The Flawed Claims About Bias in Facial Recognition
Recent improvements in face recognition show that disparities previously chalked up to bias are largely the result of a couple of technical issues.
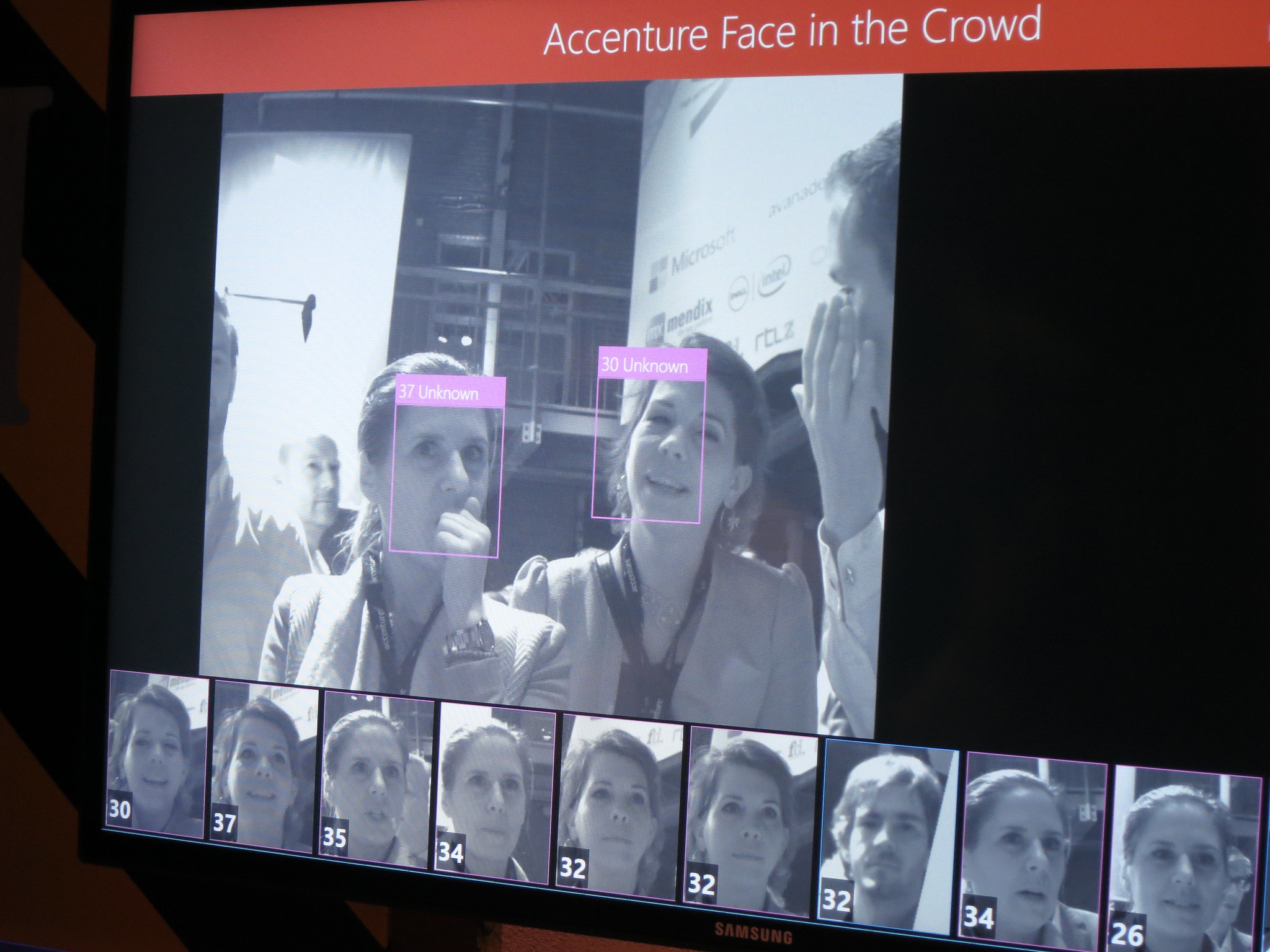
Published by The Lawfare Institute
in Cooperation With
If you’ve been paying attention to press and academic studies in recent years, you know one thing about face recognition algorithms. They’re biased against women and racial minorities. Actually, you’ve probably heard they’re racist. So says everyone from the MIT Technology Review and Motherboard to the ACLU and congressional Democrats.
There’s just one problem with this consensus. It’s wrong. And wrong in a way that has dangerous consequences. It’s distorting laws all around the country and handing the global lead in an important new technology to Chinese and Russian competitors.
That’s not to say that face recognition never had a problem dealing with the faces of women and minorities. A decade ago, when the technology was younger, it was often less accurate in identifying minorities and women. A 2012 study published by the IEEE found that when used on photos of men, whites, or the middle aged, the best commercial systems matched faces successfully about 94.5 percent of the time, but their success rates were lower for women (at 89.5 percent), Blacks (88.7 percent), and the young (91.7 percent).
These are the numbers that drove the still widely repeated claim that face recognition is irretrievably racist. In fact, that claim relies on data from an early stage in the technology’s development. And it has frozen the narrative by invoking a political and moral context that makes it hard to acknowledge the dramatic improvements in face recognition that followed the 2012 study. Racism, after all, is rarely cured by a few technical tweaks.
But face recognition algorithms are just tools. They may be accurate or not. The inaccuracies may be more common for some groups than others. But, like any tool, and especially like any new technology, improvements are likely. Treating face recognition differentials as an opportunity to explore society’s inherent racism, in contrast, doesn’t lead us to expect technical improvements. And that, it turns out, is why the “racism” framework is wrong. Recent improvements in face recognition show that disparities previously chalked up to bias are largely the result of a couple of technical issues.
The first is data. To be accurate, machine learning needs a big dataset. The more data you put in, the more accuracy you get out. Since minorities are by definition less well represented in the population than the majority, a lack of data may explain much of the “bias” in face recognition systems. That’s what tests suggest; algorithms developed in East Asia have done better than Western systems at identifying Asian faces—probably because they had more Asian faces to learn from. Luckily, this technical problem has a technical solution. Simply expanding the training set should improve accuracy and reduce differential error rates.
A second technical issue is how the images in question are captured. It’s obvious that good lighting improves face recognition. And, as camera makers already recognize, the wrong lighting or exposure can easily produce photographs that don’t do justice to people with darker skin. So simply improving the lighting and exposures used to capture images should improve accuracy and reduce race and gender differences.
In fact, that’s what more recent studies show. When it examined face recognition in 2018, the National Institute of Standards and Technology (NIST) found “massive gains in accuracy” since 2012, with error rates that fell below 0.2 percent with good lighting, exposures, focus and other conditions. In other words, used properly, the best algorithms got the right answer 99.8 percent of the time, and most of the remaining error was down not to race or gender but to aging and injuries that occurred between the first photo and the second.
Real-life implementations tell the same story. Two agencies that I know well—the Transportation Security Administration and Customs and Border Protection (CBP)—depend heavily on identity-based screening of travelers. As they rolled out algorithmic face recognition, they reported on the results. And, like NIST, they found “significant improvements” in face recognition tools in just the two years between a 2017 pilot and the start of operations in 2019. Those improvements seriously undercut the narrative of race and gender bias in face recognition. While CBP doesn’t collect data on travelers’ race, it does know a lot about travelers’ country of citizenship, which in turn is often highly correlated to race; using this proxy, CBP found that race had a “negligible” effect on the accuracy of its face matches. It did find some continuing performance differences based on age and gender, but those had declined a lot thanks to improvements in operational factors like illumination. These changes, the study found, “led to a substantial reduction in the initial gaps in matching for ages and genders”: In fact, by 2019 the error rate for women was 0.2 percent, better than the rate for men and much better than the 1.7 percent error rate for women found in 2017.
Of course, CBP offers face recognition the easiest of tests, a one-to-one match in which the algorithm just has to decide whether my face matches the picture on my passport. Other tests are harder, particularly the “one-to-many” searches that match photos from a crime to a collection of mug shots. These may have lower accuracy and, with less control over lighting and exposures, more difficulty with darker skin.
So technical improvements may narrow but not entirely eliminate disparities in face recognition. Even if that’s true, however, treating those disparities as a moral issue still leads us astray. To see how, consider pharmaceuticals. The world is full of drugs that work a bit better or worse in men than in women. Those drugs aren’t banned as the evil sexist work of pharma bros. If the gender differential is modest, doctors may simply ignore the difference, or they may recommend a different dose for women. And even when the differential impact is devastating—such as a drug that helps men but causes birth defects when taken by pregnant women—no one wastes time condemning those drugs for their bias. Instead, they’re treated like any other flawed tool, minimizing their risks by using a variety of protocols from prescription requirements to black box warnings.
Somehow, the algorithmic bias studies, and the journalists who cover them, have skipped both of these steps. They do not devote much time to asking whether the differentials they’ve found can actually cause harm. Nor do they ask whether the risk of harm can be neutralized when the algorithm’s output is actually used. If they did, face recognition wouldn’t have the toxic reputation it has today. Because it turns out that the harms attributed to face recognition bias are by and large both modest and easy to control.
Let’s start with the kind of harm most people imagine when they hear about bias in face recognition: an innocent man arrested or convicted because the algorithm falsely matched his image to a crime video. For all its prominence in popular imagination, there have been very few such cases in real life, and for good reason. Early bias studies, such as the 2012 IEEE study, found that certain populations were “more difficult to recognize.” That is, the systems were less good at finding matches in those populations. This is a difference, but it is not clear that it would lead to more false arrests of minorities. In actual use, face recognition software announces a match only if the algorithm assigns a high probability (often a 95 percent probability) to the match, meaning that weak or dubious matches are ignored. So it’s hard to see why being “difficult to recognize” would lead to more false arrests.
Even more important, it is stunningly easy to build protocols around face recognition that largely wash out the risk of discriminatory impacts. In many cases, they already exist, because false matches in face recognition were a problem long before computers entered the scene. The risk of false matches explains why police departments have procedures for lineups and photo arrays. Similar safeguards would work for machine matches: A simple policy requiring additional confirmation before relying on algorithmic face matches would probably do the trick. The protocol might simply require the investigating officer to validate the algorithm’s match using his or her own judgment, and eyesight. Indeed, this is so simple and obviously a mechanism for controlling error that one has to wonder why so few researchers who identify bias in artificial intelligence ever go on to ask whether the bias they’ve found could be controlled with such measures.
Of course, false matches aren’t the only way that bias in face recognition could cause harm. There are also false “no match” decisions. Face recognition is used more commonly for what could be called identity screening, which is done at the border, the airport, or on your iPhone to make sure the photo on your ID matches your face. False matches in that context don’t discriminate against anyone; if anything, they work in favor of individuals who are trying to commit identity theft. From the individual’s point of view, a risk of discrimination arises only from a false report that the subject and the photo don’t match, an error that could deny the subject access to his phone or her flight.
But once again, these consequences are vanishingly rare in the real world. Partly that’s because the government at least can control things like lighting and exposure, making technical errors less likely. Presumably that’s why the CBP report shows negligible error differentials for different races (or at least different countries of origin). And even where error differentials remain for some groups, such as the aged, there are straightforward protocols for reducing the error’s impact. As a practical matter, agencies that check IDs do not deny access just because the algorithm says it has found a mismatch. Instead, that finding generally triggers a set of alternative authentication methods—having a human double check your photo against your face and ask you questions to verify your identity. It’s hard to say that someone required to answer a few additional questions has been seriously harmed by the algorithm’s error, even if that error is a little more likely for older travelers. After all, face recognition software can also have problems with eyeglasses, and being required to take them off for the camera could be called discrimination based on disability, but in both cases, it’s hard to see the inconvenience as a moral issue, let alone a reason to discredit the technology.
In short, the evidence about bias in facial recognition evokes Peggy Lee’s refrain: “Is that all there is?” Sadly, the answer is yes; that’s all there is. For all the intense press and academic focus on the risk of bias in algorithmic face recognition, it turns out to be a tool that is very good and getting better, with errors attributable to race and gender that are small and getting smaller—and that can be rendered insignificant by the simple expedient of having people double check the machine’s results by using their own eyes and asking a few questions.
One can hope that this means that the furor over face recognition bias will eventually fade. Unfortunately, the cost of that panic is already high. The efficiencies that face recognition algorithms make possible are being spurned by governments caught up in what amounts to a moral panic. A host of cities and at least five states (Maine, Vermont, Virginia, Massachusetts and New York) have adopted laws banning or restricting state agencies’ use of face recognition.
Perhaps worse, tying the technology to accusations of racism has made the technology toxic for large, responsible technology companies, driving them out of the market. IBM has dropped its research entirely. Facebook has eliminated its most prominent use of face recognition. And Microsoft and Amazon have both suspended face recognition sales to law enforcement.
These departures have left the market mainly to Chinese and Russian companies. In fact, on a 2019 NIST test for one-to-one searches, Chinese and Russian companies scored higher than any Western competitors, occupying the top six positions. In December 2021, NIST again reported that Russian and Chinese companies dominated its rankings. The top-ranked U.S. company is Clearview AI, whose business practices have been widely sanctioned in Western countries.
Given the network effects in this business, the United States may have permanently ceded the face recognition market to companies it can’t really trust. That’s a heavy price to pay for indulging journalists and academics eager to prematurely impose a moral framework on a developing technology.