Explainable AI and the Legality of Autonomous Weapon Systems
This month, the Defense Advanced Research Projects Agency (DARPA) will assess the first phase of its Explainable AI program—a multi-year, multi-million dollar effort to enable artificial intelligence (AI) systems to justify their decisions.
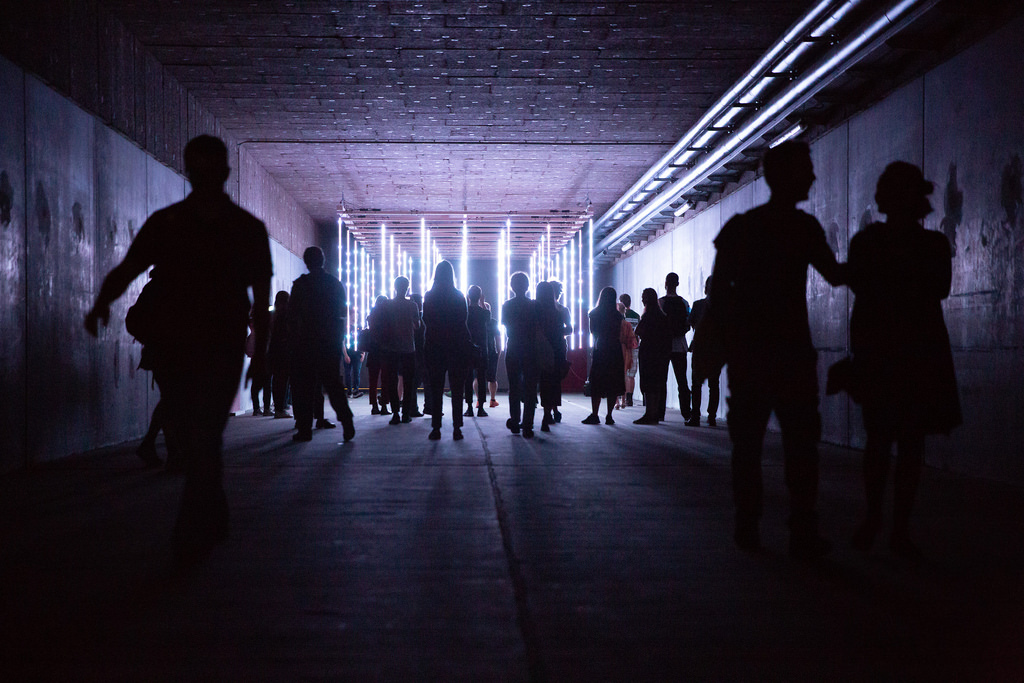
Published by The Lawfare Institute
in Cooperation With
This month, the Defense Advanced Research Projects Agency (DARPA) will assess the first phase of its Explainable AI program—a multi-year, multi-million dollar effort to enable artificial intelligence (AI) systems to justify their decisions.
To date, advanced AI systems have been able to classify objects, make predictions and improve their own performance through machine learning. One problem with AI systems, however, is that they often take the form of a black box that leaves users wondering how a particular system reached a certain conclusion, and whether its decisions can be trusted. Herein lies the promise of explainable AI: data scientists can train AI systems to better explain their behavior in a variety of ways. For instance, AI systems could list critical factors that influenced the analysis or produce visual displays that pinpoint determinative features from the raw data used to make a decision. Both of these mechanisms already exist, and AI researchers in the public and private sectors are working to further develop AI systems’ explanatory capabilities. If these efforts are successful, users—as well as those reviewing a given AI system—will have a more concrete understanding of how an AI system will perform going forward, and will be able to better assess the system’s reliability.
The potential uses of explainable AI are extensive, ranging from healthcare decisions and autonomous vehicles, to intelligence analysis and military logistics. While any application of explainable AI is exciting, it is particularly interesting as applied to autonomous weapon systems (AWS)—devices that military officials have been hesitant to deploy largely because of the black box nature of the underlying algorithms. As DARPA recently observed, “Explainable AI . . . will be essential if future warfighters are to understand, appropriately trust, and effectively manage an emerging generation of artificially intelligent machine partners.” Accordingly, from the perspective of someone deploying AWS, the benefits of explainable AI seem fairly straightforward. The more complicated question—and the subject of this post—is how explainable AI might impact assessments of the legality of AWS.
Background on AWS
As commentators have noted, AWS lack a universally accepted definition. While some states and organizations have proposed alternative definitions, many variations describe AWS as weapons that can detect, select and engage targets with varying degrees of autonomy. On one end of the spectrum are semiautonomous weapons, which can autonomously identify and engage a target upon being launched. However, a human is still “in the loop,” making the pivotal decisions about which target to engage and whether to authorize the attack. On the other end are fully autonomous weapon systems, which can search for targets, decide to engage them and execute that decision without any human involvement. Supervised AWS fall somewhere in the middle. They are capable of detecting, selecting and engaging targets on their own, but are supervised by a human operator, who retains the ability to intervene (i.e., there is a human “on” the loop).
For a more concrete image, consider an example from each category: homing munitions, missile defense systems and loitering munitions. Homing munitions are semiautonomous: A human operator determines the target before launch, and, once in the air, the weapon can independently track the target as it moves and update its flight course instantaneously. Next, missile defense systems run the full gamut of functional capacities. They identify incoming enemy targets, select which ones to engage and then destroy or derail them. But because a human operator can intervene when needed, missile defense systems are typically considered supervised AWS (though some systems have multiple settings and can be used in either semiautonomous or fully autonomous modes). Finally, loitering munitions can search for targets over a prolonged period of time, select which ones to engage and then execute the attack without any human intervention. To date, four fully autonomous loitering systems are in use, all developed by Israel,which has subsequently sold these systems to multiple states, including India and China.
Though the majority of today’s AWS are semiautonomous or supervised, the mere concept of fully autonomous weapon systems has stirred significant controversy. On one side, proponents point to the increased precision that AWS would enable, and suggest that autonomous weapons may better effectuate the intent of the humans who programmed and deployed them. Opponents, by contrast, have called for a complete, preemptive ban on fully AWS, citing (among other things) their unpredictability, vulnerability to algorithmic biases and capacity to inflict unintended harm—fears that also apply to supervised AWS, given that adequate and careful supervision is not always guaranteed.
The Legal Framework
In evaluating the legality of AWS, two questions arise under the law of armed conflict (LOAC): Is the weapon system inherently unlawful? And, if not, are certain applications unlawful?
To address the first question, LOAC establishes two central criteria. First, the weapon must not be “indiscriminate by nature.” Weapons that satisfy this requirement are those that “can be directed at a lawful target” and “[whose] effects are not uncontrollable.” Second, LOAC prohibits weapons that—by their nature—are likely to “cause superfluous injury or unnecessary suffering.” While some scholars and activists contend that AWS are intrinsically unlawful along either of these dimensions, many experts—including those at the Department of Defense—reject that position, explaining that as long as some uses might be permissible under the law of armed conflict, AWS are not unlawful per se. For that reason, much of the debate centers on the second question.
To determine whether specific uses of a weapon are lawful, LOAC focuses on three overarching principles: distinction, proportionality and precaution. To satisfy the first prong, operators must be able to direct the weapon in a manner that differentiates between civilians and combatants, as well as civilian objects and military objects. Next, the expected incidental harm from an attack (including injury to civilians and damage to their property) must be proportional to the perceived military advantage. Lastly, the party conducting the attack must take feasible measures to minimize harm to civilians.
Scholars have expressed concerns with AWS on all three fronts. Let’s start with distinction. To date, AWS have been unable to consistently distinguish between civilians and combatants in complex environments. As one report notes, although some loitering munitions systems are programmed to only detect and engage radars, they are unable to recognize the presence of civilians or civilian objects in the radar’s immediate vicinity. Likewise, in some settings, civilians and combatants may be highly interspersed in populated areas, limiting AWS’ capacity to narrowly engage their intended target. This is especially true when the targets are not wearing identifiable clothing (such as military uniforms). However, some experts believe that AWS can eventually be programmed to meet distinction requirements, and that limiting AWS’ use to rural, unpopulated areas may quell some of these concerns—as would instances when AWS are solely used to combat other unmanned AWS.
Turning to proportionality, it is unclear whether AWS are capable of making the inherently subjective judgments that necessarily factor into the cost-benefit analyses inherent to proportionality determinations. For instance, what constitutes a military advantage, and at what point does expected incidental harm outweigh that advantage? Opponents of AWS have questioned whether the weapon systems are capable of capturing—in real-time—the contextual cues that inform such determinations, as well as the propriety of AWS making (or attempting to make) “value judgments” that draw on human emotions and experiences. But at the same time, scholars point out that because military commanders already use systematic estimation methods to help make proportionality decisions, AWS could be pre-programmed to make such calculations as well: Operators could set various thresholds for “acceptable” levels of collateral damage for specific, pre-defined military objectives.
The debate surrounding precautionary measures mirrors that of proportionality—both principles call into question the ability of AWS to effectively monitor complex situations and make discretionary, value-laden decisions. Yet, like in the context of proportionality, it is also possible that AWS can be pre-programmed to make value judgments in a way that human operators see fit. In short, although AWS may not be inherently unlawful, they still have a ways to go before clearing LOAC’s hurdle.
Before turning to the potential impact of explainable AI, it is worth addressing two additional factors that lie at the heart of opponents’ concerns: accountability and meaningful human control. Without humans in (or on) the loop, it is not clear who should be held responsible when AWS go awry. In its Law of War Manual, the Department of Defense has attempted to minimize this concern by noting that LOAC “imposes obligations on persons,” not “on the weapons themselves.” In other words, “the person using the weapon” is the individual responsible for complying with the law—and the one who will be held accountable for LOAC violations. But even then, questions remain. Should the person responsible be the one who designed the system, or perhaps a specific aspect of the system? Should it be the official who authorized a weapon’s use in the field? And what if we have no way of telling what went wrong, or why? Animated by these uncertainties, many scholars, organizations and states have called for meaningful human control, in part to make the complex judgments involved in proportionality decisions, but also to ensure that human lives are not at the whim of black box algorithms. Though Defense Department policy requires AWS to “be designed to allow commanders . . . to exercise appropriate levels of human judgment,” it is not entirely evident how much human influence is “appropriate” in a given situation.
The Role of Explainable AI
Explainable AI will not single-handedly ensure the legality of AWS. But if DARPA’s vision can come to fruition—an outcome that seems at least plausible given the early success of various research groups—explainable AI has the potential to shift the discussion in the following ways:
Distinction
In the debate on AWS, one of the most commonly cited concerns is AWS’ (lack of) ability to distinguish between civilians and combatants in complex environments. While explainable AI would not change a weapon’s underlying capacity to distinguish, it would allow us to better understand this capacity’s contours and limits. For example, when testing AWS in simulated environments, operators would be able to decipher which stimuli the system recognized, what signals and cues the system considered and prioritized, and how the system incorporated novel stimuli into its analysis. Explainable AI could therefore reframe discussions about distinction to focus on whether a particular system’s decisions are sufficiently accurate to be deployed, rather than whether an operator will be able to predict how the system might react in the first place.
Proportionality and Precaution
As noted above, some scholars believe that AWS can be programmed to make proportionality calculations and engage in precautionary measures. Yet, as one Army judge advocate explains, the success of such programming depends on whether the commander is “confident [that] the system could conduct the [relevant] analysis with reasonable certainty.” Explainable AI could help provide the necessary check. Not only could commanders better ensure that the weapon systems were properly detecting and accounting for the pre-programmed factors, but AWS could theoretically be programmed to only engage a target if the system’s own explanation satisfied certain criteria. At the very least, if AWS could explain their decisions in the register of proportionality considerations (e.g., “target was not engaged because too many civilians were in the immediate area”), our focus might then shift to the accuracy and complexity of those determinations.
Accountability
In a world with explainable AI, operators would be able to make significantly more informed decisions about the predictability of AWS. Indeed, DARPA explicitly envisions that operators will test AWS in simulated settings, review a system’s explanations for its actions and decisions, and then decide “which future tasks to delegate” to the autonomous system. With a more informative and transparent approval process in place, the question of accountability appears less random. Responsibility for any problems associated with the weapon’s autonomy (such as a system’s failure to abort a mission in light of excessive expected losses, or a system’s unlawful targeting of civilian persons or objects) could be traced back to the individual(s) who made the delegation decision.
Meaningful Human Control
Given the black box nature of the algorithms underlying advanced AWS, it is difficult to grasp how much human control is truly necessary and in what ways human control is most effective. With explainable AI, operators would be able to know, at each point in the target engagement process, what outcome the autonomous weapon reached and why it arrived at that decision. Specifically, in the context of fully autonomous weapon systems, operators would have a more meaningful role in training and evaluating the systems, and could better understand how the system might react to variables on the battlefield. In the realm of supervised AWS, this transparency would facilitate more valuable and efficient oversight, as operators could more easily detect early missteps in the system’s targeting analysis and gain a better sense of when the system was functioning properly.
Of course, this entire discussion hinges on the success of DARPA’s Explainable AI program (and parallel projects in the private sector). But if researchers can unlock the promise of explainable AI, we might see a transformation in the uses and capacities of AWS, with corresponding implications for the analysis of AWS’ legality.