Governing Robophobia
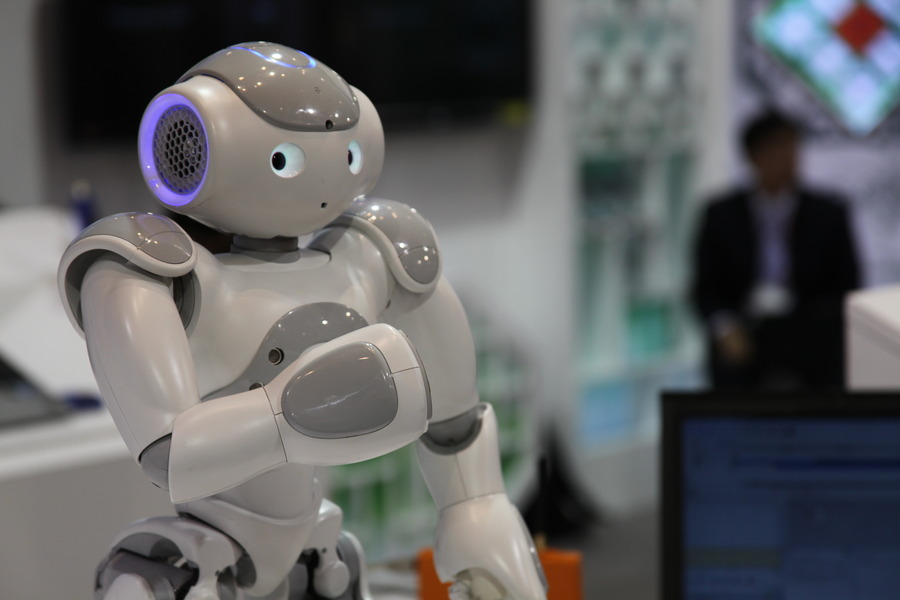
Published by The Lawfare Institute
in Cooperation With
More than two years before the public release of ChatGPT, one of us wrote an article in this publication (based on a longer law review article) about the extensive literature showing that humans exhibit a strong bias against algorithms. Entitled “Our Robophobia,” it argued that we “are biased against robots, and it is killing us.” Now, with news about artificial intelligence (AI) tools dominating headlines and public policy debates, this academic literature on human bias against robots carries new resonance.
Bias shapes how people use AI, and it shapes how policymakers seek to govern it. This research also suggests a looming risk for AI public policy: Policymakers’ bias against algorithms can result in AI policy that produces more societal costs than benefits.
Our Robophobia
When a self-driving car crashes, the outrage is everywhere: Newspapers run sensationalist articles, there are calls to shut down autonomous testing programs, and new public policies are instituted to protect the public. But when a human driver crashes, nothing is said and nothing changes. For example, when Uber’s self-driving car was involved in a deadly accident in Phoenix, Arizona’s governor shut down the test program. He said nothing about—and made no policy changes in response to—the more than 1,000 deaths on Arizona streets the same year, all at the hands of human drivers.
The public policy question of when to allow self-driving car systems on public roads is enormously consequential. Globally, more than a million people die in traffic accidents, almost all of those the result of human error. Any improvement over that record would constitute a massive public health victory. In fact, a recent study by RAND estimates that in the U.S. alone, hundreds of thousands of lives would be saved if self-driving cars were released onto public roads when they are 10 percent better than human drivers, as compared to waiting until they are 75 percent better than human drivers. But of course, that is not what we will do. As the head of Toyota’s self-driving car unit testified before Congress, people tolerate an enormous amount of harm at the hands of human drivers—“to err is human,” after all—but we “show nearly zero tolerance for injuries or deaths caused by flaws in a machine.”
This same phenomenon can be found in other domains. For example, patients in health care settings repeatedly say that they distrust AI advice for both diagnoses and treatment advice. In an experiment designed by doctors and lawyers (including one of us) to determine how people feel about AI in health care, a majority said they prefer medical advice from a human, even when they were told a robot would be more accurate, and even when they had the chance to meet with a human to go over the robot’s suggestions.
We see something similar in the law. It has been shown that lawyers have more faith in human-generated evidence, even though automated discovery tools are more accurate. In the military, a serious campaign is underway to stop autonomous weapons, even though they promise to significantly reduce mistakes and rule abuses.
It might be tempting to say that human bias against machines is just a natural desire for empathy. But studies have shown that AI chatbots can even outperform humans at providing empathy—as long as the human does not know it is talking to a bot. In a study published in the Proceedings of the National Academy of Sciences, researchers found that “AI-generated messages made recipients feel more heard than human-generated messages and that AI was better at detecting emotions.” They also found that AI “demonstrated superior discipline in offering emotional support.” However, when respondents knew that the communication came from AI rather than a human—through AI content labeling, for example—they “felt less heard.”
This is not to say that there aren’t good reasons to be skeptical of the way that AI tools will be deployed in society; not all AI technologies should be embraced unthinkingly. But these examples illustrate the breadth of evidence showing that human bias about AI affects decision-making, even when AI would confer clear benefits. Policymakers and the public are overly hesitant to trust a machine that promises better results than a human. This bias affects AI public policy.
Evidence-Based AI Governance
Evidence is among the strongest tools for counteracting bias. A careful review of existing academic research will help to produce a better understanding of the likely impacts of the technology. In many cases, the literature will show that costs of the technologies are overstated. In others, it may help to identify specific harms that are supported by evidence. For instance, even if current research shows that many of the alleged harms of generative AI in political ads are overstated, future research may indicate otherwise. In all cases, a better understanding of actual costs and benefits will create stronger, smarter governance regimes. The question is how to integrate evidence-based analysis into the policymaking process.
To ensure that AI public policy is rooted in evidence rather than bias, we suggest a policy agenda with three pillars: testing, evaluation, and learning. This approach has the potential to counteract our robophobia by aligning governance with research and helping policymakers keep pace as these new technologies evolve.
Testing
This agenda should start with testing. One option is for policymakers to enact legal frameworks that encourage policy and product experimentation. Experimentation will help develop a better understanding of the impacts of AI technologies. Because of human bias against AI, these experiments will likely indicate some uses of the technology that have significant benefits and minimal costs. They may also reveal other uses where costs exceed benefits, and where more restrictive public policy is warranted.
One experimental model is the regulatory sandbox, which states and other countries have used to enable companies to test new technologies in areas like financial services. The idea is to provide regulatory relief for a specific period of time to allow companies to trial new products. Another model is a policy experiment: A regulator can trial a new policy regime to develop a better sense of how it functions in practice. Policymakers can also consider hybrid experimental regimes, where they use new regulatory options to test new products. The idea is to encourage innovation in products and policies, but to also account for potential risks. Policymakers can limit the risks of a trial by making it finite in duration and scope, and closely monitoring it while it is occurring.
Monitoring is a critical component of experimentation. Experimental models that require close tracking of impact data will facilitate better understanding of how new technologies and new regulatory regimes play out in practice. New technologies and new regulatory models may have unanticipated costs and benefits. Experimentation can help to make these costs and benefits clearer, so long as robust monitoring mechanisms are built into the process. Where possible, governments and companies should publish data on the performance of these experiments so that others—including researchers—can learn from them as well.
Another element of testing could be encouraging more widespread red teaming to see how different models and products perform in practice. Red teaming is the practice of structured testing of an AI model, and it is already a common tool for AI platforms. It could be deployed by any firm developing AI products, and it need not look identical across companies. Larger companies with deeper pockets might be able to perform more extensive red team exercises than smaller companies with more limited resources. Any test of this sort should not only evaluate the risks of implementing an AI tool but also try to understand the relative risks of implementing an AI tool versus not implementing the tool at all. As we have discussed above, we should evaluate AI with reference to non-AI baselines. Red team exercises may reveal risks associated with AI products, but those risks might be outweighed by the costs of the status quo.
An emphasis on testing also points toward avoiding ex ante restrictions on AI technologies unless a clear cost is identified that exceeds the costs of inaction. As we have discussed, people may place disproportionate value on the potential harms of AI, even if those potential harms are likely exceeded by the harms of the status quo. If governments restrict the use of driverless cars, the effect might be to limit fatalities associated with driverless cars, but that outcome is desirable only if driverless cars produce more fatalities than cars with drivers.
Testing helps to produce that information, giving us data on the relative safety of driverless cars, AI-driven medical care, and AI-facilitated educational resources. It gives policymakers options to learn more about how innovations in those areas work in practice, without creating widespread risk on one hand or stalling innovation on the other.
Evaluation
The second prong of evidence-based AI governance is evaluation. Policymakers, engineers, and researchers can evaluate products and policies after they are implemented to determine how they perform in practice.
For example, policymakers can use cost-benefit analysis to evaluate the impacts of proposed legislation, and engineers can use cost-benefit analysis to evaluate the impacts of proposed products. This analysis need not be dispositive on the question of whether to pass legislation or release a product, but it may serve as a useful data point to better understand how a concept might play out in reality. Cost-benefit analysis is already used in the executive branch to understand the impacts of regulations, and companies routinely use A/B testing to gather data about product options. Using cost-benefit analysis to evaluate AI products could help assist decision-making in both the public and private sectors.
Several recent AI proposals would require companies to conduct impact assessments to understand the impacts of their products. But these assessments typically require companies to evaluate only the potential harms of their products. For instance, one recent proposal in California defines an impact assessment as a “risk-based evaluation.” Focusing solely on risk and harm incorporates only one side of the equation: Some products might come with significant risks but also significant benefits. If the benefits exceed the risks, then it may be desirable for a company to launch the product. A cost-benefit analysis also will account more accurately for situations in which AI tools produce better outcomes than non-AI tools. For this reason, policymakers would benefit from the use of cost-benefit analysis, rather than impact assessments, in their AI proposals. Indeed, early evidence suggests that using algorithms as the basis for policy interventions can have significant value, as measured by the benefit to society relative to their cost.
AI biases can also be addressed through transparency by governments and companies. Researchers, civil society organizations, and other experts will be unable to evaluate impacts accurately if they do not have the data they need in order to do so. Both companies and governments should seek to be as transparent as they can be in order to help others assess impact. In cases where either companies or governments are unable to share detailed data publicly, they should aim to make them public in summary form. Transparency is also key to experimentation.
Learning
Finally, an evidence-based AI policy agenda will require continuous learning. Matching public policy with evidence about how products and policies perform in practice calls for revisiting the evidence. In technology, the gaps in current research are large, and the iterative nature of product development means that even concepts that seem well established today may rest on a shakier foundation tomorrow. Public policy should invest in learning to try to keep pace.
That means that governments should create task forces to study specific AI issues, to educate themselves in how AI works in practice, and to develop technical skills in AI. They can also use capacity-building measures to promote cooperation and coordination between government agencies and offices. For instance, the Civil Rights Division at the Justice Department might benefit from working with the Office of Science and Technology at the White House or the National Institute of Standards and Technology at the Commerce Department in order to build cases on how AI is used to violate federal civil rights law.
Governments should also fund translational research that is focused on connecting the academy to policymakers. Translational research evaluates claims in the public policy conversation based on existing academic research and then seeks to develop policy frameworks that are guided by that research. The Knight Foundation has recently made significant investments in translational work, supporting the establishment of a center devoted primarily to this purpose. The government should do the same, allocating federal research dollars to individuals and organizations that are focused on translational work.
There is a deep need for more research on the efficacy of proposed policy interventions. For example, many policy proposals now include requirements for watermarks or disclaimers to help users identify that a particular piece of content was created with AI. But to date, there has been limited research suggesting that these types of disclosures work in practice. Do they constrain the behavior of bad actors? Do users observe the disclosures? If they do, are their views affected by the disclosures? The answers to these questions are far from definitive. Government funding for researching these issues will facilitate better understanding of how policy proposals are likely to work in reality.
Shifting the Narrative
Countering robophobia is not simply about what policymakers do but also about what they say. Robophobia, like other types of bias, is reenforced and entrenched by public and private narratives that put disproportionate emphasis on the harms of AI relative to beneficial and benign use cases. Policymakers can reframe how they speak about the rise of AI technologies to align their rhetoric with a realistic assessment of the risk.
One tactic for changing the narrative on AI is to use evidence-based governance as the foundation for evidence-based rhetoric. For example, if governments fund translational research on the impacts of both the technology and its regulation, they could hold hearings with the researchers to discuss their findings. Of course, in speeches, op-eds, and statements at hearings, policymakers may prefer to speak to compelling individual use cases rather than broad trends in data. The government uses public service announcements to advocate for all kinds of welfare-enhancing behaviors, and it could do the same here: “This is your brain without AI.”
Similarly, if lawmakers pass new laws to create regulatory sandboxes or policy experiments to try to gather data about how the technology and regulation will work in practice, they could draw data and use cases from these trials and incorporate them into their public messaging. Putting emphasis on relative costs and benefits—rather than focusing exclusively on one side of the ledger—will paint a more realistic picture of the impact of the technology.
Finally, government agencies with expertise in AI technology should provide briefings to other policymakers about how the tools work in practice. If policymakers are better informed about the practical realities of the technology, they may be less inclined to use robophobic rhetoric in their public communications. The National Institute of Standards and Technology is well positioned to conduct these types of briefings. Other agencies might be able to provide more specialized trainings about the use of AI in specific sectors, such as defense, health care, education, and the justice system. Educated policymakers might also be able to play a role in passing this knowledge along to their constituents, providing them with more evidence-based information about the impacts of the technology and its regulation.
***
Research suggests that our fear of robots—our robophobia—results in an unfounded skepticism about AI that in turn produces suboptimal outcomes. People decline to use AI tools even when doing so would benefit themselves and their communities. Public policy that adopts these biases will likely favor AI governance with disproportionate costs, since it will overvalue the risks of AI and undervalue its benefits. But evidence-based policymaking has the potential to counter our robophobia, creating public policy for AI rooted in a more grounded understanding of the technology’s actual impacts.