The EU’s AI Act Is Barreling Toward AI Standards That Do Not Exist
The EU needs the technical standards supporting its AI Act to be restrictive enough to protect consumers, but flexible enough to enable innovation. Given society’s current understanding of AI, there are serious doubts as to whether such standards are technically feasible.
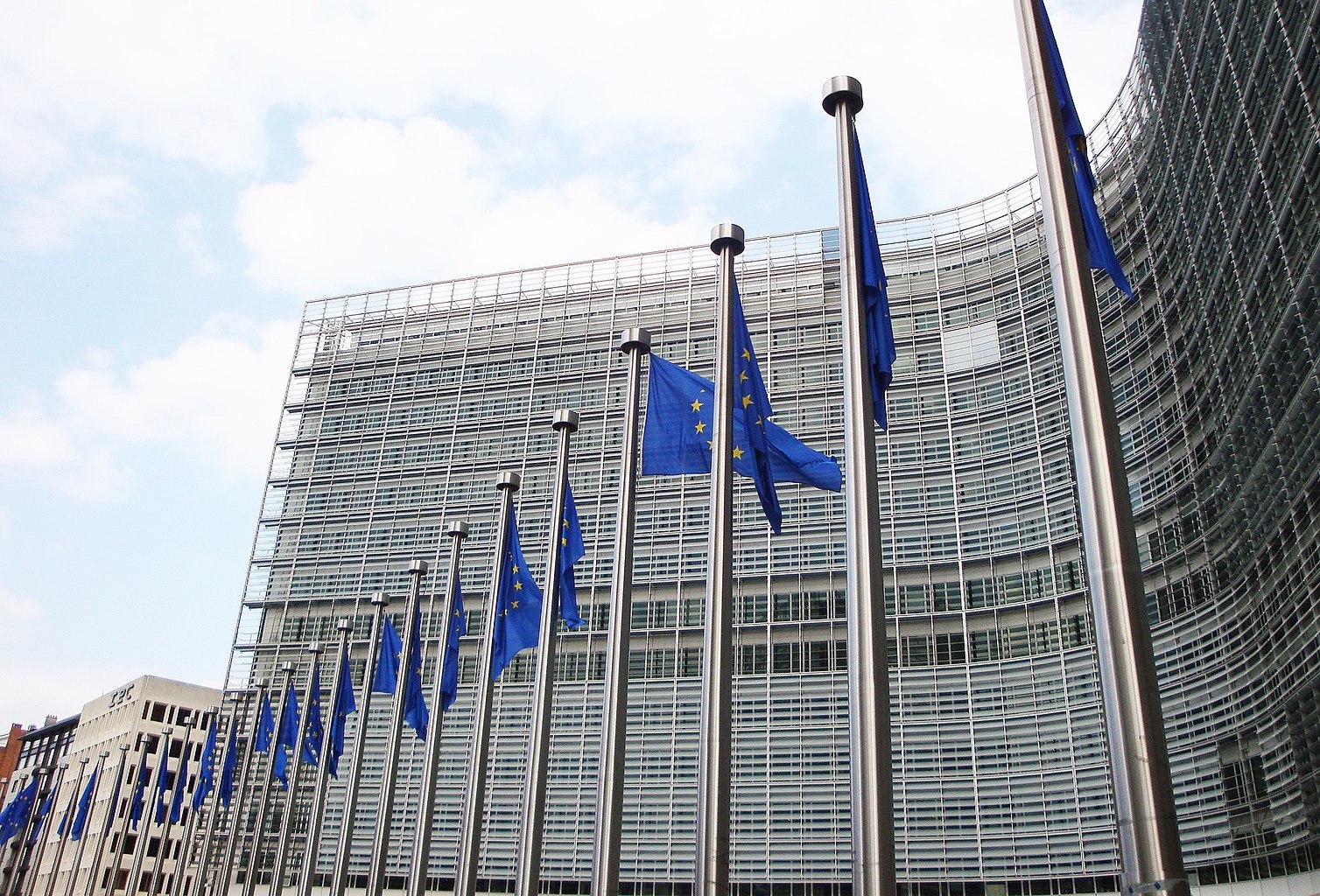
Published by The Lawfare Institute
in Cooperation With
Efforts to regulate artificial intelligence (AI) must aim to balance protecting the health, safety, and fundamental rights of individuals while reaping the benefits of innovation. These regulations will protect people from physical harms (like AI cars crashing), less visible harms (like systematized bias), harms from misuse (like deepfakes), and others. Regulators around the world are looking to the European Union’s AI Act (AIA), the first and largest of these efforts, as an example of how this balance can be achieved. It is the bar against which all future regulation will be measured. Notably, the act itself is intended only to outline the high-level picture of this balance. Starting in early 2023, accompanying technical standards will be developed in parallel to the act, and they ultimately will be responsible for establishing many of the trade-offs; early signs suggest that developing effective standards will be incredibly difficult.
Caught between an unwillingness to compromise on the protection of its citizens, geopolitical pressure to avoid impeding innovation, and the technical complexity of AI, the EU’s attempt to create technical AI standards will be more than a challenge—it may well be an impossibility. If that is the case, there are several possible outcomes, and the EU will need to seriously consider whether it is willing to overlook some state-of-the-art systems’ inherent flaws.
AI Standards: Between a Rock and a Hard Place
Historically, the EU has been willing to prioritize the protection of its citizens at the cost of innovation, but it may not be able to do the same with AI. The United States, China, and others seem more inclined to allow AI developers to proceed before technically viable standards can be set; the EU worries that acting more cautiously risks weakening its global relevance. While all regulation involves balancing this trade-off, it is unusual for the EU to be left with so little room for compromise.
EU product legislation is intentionally vague, and neither the original AIA proposal nor the Council of the EU’s recent one says much about how to manage these tensions. Both versions call for “suitable risk management measures,” and testing against “preliminarily defined metrics […] that are appropriate,” but give little indication as to what “suitable” and “appropriate” mean, allowing for both more or less restrictive implementations. While the texts give comprehensive frameworks of the kinds of requirements they would like, they do not provide technical details. The purpose of the AIA, like all EU product legislation, is to lay out essential requirements only specific enough to create legally binding obligations and to construct the institutional scaffolding for the enforcement and maintenance of these requirements.
It is up to harmonized standards to fill in the blanks left by the act, and the standard setters therefore bear the brunt of the responsibility for this compromise. They will both set the bar that systems must meet (by defining tests and metrics) and outline how the systems should be developed (by describing tools and processes that can be used). Adherence to harmonized standards is meant to offer an “objectively verifiable” way of complying with the essential requirements of EU legislation. Those who choose to follow them benefit from their associated “presumption of conformity,” meaning that complying with them is tantamount to meeting the essential requirements; those who choose not to follow them must typically prove their alternative solution is at least as good at protecting consumers as the harmonized standards. Despite their voluntary nature, they carry significant weight.
The development of the AIA’s harmonized standards faces one final constraint: technical feasibility. Putting pen to paper on standards for a technology as complex as AI was always going to be a challenge, but few appreciate how much of a challenge this is shaping up to be. Aleksander Madry, a machine learning researcher at the Massachusetts Institute of Technology, says of the technical feasibility of effective AI standards, “[W]e are not there yet.” Short of being especially restrictive, it is not clear whether AI standards can adequately protect consumers.
The Technical Feasibility of AI Standards
To ensure the protection of EU citizens (and us all), we want AI systems to respect humans’ safety and fundamental rights. Ideally, we would like to construct standards specifying how AI systems should be made and tested that respect these principles without being overly burdensome. Currently, however, we do not know how to make state-of-the-art AI systems that reliably adhere to these principles in the first place. More concerningly, we do not even know how to test whether systems adhere to these principles. Some simpler AI techniques may be manageable, but those driving recent advances—neural networks—remain largely inscrutable. Their performance improves by the day, but they continue to behave in unpredictable ways and resist attempts at remedy.
It is difficult to make neural networks reliable because, while their behavior is guided by data, they can learn from data in unintuitive and unexpected ways. They can learn to recognize the background of images rather than the object they are supposed to be identifying and learn to identify irrelevant and imperceptible patterns in pixels that just happened to work on the data on which they were trained. This quirk makes them equally hard to test, because it is not always clear what should be tested for and observers are continually surprised by ways in which these systems fail. The academic community was surprised by neural networks’ reliance on imperceptible patterns in pixels, which was discovered only when someone thought to test the systems for it. More recently, users found ways to make OpenAI’s ChatGPT, a neural network-powered chatbot, produce potentially dangerous content within hours, despite development and testing from the world’s leading researchers to avoid exactly these kinds of responses. It is nearly impossible to anticipate most of a neural network’s potential failure modes; this only becomes truer as the domains they are applied in become more complex. When it comes to crafting a standard to test for “robustness” or “accuracy,” as the EU is set to do, it is unlikely that a net of tests without significant holes can be created.
Some degree of recognition of these facts drives the requirements for human oversight and transparency (or explainability) of AI systems found in most AI regulation. The reasoning goes that if the systems cannot be made to behave well, humans should at least be given the tools required to verify and control the systems. Again, these requirements sound good in theory, but there is no obvious way to put them into practice. For example, making neural networks explainable has been notoriously hard: Zachary Lipton, a machine learning researcher at Carnegie Mellon University, says that “[e]veryone who is serious in the field knows that most of today’s explainable A.I. is nonsense”; a 2020 study has shown that the well-known tools LIME and SHAP (which are already limited to explaining individual decisions, rather than a systems’ general decision-making) can be abused to make untrustworthy models seem reliable; and a summary of the field has concluded that “it remains unclear when—or even if—we will be able to deploy truly interpretable deep learning systems [for example, neural networks].” If these measures worked, they would be useful, but for the moment they remain wishful thinking.
These difficulties (for example, when a Tesla on autopilot misses a stop sign and causes a deadly crash or when automated systems introduce bias through the entire hiring process) do not occur because engineers are ignoring known best practices, or avoiding well-known-but-expensive technical interventions, or skipping known tests that would have shown them their products were unsafe. No such best practices, interventions, or tests currently exist. And if the world’s leading engineers could not figure these out, then there may be little hope for EU standard makers.
That is not to say that the situation is completely hopeless. For narrow, low-risk applications, the imperfect tools currently available, combined with application-specific standards, could be enough. In addition, standards requiring monitoring, documentation, and record-keeping will be an invaluable part of weeding out problematic systems. Nonetheless, for neural networks applied to the kinds of high-risk applications the AIA tackles, it appears unlikely that anyone will be able to develop standards to guide development and testing that give us sufficient confidence in the applications’ respect for health and fundamental rights. We can throw risk management systems, monitoring guidelines, and documentation requirements around all we like, but it will not change that simple fact. It may even risk giving us a false sense of confidence. Faced with this reality, the EU will need to avoid creating weak, ineffective standards that rely on imperfect tests or transparency tools. There may be little to do but to use standards to set bars so general and high that they could in many cases preclude the use of neural networks.
What Happens Next?
Two of the three European Standards Organisations (ESOs), organizations independent of the EU, will be responsible for creating the AIA’s harmonized standards and confronting these technical issues head-on. The EU recently mandated that the European Committee for Standardisation and the European Committee for Electrotechnical Standardisation develop standards. These two organizations bring together the standards bodies of the EU member states, who put forward experts grouped in technical committees to draft standards. After consultation with stakeholders from industry and civil society, the standards are adopted by a vote among the standards bodies. Human rights organizations are already nervous about delegating to these industry-led organizations, which have more experience considering physical safety than human rights like equality and justice. Regardless, this process is expected to start in early 2023, and there are a few possible outcomes.
1. ESOs Develop Weak Standards
ESOs could develop standards that are too lax. Methods for evaluating AI systems do exist, but, as mentioned previously, they have been shown to be largely imperfect, and some can even be gamed to approve dodgy systems. Reliance on such methods would render the AIA toothless, and dangerous AI systems could make it to the market. If the development of standards is left entirely in the hands of ESOs, organizations with large industry interests, this outcome may be the most likely.
2. Standards Are Unhelpful or Unfinished
ESOs are far from the only organizations developing standards for AI. The standards that have been published so far, however, tend to stay high level, avoiding technical details. For example, the National Institute for Standards and Technology (NIST) in the U.S. is creating an AI Risk Management Framework, but it gets only as specific as explaining that “[r]esponses to the most significant risks, […] are developed, planned, and documented,” or mentioning technical terms with definitions too abstract to be directly actionable.
Work like NIST’s, which compiles and clarifies the many ideas floating around, is important. But the ESOs will be unable to use the same kind of ambiguity to skirt the near-impossibility of creating effective technical AI standards. Unlike others, they must develop requirements that are “objectively verifiable,” as confirmed by harmonized standards consultants (hired by the EU from Ernst & Young) before the standards officially become harmonized standards. If the standards-setting process is blocked by continual rejections from the consultants, the European Commission could decide to develop the standards itself.
If the process is blocked and the European Commission does not make its own standards, if the act comes into force before standards are finalized, or if vague standards somehow make it through the consultants, then the EU will have to navigate without the help of standards. In the case of systems requiring third-party assessment, the nationally appointed assessors, called notified bodies, will need to do this navigating. In the case of self-assessment, or if the notified body is deemed to have erroneously approved a product, the last line of defense is the nationally appointed market surveillance authorities, which hold the final say on which products are allowed on the EU market. No matter how strict or lax they end up choosing to be, a lack of helpful standards will lead to case-by-case decisions, creating expensive, lengthy processes, full of gray areas and inconsistent rulings—an undesirable outcome for all those involved.
3. The EU Bites the Regulatory Bullet
The struggle to create technical standards points to an uneasy fact—the essential requirements may simply be unmeetable for more complex AI systems. This is not necessarily a bad thing. What if, for sufficiently complex systems, there are no “suitable risk management measures”? Some observers may lament the cost to innovation, but surely it is also a sign that such systems should not be in use, and that market authorities (European or otherwise) should reject them. If the bare minimum expectations for AI systems have been established, and the systems do not meet that minimum, then it is not a sign the expectations need to be changed—it is a sign the systems should be changed. This may mean resisting geopolitical pressure and using less powerful, simpler AI systems until a deeper understanding of cutting-edge systems is obtained.
This is looking to be the case for neural networks, barring leaps in knowledge within the next few years. Without the ability to design effective tests and development processes specifically for them, the only viable solution may be to set high, possibly context-specific bars for their use, which avoids messy and poorly understood details. If developers hope to deploy neural networks, the onus will be on them to convince the world their system meets this bar.
While the EU may be the first to face this conundrum, it will certainly not be alone. All over the world where AI is being regulated, someone, somewhere will have to make decisions about which systems are good enough. Understandably, regulators want to have their cake and eat it too—they want to benefit from state-of-the-art AI systems that are also safe and trustworthy. Given that this may not be possible, they are only delaying the inevitable by creating ambiguous regulation. Tough calls will have to be made. Regulators will need to decide which concessions they are willing to make. And they will have to accept that, in some cases, avoiding risk is not a question of another risk management system, and it may require pumping the brakes on AI.