How to Think About Remedies in the Generative AI Copyright Cases
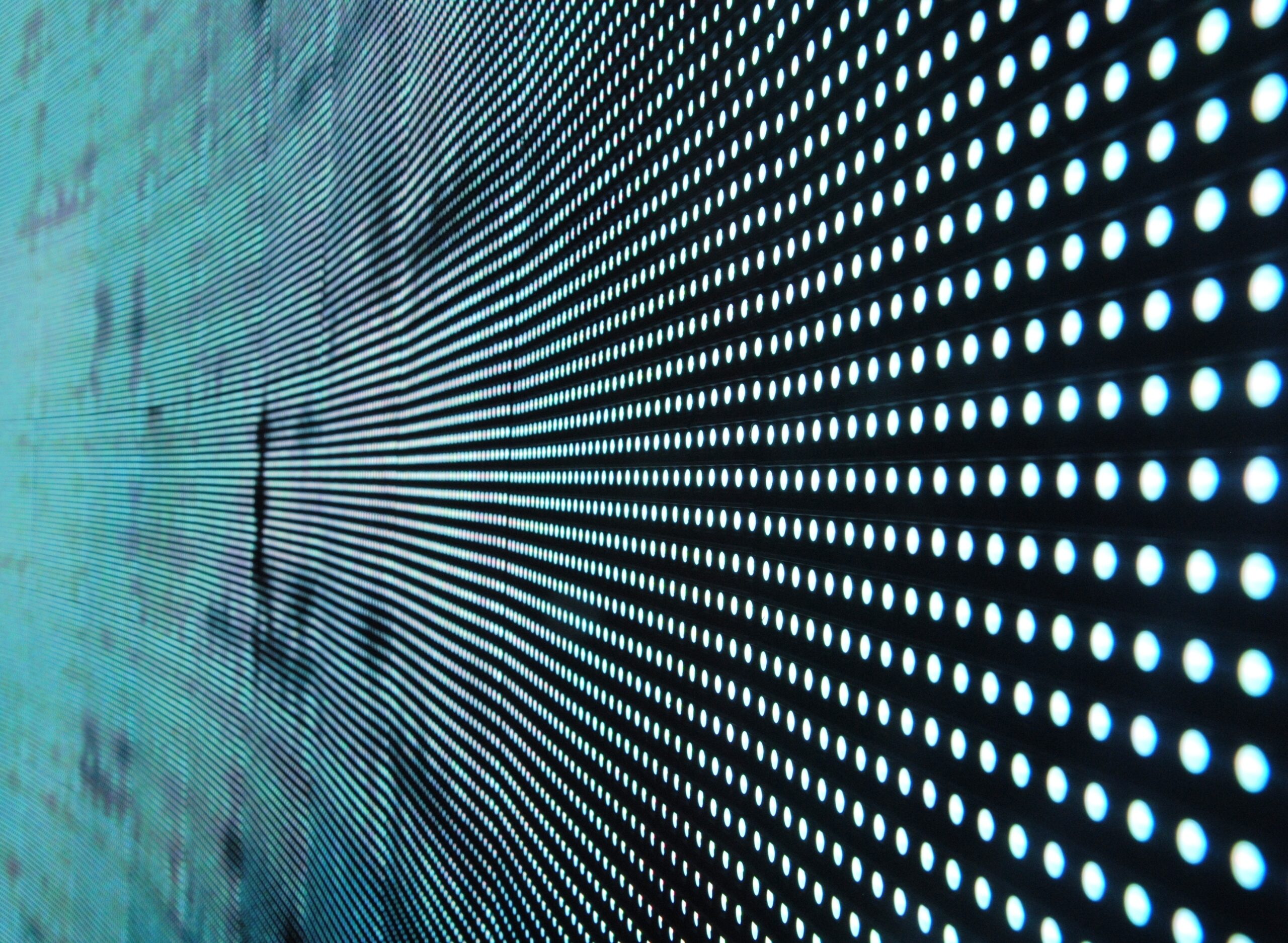
Published by The Lawfare Institute
in Cooperation With
The splashiest claims in the 16 (so far) lawsuits charging OpenAI and other developers of generative AI technologies with copyright-related violations are those that allege that making copies of in-copyright works for purposes of training generative AI models infringes copyright. Some commentators are convinced that these training data claims are sure winners; others are equally sure that the use of works to train generative AI models is fair use, especially if the data sets consist of digital copies of works found on the open internet. (Some lawsuits charge that generative AI models were trained on corpuses of “pirated books,” which may affect the developers’ fair use defenses.) It may be years before courts decide these and other claims in these lawsuits.
But let’s suppose that at least one of the plaintiffs succeeds with training data copyright claims. What happens then? So far, commentators have paid virtually no attention to the remedies being sought in the generative AI copyright complaints. This piece shines a light on them.
Virtually all complaints ask for awards of actual damages and disgorgement of profits attributable to infringement, prejudgment interest, attorney fees, and costs. Most ask for injunctive relief and any other remedy that the court may deem just. In these respects, the complaints are quite ordinary.
But three types of remedy claims merit special attention: claims for awards of statutory damages; court orders to destroy models trained on infringing works; and most bizarrely, court orders to establish a regulatory regime to oversee generative AI system operations.
Two Types of Statutory Damage Claims
Most of the generative AI copyright complaints include claims for awards of statutory damages. Such awards are authorized under U.S. law as alternative remedies to awards of actual damages and profits attributable to copyright violations.
Copyright owners generally like to claim statutory damages because these damages do not have to be tethered to the size of actual damages or profits attributable to copyright violations. The original rationale for this type of award in copyright cases was to allow right holders to obtain some compensation when it was too difficult or expensive to prove how much actual damage they had suffered because of infringement. Courts sometimes award statutory damages to approximate actual damages. When infringements are reckless or willful, awards might sensibly be set at some modest multiple over actual damages. But the relevant statutes do not require such tethering.
Two types of statutory damage claims are evident in the generative AI complaints. One type is for wrongful removal or alteration of copyright management information (CMI) (such as copyright notices) from copies of works used as training data. (That is, in the process of collecting or curating data sets or training models, CMI that was initially attached to works in the training data set may no longer be recognizably associated with those works in the trained models.) A second type of statutory damage claim is for copyright infringement. Some generative AI complaints ask for both kinds of statutory damages, while others ask for only one type of such damages.
The U.S. Supreme Court has ruled that copyright litigants have a constitutional right to have juries decide the amount of statutory damages to be awarded.
CMI Statutory Damages
The range of statutory damages awards for violating CMI rules starts at $2,500 per violation and goes up to $25,000 per wrong. (There are no criteria in the statute to provide guidance about where within that range such damage amounts should be awarded.) CMI plaintiffs need not have registered their claims of copyright with the U.S. Copyright Office to be eligible for CMI statutory damage awards.
Most generative AI complaints do not estimate the amount of CMI statutory damage awards that plaintiffs are seeking. An exception is the Doe v. GitHub complaint. It claims that Copilot, a code completion tool, violates CMI rules because it does not comply with open source license attribution requirements when suggesting lines of useful computer code to users. Copilot, which is a joint venture of GitHub and OpenAI, draws upon Codex, OpenAI’s large language model (LLM) that was trained on 5 billion lines of open source software code. (Microsoft, which owns GitHub and has invested heavily in OpenAI, is a fellow defendant.)
Here is the GitHub complaint’s explanation of the CMI statutory damages being sought:
Plaintiffs estimate that statutory damages for Defendants’ direct violations of the [CMI rules] alone will exceed $9,000,000,000. That figure represents minimum statutory damages ($2,500) incurred three times for each of the 1.2 million Copilot users Microsoft reported in June 2022. Each time Copilot provides an unlawful Output it violates [the CMI rules] three times (distributing the Licensed Materials without: (1) attribution, (2) copyright notice, and (3) License Terms). So, if each user receives just one Output that violates [CMI rules] throughout their time using Copilot (up to fifteen months for the earliest adopters), then GitHub and OpenAI have violated [the CMI rules] 3,600,000 times. At minimum statutory damages of $2500 per violation, that translates to $9,000,000,000.
It is questionable whether CMI violation claims of this sort will ultimately be successful. All three judges who have considered motions to dismiss CMI claims in generative AI cases have thrown them out, including the judge in the GitHub case, albeit with leave to amend. Merely removing or altering CMI is by itself not a violation of those rules. Removal or alteration must “induce, enable, facilitate, or conceal” copyright infringement to constitute violations.
Congress set the range of statutory damages available for CMI violations quite high in 1998 ($2,500 as the minimum) because it was concerned that tampering with CMI would enable widespread infringements of exact copies of protected works. Generative AI outputs are unlikely to bring about this result unless systems were trained to do so. Currently deployed models such as GPT4 or Claude rarely produce outputs that are exact copies of works used as inputs, or even substantially similar to them. However, it is sometimes possible for determined users to prompt models to produce potentially infringing outputs if the models have “memorized” that content.
Copyright Statutory Damages
Several generative AI complaints ask for awards of statutory damages for copyright infringement. Copyright owners must have registered their claims of copyright before infringement commenced to be eligible for these statutory damages. Three of the generative AI plaintiffs—namely, the New York Times, Concord Music, and Getty Images—may qualify for these damages insofar as they regularly register their works. The Authors Guild’s class-action lawsuit against OpenAI has limited the putative class to authors of timely registered works. Most class-action plaintiffs will not qualify for copyright statutory damage awards, even though some claim them anyway.
The copyright statutory damage range is wider than for CMI violations. The minimum award is $750 per infringed work and goes up to $30,000 per work for ordinary infringements. Awards are permissible, however, up to $150,000 per infringed work if infringement was willful. The only guidance the copyright statute provides is the directive that such awards should be “just.”
The generative AI plaintiffs who claim copyright statutory damages unsurprisingly assert that the defendants’ infringements were “willful,” thereby declaring their entitlement to maximum statutory damage awards per infringed work.
If the plaintiffs succeed in claiming that the uses of works as training data infringe copyrights, copyright statutory damage awards would almost certainly be staggeringly large as millions of works may have been used as training data.
In the generative AI cases, extremely large statutory damage awards seem difficult to justify given that the data sets used as training for most generative AI systems derive mostly from copies available on the open internet, albeit sometimes from the dark web. Actual damages arising from the use of internet-based works for model training purposes may be miniscule or nonexistent, although the generative AI plaintiffs argue that the outputs may reduce demand for the originals.
Nevertheless, the generative AI copyright plaintiffs are claiming statutory damages that could bankrupt most generative AI companies, although perhaps not those as large as Alphabet, Meta, and Microsoft. Those companies may also be able to afford to pay substantial licensing fees; many startups and nonprofit generative AI developers probably cannot.
Model Destruction
Four of the 16 generative AI copyright complaints explicitly ask courts to order generative AI defendants to destroy the models that were trained on their works. U.S. copyright law has long allowed impoundment and destruction of infringing items and of materials used in the process of making infringing copies. Other generative AI copyright plaintiffs may eventually amend their complaints to ask for this remedy. Or they may ask for impoundment and destruction as part of a requested injunctive order.
The New York Times’s complaint against OpenAI and Microsoft goes farthest in seeking model destruction as a remedy. It asks the court to order the destruction “of all GPT or other LLM models and training sets that incorporate Times Works,” even though OpenAI and Microsoft are the only defendants in that lawsuit. The threat of model destruction is, however, very real for these defendants.
One potential wrinkle in the Times’s and other model destruction claims is that training data sets are distinct entities from generative AI models. The entity that prepares training data sets is not necessarily the same as the entity that uses the data sets to train models. (Stability AI’s Stable Diffusion model for generating images was, for instance, trained on a data set prepared by a German nonprofit research entity known as LAION. That data set consists of links to images on the internet, not copies of the images as such.)
Once a model has been trained, the data set used in the training process may no longer have any utility. Or the data set may be used only infrequently for retraining, fine-tuning, or other purposes. Models do not generally contain recognizable expressions from the in-copyright works on which the models were trained because the training process changes how data in those works are represented in the model.
The separate existence of training data sets and models means that it is possible that use of works as training data might infringe copyrights, but the models might not. Thus far, courts have been unwilling to adopt a “fruit of the poisonous tree” theory of copyright liability when a preparatory use of protected works infringes, but a subsequent product derived in part from the earlier use does not. The New York Times and other plaintiffs in the generative AI copyright cases may try to persuade courts to adopt this theory so that developers of models trained on infringing data would not escape liability on this ground.
A second wrinkle with generative AI model destruction claims pertains to open source training data sets and models. Stability AI, for instance, built Stable Diffusion’s model on LAION’s open source training data set. It claims to have embodied Stable Diffusion in open source software that has been widely disseminated on the internet. Because of this, Stability may be unable to destroy all copies of the Stable Diffusion model, even if Getty persuades the court to order destruction of that model insofar as it was trained on Getty’s images. Although Stability cannot possibly track down every copy of this open source software, a court could order Stability to destroy copies of Stable Diffusion in its possession and to cease further use of Stable Diffusion.
A third wrinkle in the generative AI copyright destruction requests is that impoundment and destruction of infringing materials are discretionary remedies. That is, plaintiffs may ask courts to order such remedies. However, courts may decline to grant these remedies, just as they have discretion not to issue injunctions upon findings of actual or likely infringement.
Among the considerations that may weigh against issuing such an order are the existence of non-infringing materials in training data sets, substantial investments in trained models, and/or negative impacts of such an order on the public.
What About New Regulations?
The class-action complaint against Alphabet filed by several anonymous class representatives contains the most novel remedial request of the 16 complaints.
Here are the first three requests in this complaint’s remedial request:
- Establishment of an independent body of thought leaders (the “AI Council”) who shall be responsible for approving uses of the Products before, not after, the Products are deployed for said uses;
- Implementation of Accountability Protocols that hold Defendants responsible for Product actions and outputs and bar them from further commercial deployment absent the Products’ ability to follow a code of human-like ethical principles and guidelines and respect for human values and rights, and until Plaintiffs and Class Members are fairly compensated for the stolen data on which the Products depend; [and]
- Implementation of effective cybersecurity safeguards of the Products as determined by the AI Council, including adequate protocols and practices to protect Users’ Personal Information collected through Users’ inputting such information within the Products as well as through Defendants’ massive web scraping, consistent with industry standards, applicable regulations, and federal, state, and/or local laws[.]
This complaint also asks the court to order Alphabet to establish a monetary fund to compensate class members for its past and ongoing misconduct, “to be funded by a percentage of gross revenues from the Products” to be administered by a court-appointed official.
All I can say about this request for relief is “good luck with that.” It should be up to legislatures to establish regulatory regimes of the sort the complaint against Alphabet proposes.
Concluding Thoughts
All but one of the generative AI copyright lawsuits is likely years away from being definitively resolved. However, a Thomson Reuters lawsuit against Ross Intelligence for use of Westlaw headnotes as training data for Ross’s generative AI system for analyzing legal issues is scheduled to go to trial in late August 2024. Ross claims it made only fair use of the headnotes. A trial court denied the litigants’ cross-motions for summary judgment, finding that there were triable issues of fact on the infringement and fair use claims.
Thomson Reuters is among the generative AI plaintiffs that is asking for a court order to destroy a generative AI model trained on infringing data. Thus, we may know within the year how receptive courts will be to such remedy requests in generative AI cases. (For what it’s worth, I find Ross’s fair use defense quite persuasive. If Ross prevails, we will know no more about likely remedies in generative AI cases than we know today.)
None of the generative AI copyright complaints has explicitly asked a court to order generative AI developers to obtain a license from a collecting society, such as the Copyright Clearance Center, for permission to use in-copyright works as training data, subject to providing compensation for past and future uses of copyrighted works to train AI models.
The Authors Guild, which is the lead plaintiff in one class-action lawsuit, supports a collective license approach for authorizing use of in-copyright works as training data. Because no existing collecting society has obtained permission from all affected copyright owners to grant such a collective license, a court order of this sort would seem inappropriate.
The U.S. Copyright Office in late August 2023 issued a notice of inquiry asking for comments on, among other things, whether it should propose that Congress create a collective licensing regime for generative AI training and deployment. Its report on this and other generative AI copyright-related issues is likely to be published in the second half of 2024.
Whether a collective licensing regime for generative AI uses of in-copyright works is a good idea (as some believe) or a bad one (as others believe) depends on one’s perspective. Getting the economics right would be no small task. How the generative AI litigations play out is likely to influence what kind of legislation about generative AI copyright issues (if any) is ultimately proposed and enacted.