How the U.S. Government Can Learn to See the Future
The intelligence community should implement better geopolitical forecasting methods.
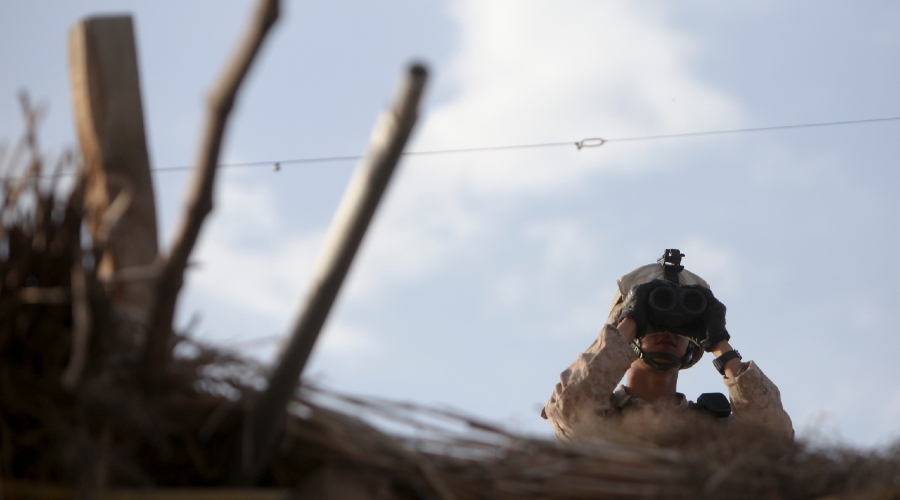
Published by The Lawfare Institute
in Cooperation With
Editor’s Note: Intelligence assessments are made under tremendous time pressure with imperfect information, so it is no surprise that they are often wrong. They can be better, but the intelligence community often fails to use the best analytic techniques. Julia Ciocca, Michael C. Horowitz, Lauren Kahn and Christian Ruhl of Perry World House at the University of Pennsylvania explain the current deficiencies in assessment techniques and argue that rigorous probabilistic forecasting, keeping score of assessments, and employing the “wisdom of crowds” produces better results.
Daniel Byman
***
In 1973, then-Secretary of State and National Security Adviser Henry Kissinger argued that policymaking could be reduced to a process of “making complicated bets about the future,” noting that it would be helpful if he could be supplied with “estimates of the relevant betting odds.”
Despite Kissinger’s plea for betting odds, forecasting efforts in the government today remain underdeveloped. The early failure to anticipate and prepare for the coronavirus, despite indicators that a pandemic was likely, has cost hundreds of thousands of American lives. Traditionally, the U.S. government relies on qualitative expert analysis, scenario-planning exercises and war games to assess the world. U.S. intelligence analysts play a critical role in informing policy decisions and help to keep the country and the world safe. Even in the best case, however, expert judgments often have limits because of constraints on the ability of any one individual to integrate information.
Moreover, the tentative, vague language and caveated conclusions that can emerge from scenario-planning exercises can fall into the age-old trap of “treating the conventional wisdom of the present as the blueprint for the future 15 to 20 years down the road.” Broadly, open-ended explorations through methods like war games or scenario planning are generally more helpful for identifying possibilities than for comparing the probabilities of these possible outcomes. For instance, in September 2019, the U.S. Naval War College convened a war game called “Urban Outbreak 19,” examining how a respiratory epidemic might spread in urban areas. The game identified key decision-making dynamics but did not deliver actionable probabilities for the scenarios. Quantitative probabilities might have been particularly useful in this case—the game was held months before the coronavirus pandemic.
There is a better way. Research over the past decade funded by the U.S. government demonstrates that “keeping score” by quantifying the probability that a potential event will or will not happen leads to improved forecasting accuracy. This is especially true when that scorekeeping is paired with training to reduce cognitive biases, as well as tools that combine the forecasts of many people together, harnessing the “wisdom of crowds.” These methods are not just for carnival games and stock trading. They can provide clearer insight on national security questions.
Unfortunately—for reasons related to bureaucratic politics and inertia, rather than science—successful experiments in new approaches to geopolitical forecasting fell by the wayside. Now is an opportune time for a change, as the Biden administration, early in its term, seeks to leave its mark on national security.
***
Rigorous probabilistic reasoning remains rare in the intelligence community. Sherman Kent, the “father of intelligence analysis,” once quipped, “I’d rather be a bookie than a goddamn poet” when one of his colleagues complained that using phrases such as “50-50 odds” would make the CIA sound like a “bookie shop.” Analysts do not often quantify their forecasts or confidence in these forecasts, aside from words of estimative probability such as “unlikely,” “likely,” “almost certainly,” and so on, which cannot distinguish that an “unlikely” event of 2 percent probability is twice as likely to happen as an “unlikely” event of 1 percent probability. Moreover, the vague boundaries between each stage of likelihood can contribute to critical strategic misunderstandings. For example, in 1961, when discussing the Bay of Pigs invasion, the Joint Chiefs of Staff estimated that the plan had only a 30 percent chance of success. However, the report for President Kennedy stated that the plan “[had] a fair chance of success.” Kennedy misinterpreted this characterization as a favorable rating, and even the authors of the report later acknowledged that this “vague language had enabled the strategic blunder.” Traditional methods also do not focus on falsifiability, specificity, crowdsourcing and performance tracking—even though these qualities empirically improve forecasting performance.
Keeping score, in contrast, means having forecasters use numeric probabilities to express their confidence, systematically tracking forecasters’ performance, and evaluating that performance using measures like “Brier scores” that track accuracy. Keeping score leads people to improve as forecasters, while also leading to accountability and transparency. Moreover, access to probabilistic forecasts could provide intelligence analysts with additional information to help them better aggregate the highly complex and often vague information that they must evaluate.
During the Obama years, the U.S. government initiated several quantitative geopolitical forecasting projects designed to complement traditional analysis methods. Between 2008 and 2018, the Defense Advanced Research Projects Agency (DARPA) and its intelligence community counterpart, the Intelligence Advanced Research Projects Activity (IARPA), launched a portfolio of a dozen prediction and forecasting initiatives. Some were very successful, such as the Aggregative Contingent Estimation (ACE) Program, which illustrated the potential utility of open-sourced forecasts by crowds. (Full disclosure: One of the authors, Michael C. Horowitz, was an investigator on the Good Judgment Project in IARPA’s ACE Program.) Another program reportedly had “the largest dataset on the accuracy of analytic judgments in the history of the [intelligence community],” and yet another was credited with predicting the 2013 Brazilian Spring and 2014 protests in Venezuela.
Despite promising results, nearly all of these programs ended during the Trump years. Some initiatives came to a natural end; others lost bureaucratic support. The U.S. government often faces challenges when seeking to transition promising research and development efforts into programs of record. In fact, there is a foreboding name given to this trend in the defense procurement world—the “valley of death.” Many U.S. government forecasting attempts failed to emerge from the valley because of failures to effectively communicate probabilities and their value to intelligence agency officials and policymakers, and bureaucratic resistance from those who feared forecasting efforts would upend their careers or the hierarchy of subject matter experts.
Rolling back or failing to advance these efforts was a mistake. These forecasting methods are relatively simple—and they work. First, crowdsourced forecasts leverage the wisdom of crowds in a market-like way that lends greater accuracy to the aggregated forecasts than to any individual analyst’s prediction. Second, keeping score opens new avenues for accountability and on-the-job training; by systematically tracking forecasting performance, a more meritocratic system can reward high performers, identify blindspots and room for improvement, and even expose sham “experts” for what they really are. Third, because the accuracy of these methods can be conceptually and empirically tested, they can be subject to research and development that makes it easier for these methods to continually improve. Finally, they make policymakers’ jobs easier by giving them what Kissinger always wanted: betting odds. With these odds in hand, a national security adviser or secretary of state (or both, in Kissinger’s case) could better understand the risk of certain events and develop more appropriate policy solutions. For example, a national security adviser who received a report that the risk of a war with China had risen from 10 percent to 20 percent might advise the president to increase efforts to deter conflict by shifting force posture, opening diplomatic channels, consulting with allies and partners, and/or other options.
Since the beginning of the coronavirus pandemic, the value of reliable forecasts about similar events is clearer than ever. The administration’s planned National Center for Epidemic Forecasting and Outbreak Analytics is a step in the right direction. Although the outlines of the center are still taking shape, it could become “the contagion equivalent of the National Weather Service” by using evidence-based forecasting techniques. However, expanding such forecasting efforts to cover other topics in other areas of government and integrating them within existing institutions and processes will prepare the United States for all kinds of threats and challenges, not just epidemics. Now is the time for the U.S. government to take geopolitical forecasting methods seriously again and to do it better.
The platforms and mechanisms for better forecasting exist; it is just a question of how they can be improved and implemented. In a recent white paper, we outlined critical steps for successful U.S. government implementation. They include building a forecasting platform with prediction polls rather than prediction markets, promoting broad participation by hosting classified and unclassified platforms, making efforts complementary to existing forecasting methods and exercising, and finding an effective bureaucratic home for the efforts. These efforts should be housed within the bureaucratic entity responsible for geopolitical forecasting—the intelligence community. Using explicit probabilities and encouraging analyst use of these probabilities in outputs will be necessary. If an intelligence community home is not possible, the Office of Science and Technology Policy represents another potential base.
Finally, efforts inside the government should be combined with open-source forecasting supported outside the government. Results from the ACE Program did not just illustrate the accuracy of crowdsourced forecasts; they showed that forecasters with access to only publicly available sources outperformed intelligence analysts when both forecasted on the same questions. These elite open-source forecasters had high levels of cognitive ability and a large degree of motivation, and they received training in probabilistic reasoning and cognitive debiasing.
***
This will not be quick or without difficulties. Probabilistic forecasting will face bureaucratic pushback from actors who see keeping score as threatening their work or status. President Biden, who is known for quoting W.B. Yeats, Seamus Heaney, and other Irish poets at length, appears to prefer poets over bookies—unlike Sherman Kent—but he now has the chance to improve how well the government sees the future by implementing new systems to promote crowdsourced forecasting methods. One of the Heaney lines Biden quotes most often implores the reader to believe in social progress: “a further shore is reachable from here.” By adopting better forecasting methods, the administration could better steer the ship of state toward that shore.