Meta among the largest AI players makes its frontier models open-weight. Many other companies, however, make smaller open-weight models available, including Google, Microsoft, Apple, and French startup Mistral.
These models carry many present-day benefits. Researchers are able to probe into the internals of the models in a way that is impossible with closed models such as OpenAI’s ChatGPT. Businesses are able to adopt AI for their needs with more flexibility but without the risk of handing their sensitive data over to a closed model provider. Open-weight AI models are a version of open-source software—applications, utilities, and other software tools whose source code is made available to the public. Open-source software is at the heart of almost every website and smartphone sold today.
Some observers believe the same benefits of open-source software will apply to open-weight AI. Others believe that open-weight AI models constitute a potential catastrophic risk: The ability for anyone to edit open-weight models means that anyone can, in principle, remove their built-in safety features. For this reason, most forms of centralized model regulation are difficult to reconcile with open-weight AI. This is because of the permissionless nature of the open-source software tradition. Once a model is released as open-weight, anyone can copy and modify it for their own purposes. Few developers would do this if they could later be held liable for what someone else—completely unknown to them—does with their software. It is impossible to enforce mandatory technical standards on models that can be distributed for free on the internet. A licensing regime for open-weight models, while not strictly speaking infeasible, makes little sense if anyone can modify the models once they are released.
A preemption law could be used to prevent states from passing laws that could hinder the distribution of open-weight AI models. The above examples—licensing regimes, mandatory standards, and liability—could be explicitly stated, but the law should apply more broadly to prevent gray areas. The law could further be extended to closed-weight models by stating that if a proposed state law’s provisions would infringe on the distribution of open models, it cannot apply to closed models either.
Reserving Technical Standard-Setting to the Federal Government
Another approach policymakers could consider would be to reserve technical standard-setting for AI models to federal agencies like the National Institute for Standards and Technology (NIST), which has the most technical AI expertise of any government agency, state or federal. Importantly, this would include not only the ability to set technical standards but also the ability to mandate them for particular products unless and until the federal government has done so.
An exception could be made for the area in which state sovereign interests are especially high: state procurement for its own purposes. For example, if a state legislature sought to mandate that state or local government agencies could only purchase software that complied with NIST’s AI Risk Management Framework, they would be able to do so without violating the preemption law. The restriction would instead apply to the state’s ability to mandate such standards for commercial or personal uses of AI by businesses or individuals within its jurisdiction.
Restricting Liability Regimes
A third option would be to restrict state governments’ ability to create liability regimes, beyond a rebuttable presumption that users are responsible for their use of an AI model. This is in contrast to SB 1047, which seeks to impose liability for end-user misconduct on developers. Proponents of such measures argue that they are necessary to incentivize frontier AI developers to institute reasonable safety standards.
The trouble is that we do not currently know what reasonable safety standards for general-purpose AI models are, nor do we fully understand how to implement them at a technical level. Liability in other product areas with potential danger, such as automobiles, works because we have a robust technical sense of when a car has failed versus when a driver has failed. If a driver hits a pedestrian while scrolling their phone, everyone intuitively understands that the driver is responsible. If a well-maintained car’s brakes fail, causing a pedestrian to be struck, we similarly understand that the driver is not usually at fault. But had the first car manufacturers been required to implement reasonable safety standards without any guidance as to what those standards entailed, the result may well not have been safer cars but rather no cars at all.
To be sure, our intuition for how liability should apply to AI models is somewhat hazy, and we could easily expect it to change as AI models become more capable over time. We’re certainly not arguing for a Section 230-like liability immunity for model developers, just as no one would support a generalized liability waiver for automobile makers. Rather, in this moment, when the industry is still nascent, a rebuttable presumption of user responsibility likely strikes the right balance between innovation and accountability.
Over time this may very well change. Strong, detailed technical standards will help policymakers, companies, and courts alike navigate this complex and emerging area of liability law. In the meantime, however, preempting states from creating their own liability regimes—which could severely restrict the development, distribution, and adoption of AI models—could help America maintain its innovation ecosystem.
* * *
There are many unanswered questions about AI. Policymakers at all levels of American government are right to want to answer them. Yet we should not forget that uncertainty is the norm, not the exception, at the frontier of technology. Humans invented the steam engine a century before they discovered the science of thermodynamics that makes steam a viable source of power. We discovered electricity before we discovered the electron. We should not shy away from uncertainty, nor should we rush to resolve it with the first answer that comes to mind.
Ensuring that we do not rush is perhaps the best thing the federal government can do today to protect American innovation and global competitiveness in AI. Indeed, finding answers to the questions posed by AI should be hard, and more often than not, hard things take time to achieve. Preemption of state AI model regulation will give all of us the time we need to debate and resolve these challenging topics.