Lawfare Daily: Elliot Jones on the Importance and Current Limitations of AI Testing
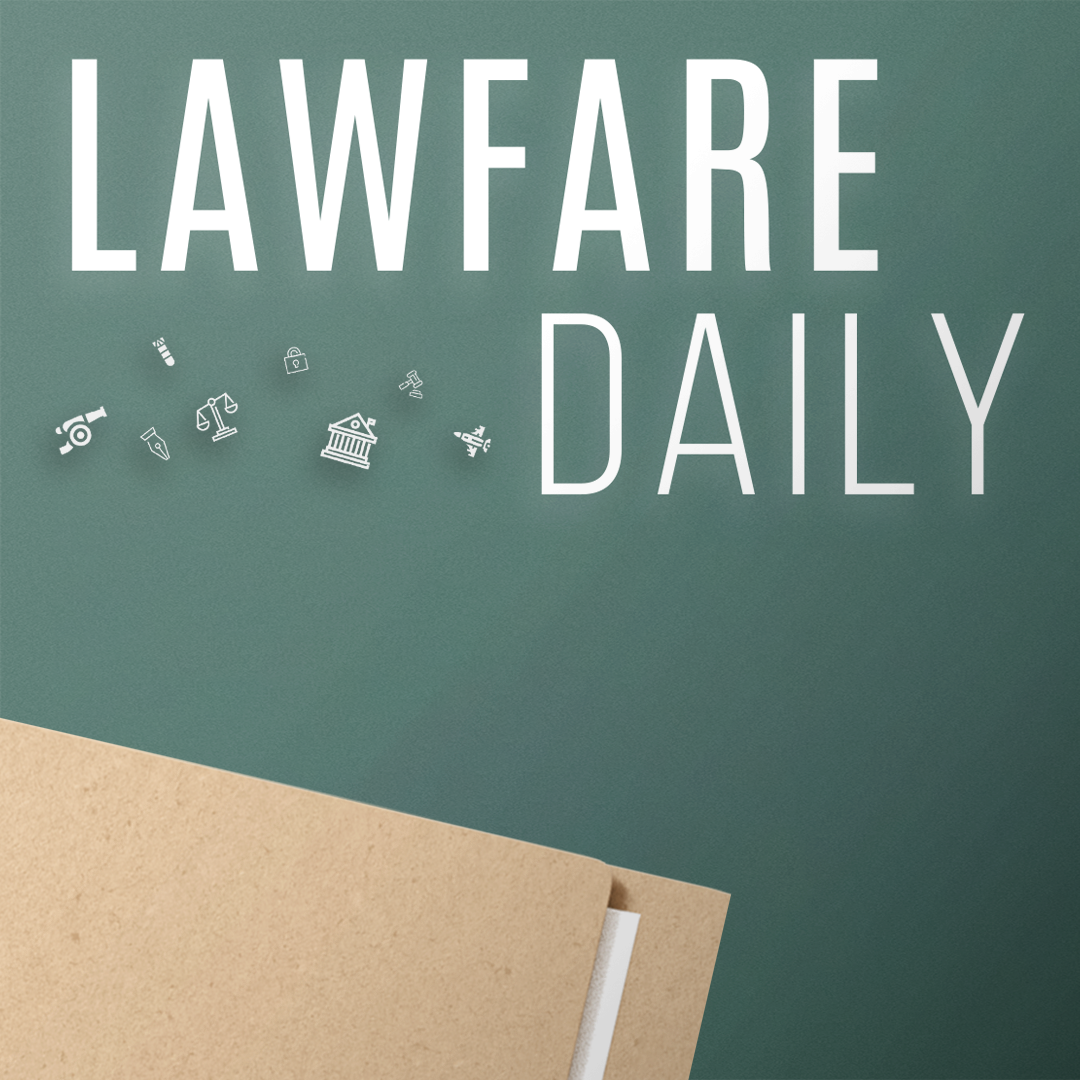
Published by The Lawfare Institute
in Cooperation With
Elliot Jones, a Senior Researcher at the Ada Lovelace Institute, joins Kevin Frazier, Assistant Professor at St. Thomas University College of Law and a Tarbell Fellow at Lawfare, to discuss a report he co-authored on the current state of efforts to test AI systems. The pair break down why evaluations, audits, and related assessments have become a key part of AI regulation. They also analyze why it may take some time for those assessments to be as robust as hoped.
To receive ad-free podcasts, become a Lawfare Material Supporter at www.patreon.com/lawfare. You can also support Lawfare by making a one-time donation at https://givebutter.com/c/trumptrials.
Click the button below to view a transcript of this podcast. Please note that the transcript was auto-generated and may contain errors.
Transcript
[Introduction]
Elliot Jones: On one level, there's just a generalization problem, or a kind of external validity problem of, a lot of the tests can do what they need to do. It can tell you, does the system have stored that knowledge, but translating that's whether the system has stored knowledge or not into, can someone take that knowledge? Can they apply it? Can they use that to create a mass casualty event? I just don't think we have that knowledge at all.
Kevin Frazier: It's the Lawfare Podcast. I'm Kevin Frazier, assistant professor at St. Thomas university college of law and a Tarbell fellow at Lawfare joined by Elliot Jones, a senior researcher at the Ada Lovelace Institute.
Elliot Jones: One thing we actually did hear from companies, from academics and from others is they would love regulators to tell them, what evaluations do you need for that? I think that a big problem is that there isn't actually had been that conversation between what are the kinds of tests you would need to do that regulators care about, that the public cares about, that are going to test the things people want to know.
Kevin Frazier: Today we're talking about AI testing in light of a lengthy and thorough report that Elliot coauthored.
[Main Podcast]
Before navigating the nitty gritty, let's start at a high level. Why are AI assessments so important? In other words, what spurred you and your coauthors to write this report in the first place?
Elliot Jones: Yeah, I think what really spurred us to think about this is that we've seen massive developments in the capabilities of AI in the last couple years, the kind of ChatGPT and everything that has followed. I think everyone's now aware how far some of this technology is moving, but I think we don't really understand how it works, how it's impacting society, what the risks are. And I think a few months ago, when we started talking about this project, there was the U.K. AI safety summit. There were lots of conversations and things in the air about like, how do we go about testing, how safe these things are, how these talking. But we felt a bit unclear about like where the actual state of play was there. We thought about, we looked at this and looked around and like, there was a lot of interesting work out there, but we couldn't find any kind of comprehensive guide to actually, how useful are these tools? How much can we know about these systems?
Kevin Frazier: To level set for all the listeners out there, Elliot, can you just quickly define the difference between a benchmark, an evaluation, and an audit?
Elliot Jones: That is actually a slightly trickier question than it sounds. At a very high level, a kind of evaluation is just trying to understand something about a model or the impact the model is having. And when we spoke to experts in this field who work on, in foundation model developers, in independent assessors. Some of them use the same definition for audits. Sometimes audits were a, you know, a subset of evaluation. Sometimes evaluation is a subset of audits. But I think for a very general sense for listeners, evaluations are just trying to understand the kind of, what can the model do? What behaviors does it exhibit? And maybe what the broader impacts of it has on, say, energy costs, jobs, the environment, other things around the model.
For benchmarking in particular, benchmarking is often using a set of standardized questions that you give to the model. So you say, we have these hundreds of questions, maybe from saying like an AP History exam, where you have the question, you have the answer, you ask the model the question, you see what answer you get back and you compare the two. And that allows you to have a fairly kind of standardized and comparable set of scores that you can compare across different models. So, a benchmark is a kind of subset.
Kevin Frazier: And when we focus on that difference between evaluations and audits, if you were to define audits distinctly, if you were trying to separate them from the folks who conflate it with evaluations, what is the more nuanced, I guess, definition of audits?
Elliot Jones: The important thing when I think, when I think about audits is that they are kind of well-structured and standardized. So if you're going into, say, a financial audit, you kind of, there's a process. The audits are expected to go through, to assess the books, to check out what's going on. Everyone kind of knows exactly what they're gonna be doing from the start. They know what end points they're trying to work out. So I think an audit would be something where there is a good set of standardized things you're going in, you know exactly what you're going to do. I know exactly what you're testing against. Audits might also be more expansive than just the model. So an audit might be a kind of governance audit where you look at the kind of practices of a company. Or you look at how the staff are operating, not just what is the model, what is the model doing? Whereas evaluations sometimes can be very structured as I kind of discussed with benchmarks, but can also be very exploratory where you just give an expert a system and see what they can do in 10 hours.
Kevin Frazier: We know that testing AI is profoundly important. We know that testing any emerging technology is profoundly important. Can you talk to the difficulties again at a high level of testing AI? Folks may know this from prior podcasts. I've studied extensively car regulations, and it's pretty easy to test a car, right? You can just drive it into a wall and get a sense of whether or not it's going to protect passengers. What is it about AI that makes it so difficult to evaluate and test? Why can't we just run it into a wall?
Elliot Jones: Yeah, I think it's important to distinguish which kind of AI we're talking about, whether it's narrow AI, like we're talking about a chest x-ray system, where we can actually see what the results we're getting. It has a very specific purpose. We can actually test it against those purposes. And I think in that area, we can do the equivalent of running it into a wall and seeing what happens. And what we decided to focus on in this report was foundation models, these kind of very general systems, these large language models, which can do hundreds, thousands of different things. And also they can be applied downstream in finance, in education, in healthcare, and because there are so many different settings, these things could be applied in so many different ways that people can fine tune them, that they can fine do applications.
I think the developers don't really know how the system can be used when they put them out in the world. And that's part of what makes them so difficult. To actually assess because you don't have a clear goal. You don't know exactly who's gonna be using it, how they're gonna be using it, how. And so when you start to think about testing, you're like, oh God, where do we even start? I think the other difficulty with some of these AI systems is we actually just don't understand how they work on the inside. With a car, I think we have a pretty good idea how the combustion engine works, how the wheel works. If you ask a foundation model developer. So why does it give the output it gives? They can't really tell you.
Kevin Frazier: So it's as if Henry Ford invented all at the same time a car, a helicopter, a submarine, put it out for commercial distribution and said, figure out what the risks are. Let's see how you're going to test that. And now we're left with this open question of what are the right mechanisms and methods to, to really identify those risks. So obviously, this is top of mind for regulators. Can you tell us a little bit more about the specific regulatory treatment of AI evaluations, and I guess we can just run through the big three, the U.S., the U.K., and the EU?
Elliot Jones: Yeah, so I guess I'll start with the EU, because I think they're the furthest along on this track in some ways. The European Union passed the European AI Act earlier this year. And as part of that, there are obligations around trying to assess some of these general purpose systems for systemic risk to actually go in and find out how these systems are working, what are they going to do? And they've set up this European AI office, which right now is consulting on its codes of practice that they're going to set out requirements for these companies that say, maybe you do need to evaluate for certain kinds of risks. So is this a system that might enable like more cyber warfare? Is there a system that might enable systemic discrimination? Is this a system that might actually lead to over reliance or concerns about critical infrastructure? So the European AI office is already kind of consulting around whether evaluation should become a requirement for companies.
I think in the U.S. and the U.K., things are both are much more on a voluntary footing right now. The U.K. back in, when would it have been November, set up its AI Safety Institute, and that has gone a long way in terms of voluntary evaluations. So that has been developing different evaluations, often with a national security focus around, say, cyber, bio, other kinds of concerns you might have. But that has been much more on a voluntary footing of companies choosing to share their models with this British government institute. And then somehow, and I think I'm not even really sure exactly how this kind of plays out. The issue is doing these tests. They've been publishing some of the results. But that's all very much on this, kind of, voluntary footing. And there has been kind of reports in the news that actually that's caused a bit of tension on both sides because the companies don't know how much they're supposed to share or how much they want to share. They don't know if they're supposed to make changes when the U.K. says, look at this result. They're like, cool. What does, what does that mean for us?
And I think the U.S. is in a pretty similar boat, maybe one step back because the United States AI Safety Institute is just, is still being set up. And so it's working with the U.K. AI Safety Institute. And I think they're, kind of, working a lot together on these evaluations. But that's still much more on a, the companies choose to work with these institutes, they choose what to share, and then the government kind of works with what it's got.
Kevin Frazier: So there are a ton of follow up questions there. I mean, again, just to for folks who are thinking at my speed, if we go back to a car example, right? And let's say the car manufacturers get to choose the test or choose which wall they're running into at which speed and who's driving all of a sudden we could see these tests could be slightly manipulated which that's problematic so that's, that's one question I want to dive into in a second.
But another big concern that kind of comes to mind immediately is the company's running the test themselves. Where if you had a car company for example controlling the crash test that might raise some red flags about, well, do we know that they're doing this to the full extent possible? So you all spend a lot of time in the report diving into this question of who's actually doing the testing. So under those three regulatory regimes, am I correct in summarizing that it's still all on the companies even in the EU, the U.K., and the U.S.?
Elliot Jones: So on the EU side, I think it's still yet to be seen. I think they haven't drafted these codes of practice yet. This kind of stuff hasn't gotten going. I think some of this will remain with the companies in the act. There are a lot of obligations for companies to demonstrate that they are doing certain things that they are in fact carrying out certain tests. But I'm pretty sure that the way the EU is going, there is also going to be a requirement for some kind of like third party assessment. This might take the form of the European AI office itself, carrying out some evaluations, going into companies and saying, give us access to your models, we're going to run some tests.
But I suspect that in, similarly to how finance audits work, it's likely to be outsourced to a third party where the EU office says, look, we think that these are reputable people. These are companies or organizations that are good at testing, that have the capabilities. We're going to ask them to go in and have a look at these companies and then publish those results and get a sense from there. It's a bit unclear how that relationship is going to work. Maybe the companies will be the ones choosing the third-party evaluators, in which case you have still some of these concerns and questions, maybe a bit more transparency.
In the U.K. and U.S. case, some of this has been the government already getting involved. As I kind of just said earlier, the U.K. AI Safety Institute has actually got a great technical team. They've managed to pull in people from OpenAI, from DeepMind, other people with great technical backgrounds, and they're starting to build some of their own evaluations themselves and run some of those themselves. I think that's a really promising direction because as you were kind of mentioning earlier about companies choosing their own tests. In this case, it's also having for like, for a benchmark, for example, if you've got the benchmark in front of you, you can also see the answers, so you're not just choosing what test to take. You've also got the answer sheet right in front of you. Whereas if you've got say the U.K. AI Safety Institute or the U.S. AI Safety Institute building their own evaluations, suddenly the companies don't know exactly what they're being tested against either. And that makes it much more difficult to manipulate and game that kind of system.
Kevin Frazier: And go into that critical question of the right talent to conduct these AI evaluations. I think something we've talked about from the outset is this is not easy. We're still trying to figure out exactly how they work what evaluations are the best, which ones are actually going to detect risks, and all these questions, but key to that is actually recruiting and retaining AI experts. So is there any fear that we may start to see a shortage of folks who can run these tests? I mean, we know the U.S. has an AC, the U.K. has an AC, again, that's AI Safety Institute. South Korea, I believe, is developing one. France, I believe, is developing one. Well, all of a sudden we've got 14, 16, who knows how many ACs are out there. Are there enough folks to conduct these tests to begin with, or are we going to see some sort of sharing regime, do you think, between these different testers?
Elliot Jones: I'll tackle the sharing regime question first. So we are already starting to see that. For some of the most recent tests on Claude 3.5, where Anthropic shared early access of their system, they shared it with the U.S. and the U.K. AC. And they kind of worked together on those tests. I think that it was the U.S. AC primarily getting that access from Anthropic, kind of getting, using the heft of the U.S. government basically to get the company to share those things, but leaning on the technical skills within the U.K. AC to actually conduct those tests. And there's been an announced kind of international network of AI safety institutes that's hopefully going to bring all of these together. And I expect that maybe in future we'll see some degree of specialization and knowledge sharing between all of these organizations that in the U.K., they've already built up a lot of talent around national security evaluations. I suspect we might see the United States AI Safety Institute looking more into say questions of systemic discrimination or more societal impacts. Each government is going to want to have its own kind of capabilities in house to do this stuff. I suspect that we will see that sharing precisely because as you identify, there are only so many people who can do this.
I think that's only a short-term consideration though, and it's partly because we've been relying a lot on people from, coming from the companies to do a lot of this work. But I think the existence of these AI safety teachers themselves will be a good training ground for more junior people who are coming into this, who want to learn how to evaluate the systems, who want to get across these things, but don't necessarily want to join a company. Maybe they'll come from academia, they'll be going to these ACs instead of joining a DeepMind or an OpenAI. And I think that that might kind of ease the bottleneck in future. And I kind of imagined that. I was talking earlier about having these third-party auditors and evaluators. I suspect we might see some staff from these AI safety institutes going off and founding them and kind of growing that ecosystem to provide those services over time.
Kevin Frazier: When folks go to buy a car, they, especially if they have kids or dogs or any other loved ones for all the bunny owners out there, or you pick your pet. You always wanna check the crash safety rating. But as things stand right now, it sounds as though some of these models are being released without any necessarily required testing. So you've mentioned a couple times these Code of Practices that the EU is developing. Do we have any sort of estimate on. when those are going to be released and when testing may come online?
Elliot Jones: Yeah. Yeah. So I think we're already starting to see them being drafted right now. I think that over the course of the rest of the summer and the autumn, the EU is going to be starting to create working groups of work through each of the sections of the Code of Practice. I think we're kind of expecting it to wrap up around next April, so I think by the kind of spring of next year we'll be starting to see at least the kind of first iteration of what these codes of practice look like. But that's only when the codes of practice are published. When we see these actually being implemented, when we see companies taking steps on this questions. Maybe they'll get ahead of the game. Maybe they'll see this coming down the track and start to move in that direction. A lot of these companies are going to be involved in this consultation, in this process of deciding what's in the Code of Practice. But equally, they could get published and then it'd take a while before we actually see the consequences of that.
Kevin Frazier: April of next year. I'm no, by no means a technical AI expert, but I venture to guess the amount of progress that can be made in the next eight months can be pretty dang substantial. So that's, that's quite the time horizon. Thankfully though, as you mentioned, we've already seen in some instances compliance with the U.K. AC testing, for example, but you mentioned that some labs maybe are a little hesitant to participate in that testing. Can you, so can you detail that a little bit further about why labs may not be participating to the full extent, or may be a little hesitant to do so?
Elliot Jones: Yeah, so, yeah, I, it's not quite clear which labs have been sharing and not sharing. I know that Anthropic has because they said it when they published Claude 3.5. To the others, it's kind of unclear. There's a certain opaqueness on both sides about exactly who is involved. But as to why they might be a bit concerned, I think there are some legitimate reasons, questions like say around commercial sensitivities, if you're actually evaluating these systems, then that means you probably need to get quite a lot of access to these systems. And if you're Meta and you're publishing Lama 300 billion, just out on the web, maybe you're not so worried about that you're kind of. Putting all the weights out there and just seeing how things go. But if you're an OpenAI or a Deep Mind and Anthropic, that's a big part of your kind of your value. If someone leaked all of the GPT-4 weights onto the internet, that would be a real, real hit to open AI. So I think there are legitimate security concerns they have around this sharing.
I think there's also another issue where, because this is a voluntary regime, if you choose to share your model, and the AI Safety Institute says it's got all these problems, but someone else doesn't. Then that just makes you look bad because you've exposed all the issues with your system, even though you probably know that the other providers have the same problems too, because you're the one who stepped forward and actually given an access and let your system be evaluated. It's only your problems that get exposed. So I think that's another issue with the voluntary regime of if it's not everyone involved, then that kind of disincentivizes anyone getting involved.
Kevin Frazier: Oh, good old collective action problems. We see them yet again and almost always in the most critical situations. So speaking of critical situations, I'll switch to critical harm. Critical harm is what is the focus of SB 1047. That is the leading AI proposal in the California state legislature that as of now, this is August 12th, is still under consideration. And under that bill, labs would be responsible for identifying or making reasonable assurances that their models would not lead to critical harm such as mass casualties or cyber security attacks that generate harms in excess of, I believe, 500 million dollars. So when you think about that kind of evaluation, is that possible? How do we know? that these sorts of critical harms aren't going to manifest from some sort of open model or even something that's closed like, Anthropic’s models or OpenAI's models.
Elliot Jones: I think with the tests we currently have, we just don't know. I think the problem is that, I guess there's a step one of trying to even create evaluation of some of these critical harms. There are some kind of evaluations out there like the weapons of mass destruction proxy benchmark, which tries to assess using multiple choice questions, kind of whether or not a system has knowledge of biosecurity concerns, cyber security concerns, kind of chemicals security concerns, things that maybe could lead down the track to some kind of harm. But that's, as it says, very much just a proxy. The system having knowledge of something doesn't tell you whether or not it's actually increasing the risk or chance of those events occurring.
So I think that on one level, there's just a generalization problem or a kind of external validity problem of all the tests can do what they need to do. It can tell you, does the system have stored that knowledge? But translating that, whether the system has stored knowledge or not into, can someone take that knowledge? Can they apply it? Can they use that to create a mass casualty event? I just don't think we have that knowledge at all. And I think this is where in the report, we talk about pairing evaluation with post market monitoring with instant reporting. And I think that's a key step to be able to do this kind of assessment of saying, okay. When we evaluated the system beforehand, we saw these kinds of properties. We saw that it had this kind of knowledge. We saw it had this kind of behavior. And at the other end, once it was released into the world, we saw these kinds of outcomes occur.
And hopefully that would come long before any kind of mass casualty event or really serious event. But you might be able to start matching up results on say this proxy benchmark with increased chance of people using these systems to create these kinds of harm. So I think that's one kind of issue. But right now, I don't think we kind of have that like, historical data of seeing how the kind of tests before the system is released match up to behaviors and actions after the system is released.
Kevin Frazier: As you pointed out earlier, usually when we think about testing for safety and risks, again, let's just go to a car example. If you fail your driving test, then you don't get to drive. Or if you fail a specific aspect of that test, let's say parallel parking, which we all know it's just way too hard when you're 15 or 16, then you go and you practice parallel parking. What does the report say on this question of kind of follow up aspects of testing? Because it's hard to say that there's necessarily a whole lot of benefit to testing for the sake of testing. What sort of addons or follow up mechanisms should we see after testing is done?
Elliot Jones: Yeah, I guess there's like a range of different things you might want to see a company do. I think for some tests where you see somewhat biased behavior or somewhat kind of biased outputs from a system. Maybe all that means is that you need to look back at your data, set your training system on say, okay, it's under underrepresenting these groups. It's not including say African Americans or African American perspectives as much. So we need to add some more of that data into the training. And maybe that can fix the problem that you've identified. That can go some way to actually resolving that issue. So there is some stuff you can do that's just kind of, as you're training the model, as you're testing it, kind of adjusting it and making sure that it's kind of adding onto that.
A kind of second step you can do is you might find that actually it's very difficult to fine tune out some of these problems. But that actually there are just certain kinds of prompts into a system, say someone asking about, how would I build a bomb in my basement that you can just build a safety filter on top that says, if someone asked this kind of question of the system, let's just not do that. As your evaluation tells you there is this harmful information inside the model where you can't necessarily completely get rid of it, especially if it's going to really damage the performance, but you can put guardrails around the system that make that inaccessible or make it very hard for a user to do that. And similarly, you might want to monitor what the outputs of the model is if you start seeing it mentioned, how to build a bomb. Then you might just want to cut that off and either ban the user or prevent the model from completing its output. I think when we get into slightly trickier ground and areas where I think companies haven't been so willing to do is on delaying deployment of a model or even restricting access to the model completely and deciding not to publish it.
I think one example of this is that OpenAI had a kind of voice cloning model, a very, very powerful system that could generate very realistic sounding voice audio, and they decided not to release it. And I think that's actually quite admirable to say, we did some evaluations, we discovered that this system could actually be used for say, mass spear phishing. If you think about, you get a call from your grandparents and they're saying, oh, I'm really in trouble. I really need your help. And it's just not them. And imagining that capability being everywhere. That's something really dangerous and they've decided not to release it. But equally, I suspect that as there are more and more commercial pressures, as these companies are competing with each other, there's going to be increasing pressure to, this system is a bit dangerous, maybe there are some risks, maybe there are some problems. But we spent a billion dollars training the system. So we need to get that money back somehow. And so they're going to push ahead with deploying the system. And so I think that's the kind of steps that a company might take that are going to get a bit more tricky around not just putting guardrails around it or tweaking it a bit, but actually saying, we've built something that we shouldn't release.
Kevin Frazier: I feel as though that pressure to release regardless of the outcomes is only going to increase as we hear more and more reports about these labs having questions around revenue and profitability. And as those questions maybe persist, that pressure is only going to grow. So that's quite concerning. And I guess I also want to dive a little bit deeper into the actual costs of testing. When we talk about crashing a car, you only have to take one car. Let's say that's between 20 grand and 70 grand, or for all those Ferrari drivers out there, we've got a half a million dollar car or something that you're slamming into a wall. With respect to doing a evaluation of an AI model, what are the actual costs of doing that? Do we have a dollar range on what it takes to test these different models?
Elliot Jones: To be perfectly honest, I don't have that. I don't know the amounts. I think the closest I've kind of seen is that Anthropic talks about when they were implementing one of these benchmarks. Even this off the shelf, kind of publicly available, widely used benchmark, that still required a few engineers spending a couple months of time working on implementing that system. And that's for something that they don't have to come up with a benchmark themselves. They don't have to come up with anything new. It's just taking something off the shelf and actually applying it to their system. And so I can imagine a few engineers at a couple months of time, and they pay their engineers a lot. So that's going to be in the like hundreds of thousands of dollars range, let alone the cost of compute of running the model across all of these different prompts and outputs. And that was just for one benchmark. And many of these systems are trained on lots of different benchmarks. There's lots of red teaming involved. When say a company like OpenAI is doing red teaming, they're often hiring tens or hundreds of domain experts to try and really test capabilities these systems have, and I can imagine they're not cheap either. So I don't have like a good dollar amount. But I imagine it's pretty expensive.
Kevin Frazier: I think it's really important to have a robust conversation about those costs so that all stakeholders know, okay, maybe it does make sense. If you're an AI lab and now you have 14 different AI safety institutes demanding you adhere to 14 different evaluations, that's a lot of money. That's a lot of time. That's a lot of resources. Who should have to bear those costs is an interesting question that I feel like merits a quite, quite a robust debate.
Elliot, we've gotten quite the overview of the difficulty of conducting evaluations, of the possibility of conducting audits, and then in some cases instituting benchmarks. One question I have is how concerned should we be about the possibility of audit washing. This is the phenomenon we've seen in other contexts where a standards developed or a certification is created. And folks say, you know, we took this climate pledge or we signed this human rights agreement. And so now you don't need to worry about this product. Everything's good to go. Don't ask any questions. Keep using it. It'll be fine. Are you all concerned about that possibility in an AI context?
Elliot Jones: Yes, I'm, I'm definitely concerned about that. I think the one thing we'd really want to emphasize is like, evaluations are necessary. You really have to go in and look at your system. Given the current state of play of this quite nascent field, these evaluations are only ever going to be indicative. They're only ever going to be, here are the kinds of things you should be kind of thinking about or worrying about. You should, with the current evaluations, not ever say, look, we did these four tests and it's fine. Partly because as we kind of discussed before, we haven't actually seen these in the real world long enough to know what those kinds of consequences are going to be. And without that kind of follow up, without that kind of post market monitoring, without that instant reporting, I would really not want anyone to say, this is a stamp of approval just because they passed a few evaluations.
Kevin Frazier: Thinking about the report itself, you all, like I said, Did tremendous work. This is a thorough research document. Can you walk us through that process a little bit more? Who did you all consult? How long did this take?
Elliot Jones: Yeah, sure. This was quite a difficult topic to tackle in some ways, because a lot of this, as a quite nascent field, is kind of held in the minds of people working directly on these topics. So we kind of started off this process by, between January and March this year, talking to a bunch of experts, some people working in foundation model developers, some people working in third party auditors and evaluators, people working in government, academics all working in these fields to just try and, you know, get a sense from them, people who have like hands on experience of running evaluations and seeing how hard they are to do in practice of repeating those things and seeing, do these actually play out in real life? So a lot of this work is based on just trying to talk to people who are kind of at the coalface of evaluation and getting a sense of what they were doing. As to exactly who, that's a slightly difficult topic. I think because this is quite a sensitive area, a lot of people wanted to be off the record when talking about this, but we did try and cover a fairly broad range of developers, of assessors, of these kinds of things.
Alongside that, we did our own kind of deep dive literature review. There are some great survey work out there. Laura Weidinger at DeepMind has done some great work kind of mapping out the space of like socio technical risks and the evaluations there. And so drawing on some of these existing survey papers, doing our own kind of survey of different kinds of evaluation. We worked with William Agnew as our technical consultant who has a bit more of a computer science background, so he could get into the nitty gritty of some of these more technical questions. So we tried to marry that kind of on the ground knowledge from people with what was out there in the academic literature.
I would say this is just a snapshot. This took us like six months, and I think some of the things we wrote are essentially already out of date. Some of the work we did looking at where are evaluations at? What is the coverage? People are publishing new evaluations every week. So this is definitely just a snapshot, but yeah, we tried to kind of marry the academic literature with speaking to people on the ground.
Kevin Frazier: So we, we know that other countries, states, regulatory authorities are going to lean more and more on these sorts of evaluations and they already are to a pretty high extent. From this report would you encourage a little more regulatory humility among current AI regulators to maybe put less emphasis on testing or at least put less weight on what testing necessarily means at this point in time?
Elliot Jones: To a degree, I think it depends what you want to use these for. I think in our report we try and break down kind of three different ways you might use evaluations as a tool. One is a kind of almost future scoping slash what is going to come down the road, just giving you a general sense of the risks, what to prioritize, what to look out for.
I think for that evaluations are really useful. I think that they can give you a good sense of maybe the cyber security concerns a model might have, maybe some of the bio concerns. It can't tell you exactly what harm it's going to cause, but it can give you a directional question of where to look. I think another way in which current evaluations can already be useful is if you're doing an investigation. If you're a regulator and you're looking at a very specific model, say you want to look at ChatGPT in May 2024, and you're concerned about how it's representing certain different groups. Or it's how it's being used in recruitment, say you're thinking about how is this system going to view different CVs and what comments is it going to give about, you know, a CV depending on different names. You can do those tests really well if you want to test it for that kind of bias. I think actually we're already kind of there and it can be a very useful tool for a regulator to assess these systems. But I think you have to have that degree of specificity because the results of valuations change so much just based on small changes in the system and based on small changes in context. Unless you have a really clear view of exactly what concern you have, they're not going to be the most useful.
The third kind of way you might use it is this kind of safety sign off, say, this is, this system is perfectly fine. Here's our stamp of approval. We are definitely not there. And I think if I was a regulator right now, one thing we actually did hear from companies, from academics and from others is they would love regulators to tell them, what evaluations do you need for that? I think that a big problem is that there isn't actually had been that conversation between what are the kinds of tests you would need to do that regulators care about, the public cares about, that are going to test. the things people want to know. And what are they going to build? And I think absent that guidance, industry and academia are just going to pursue what they find most interesting or what they care about the most. So I think right now it's incumbent on regulators, on policymakers to say, here are the things we care about. Here's what we want you to build tests for. And then maybe further down the line, once those tests have been developed, once we have a better sense of the science evaluations then we can start thinking about using it for that third category.
Kevin Frazier: And my hope, and please answer this in a favorable way, have you seen any regulators say, oh my gosh, thank you for this great report. We're going to respond to this and we will get back to you with an updated approach to evaluations. Has that occurred? What's been the response to this report so far?
Elliot Jones: I don't want to mention anyone by name. I feel like it'd be a bit unfair to do that here, but yeah, I think it's generally been pretty favorable. I think that actually a lot of what we're saying has been in the air already. As I said, we spoke to a lot of people kind of working on this, already thinking about this. And part of our endeavor here was to try and bring together conversations people are already having, discussions already have, but in a very comprehensible and public facing format, and I think the regulators were already, and are taking these kinds of questions seriously.
I think one difficulty is a question of regulatory capacity. Regulators are being asked to do a lot in these different fields. If I take the European AI office, for example, they've got, you know, I think maybe less than a hundred people now for such a massive domain. And so one kind of question is just, they have to prioritize, they have to try and cover so many different things. And so I think without more resources going into that area, and that is always going to be a political question of, what things do you prioritize? Where do you choose to spend the money? It's just going to be difficult for regulators to have the time and mental space to deal with some of these issues.
Kevin Frazier: And that's a fascinating one too, because if we see this constraint on regulatory capacity, I'm left wondering, okay, let's imagine I'm a smaller lab or an upstart lab. Where do I get placed in the testing order, right? Is OpenAI going to jump to the top of the queue and get that evaluation done faster? Do I have the resources to pay for these evaluations if I'm a smaller model? So really interesting questions when we bring in that big I word, as I call it, the innovation word, which seems to dominate a lot of AI conversations these days. So at the Institute, you all have quite an expansive agenda, and a lot of smart folks. Should we expect a follow-up report in the coming months, or are you all moving on to a different topic, or, what's the plan?
Elliot Jones: Yeah, I think partly we're wanting to see how this plays out, wanting to see how this field moves along. I think one question that we are thinking about quite a lot, and might, is this kind of question of third party auditing, third party evaluation? How does this kind of space grow? As we kind of mentioned a bit briefly in the report, there is currently a kind of a lack of access for these evaluators right now, a lack of ability of them to get access to these things, especially on their own terms, rather than on the terms of the companies. There's a lack of standardization. If you are someone shopping around as a smaller lab or a startup for evaluation services, it's a bit opaque with you on the outside, who is going to be doing good evaluations, who does good work and who is trying to sell you snake oil. And so I think that one thing we're really thinking about is how do we kind of create this auditing market where people on both sides, so you as the lab know you're buying a good service that regulators will trust, that everyone will work.
But also you as a consumer, when you're thinking about using an AI product, you can look at it and say, oh, it was evaluated by these people. I know that someone has kind of certified them, that someone has said, these people are up to snuff and they're going to do a good job. And so I think that's one thing we're really thinking about of how do you build up this market so that it's not just reliant on regulatory capacity. Because I think while that might be good in the short term for some of these biggest companies, it is just not going to be sustainable in the long term for government to be paying for and running all of these evaluations for everyone if AI is as big as some people think it will be.
Kevin Frazier: And thinking about some of those perspective questions that you, you all may dig into and just the scope and scale of this report. Is there anything in the off chance that not all listeners go read every single page. Is there anything we've missed that you want to make sure you highlight for our listeners?
Elliot Jones: I think one other thing I do want to bring up is the kind of lack of involvement of affected communities and all of this that we asked almost everyone we spoke to, so do you involve affected communities in your evaluations? And basically everyone said no. And I think this is a real problem that as I kind of mentioned before about what do regulators want? What does the public want in these questions? Actually deciding what risks we need to evaluate for and also what is an acceptable level of risk is something that we don't want to be left just to the developers or even just to a few people in a government office. It's something we want to involve everyone in to decide. There are real benefits to these systems. These systems are actually enabling new and interesting ways of working, new interesting ways of doing things, but they have real harms too.
And we need to actually engage people, especially those most marginalized in our society, in that question and say, what is the risk you're willing to take on? What is an acceptable evaluation mark for, for this kind of work? And that can be at multiple stages. That can be in actually doing the evaluation themselves. Have you got, if there are like a very diverse group of people red teaming a model, trying to pick it apart, have you got them involved in the goal setting stage? At that kind of product stage when you're about to launch something into the world, are you making sure that it actually does involve everyone who might be subject to that? If you're thinking about using a large language model in recruitment, have you got a diverse panel of people assessing that system and understanding is it going to hurt people from ethnic minority backgrounds? Is it going to affect women in different ways? So I think that's a really important point that I just want everyone to take away. I would love to see much more work in how you bring people into the evaluation process, because that's something we just really didn't find at all.
Kevin Frazier: Okay, well Elliot, you've got a lot of work to do, so I'm gonna have to leave it there so you can get back to it. Thanks so much for joining.
Elliot Jones: Thanks so much.
Kevin Frazier: The Lawfare Podcast is produced in cooperation with the Brookings Institution. You can get ad-free versions of this and other Lawfare podcasts by becoming a Lawfare material supporter through our website, lawfaremedia.org/support. You'll also get access to special events and other content available only to our supporters.
Please rate and review us wherever you get your podcasts. Look out for our other podcasts, including Rational Security, Chatter, Allies, and the Aftermath, our latest Lawfare Presents podcast series on the government's response to January 6th. Check out our written work at lawfaremedia.org. The podcast is edited by Jen Patja. Our theme song is from Alibi Music. As always, thank you for listening.