Lawfare Daily: Sam Manning on Benefits Sharing in the Context of AI
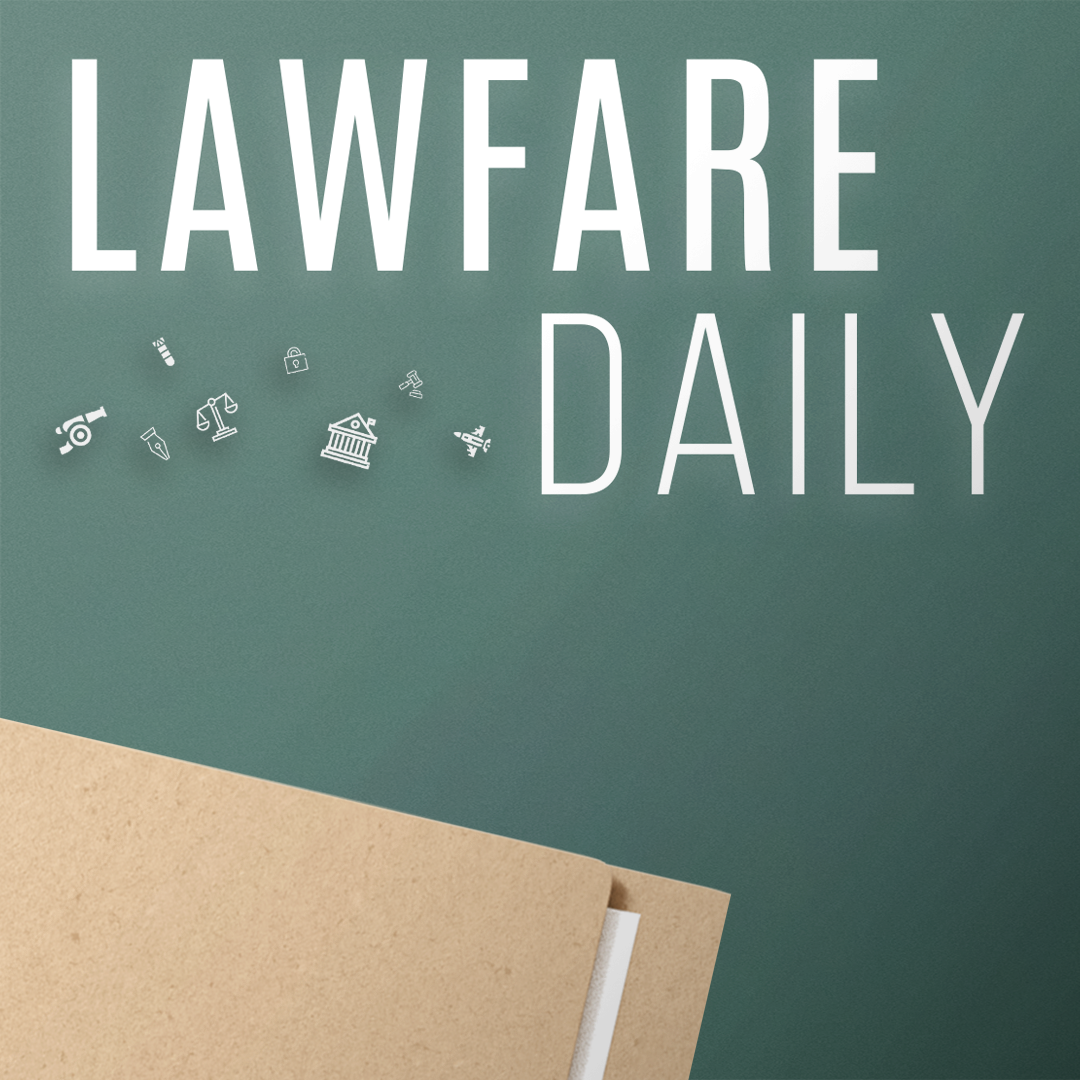
Published by The Lawfare Institute
in Cooperation With
Sam Manning, Senior Research Fellow at GovAI, joins Kevin Frazier, Assistant Professor at St. Thomas University College of Law and a Tarbell Fellow at Lawfare, to discuss his research on different options to share AI's benefits at the international level. The two also explore Sam's analysis of the incentives that may steer adoption of different benefits sharing strategies and his plans for future AI research.
To receive ad-free podcasts, become a Lawfare Material Supporter at www.patreon.com/lawfare. You can also support Lawfare by making a one-time donation at https://givebutter.com/lawfare-institute.
Click the button below to view a transcript of this podcast. Please note that the transcript was auto-generated and may contain errors.
Transcript
[Intro]
Sam Manning: So any kind of benefit sharing scheme that or approach here that is centered on distributing access to resources or access to models, for example, is going to contrast with a lot of other incentives.
Kevin Frazier: It's the Lawfare Podcast. I'm Kevin Frazier, assistant professor at St. Thomas University College of Law and a Tarbell Fellow at Lawfare, joined by Sam Manning of GovAI.
Sam Manning: As these systems become more capable and both their benefits and their risks become more salient to a wider range of actors around the world, the need for international agreements on AI governance may, you know, become increasingly necessary.
Kevin Frazier: Today we're diving into the complex landscape of AI's economic impacts and potential policy solutions, drawing on Sam's recent work. We'll be discussing his research on designing policy options to help ensure that advanced AI can foster broadly shared economic prosperity.
[Main Podcast]
Sam, I am fascinated by your scholarship. You are very much looking at the important future questions of AI's development and deployment, and I just want to start there. There's some folks who are fearful that we may be eventually heading into a sort of AI winter, or perhaps we're going to experience an AI bubble. Why do you think it's important or what gives you a sort of rationale for why we should be exploring these future questions of how AI is going to shape society?
Sam Manning: Yeah, so I think a lot of this is rooted in the stated intentions of some of the, kind of, most, you know, powerful and most, kind of, successful actors that are trying to drive this technology forward. So if you look at, like, for example, OpenAI's mission statement, it's to build artificial general intelligence, you know, tools that can outperform humans at most economically valuable work and make sure that benefits society, right?
So like, imagine the implications of success in that technological endeavor. And it's like, man, these are wild societal transformations that, kind of, probably warrant some type of proactive, you know, thinking here and probably policy action as well to try to make sure that while, you know, in the case that we have kind of this quite radical technological transformation, particularly around the economy and work, which is what most of my research and my, you know, work focuses on, then we're kind of starting now to build up some societal resilience, starting now to build up some anticipatory kind of policy thinking to hopefully shape this progress in a way that can make sure that it's a good thing, and we take advantage of all the kind of potentially wonderful, you know, benefits of technological progress, including in terms of like labor-saving technologies, while either mitigating some of the potential downsides and risks that comes with kind of ushering in a new you know, quite powerful technology or yeah, just kind of making sure that we're balancing risks and benefits here.
And so, yeah, that's the bigger picture motivation. I will say also, I think there's lots of things that are happening like right now, you know, so even if it's the case that these developers don't fully, you know, realize this technological ambition, there's still just like lots of really useful AI technologies that are, have been deployed into the world now and are having a real world impact, both positive and negative.
And so I think, and it's also a thing that, like, is on the mind of lots of policymakers and voters. And if we can try to do research to improve, like, you know, decision making by either these developers and by policymakers now, then that seems like a worthwhile, you know, endeavor.
Kevin Frazier: I really like this approach because I think if you had talked to anyone when Facebook was first created, and Mark Zuckerberg's talking about, oh, I'm going to connect the entire world and make this community of individuals across the entire nation and then around the world, folks were saying, ah, you know, sure. But you're just a guy in a garage or a dorm room. I'm not sure you're really going to realize that aspiration.
If we had taken him perhaps just a little bit more seriously, if we had taken that approach to anticipatory governance just a little more earnestly, then perhaps we wouldn't be dealing with a lot of the social ills from social media we've been experiencing.
And the importance of kind of taking Sam Altman, for example, at his word, as opposed to Mark Zuckerberg, is, as opposed to Mark just starting off in a dorm room, Sam has billions of dollars at his disposal, board laden with, you know, former Department of Defense officials with incredibly well-connected individuals.
So if we were ever going to say, hey, you know, let's just assume this technology might realize its aspirations. I think this is a pretty good place to start.
And one of the things that I'd also applaud you for doing is diving into those goals that the companies have set forth for themselves, and one of those that you point out is OpenAI really, kind of, stating an emphasis on wanting to make sure that AI is societally beneficial. And not just in a sort of amorphous sense, but really trying to correct upon previous examples of technology, perhaps benefiting just a few individuals, you know, or benefiting a few countries.
And so this really tees up your analysis into the idea of benefit sharing. And I guess I would love if you could just give us first a sense of what does benefit sharing mean at a high level? And what are perhaps some previous examples or some analogs we could look to for benefit sharing in a sort of technology context?
Sam Manning: Yeah, sure. And I guess just before getting into that, just one other point, too, in terms of, like, contrasting Mark Zuckerberg and Sam Altman's visions, I think it's also just the case that here now that there's just so much incentive to develop these technologies. Like, it's currently happening. We know that you get returns to scaling up the training of these systems. They can then become more useful. We know the problems that remain in terms of making them more useful to consumers and to businesses.
And I think there's just so much energy and investment being put in, that even beyond say, you know, Sam Altman or Mark Zuckerberg making these claims, it's like there's a lot to gain here and there's a lot of incentive and pressure to progress it. And so we might as well take that seriously and start, you know, thinking more seriously about the societal ramifications of that.
Now, yeah, one of the big ramifications and one of the, it's not just OpenAI here. I think, you know, if you look at Google DeepMind's mission now, I think it's something like build AI in order to benefit humanity, or something along those lines, right?
And so this concept of broadly distributing AI's benefits is part of these mission statements from developers. It's also a thing that has been pledged by governments around the world, and it's the first guiding principle in the UN's advisory body on AI. It's like, we want to ensure that benefits are, you know, shared around the world. This concept can be, yeah, you know, despite all these claims, it's a bit, like, ambiguous or nebulous around, like, what exactly that would look like and what exactly that means. And how it could be implemented, right?
And so there's, I think, yeah, some work that we're working on now is trying to sort of like flesh out what are some options here, and what are some plausible kind of paths forward, to ensure that the great things, the benefits of these technologies are distributed to, you know, all around the world and not just concentrated in kind of high income, you know, parts of the world, already well off places, and can be kind of applied for the benefit of others. Maybe I can get into some of the details on something in there. If that makes sense.
Kevin Frazier: Yeah. I'd love to dive into those weeds. I guess, first, to me, it is so important, though, to be doing this benefit sharing research, not only to think about taking companies, taking governments, taking advisory bodies at their word, for saying this is a priority.
But also looking back and seeing, all right, we've heard some prior instances, whether it's even just electricity, for example, when electricity was introduced, we really leaned on people like, for example, Lyndon Johnson to go out and electrify rural parts of America. It wasn't something that just happened. Electricity didn't suddenly appear in rural communities, but we needed folks to champion making sure these new technologies were in communities that were perhaps left behind, or perhaps would benefit most from these new technologies.
And in particular, we see this in an internet context, an area that I could, we could, share a whole nother podcast about that, but I'll spare my listeners for that future episode, and instead just emphasize that we see so much of a need, even just for internet access, continue on to today. And so how can we correct that previous failure to disperse benefits with respect to this really promising technology of AI?
And so when we think about benefits sharing, the obvious next question is, okay, what do you mean by benefits? What the heck does that mean? And thankfully, you all did an excellent job of teeing up three different examples of what benefit sharing could look like. Perhaps let's just start with your first idea for benefit sharing, which is access to AI resources. What would that look like?
Sam Manning: Yeah, so yeah, I think you're making reference to some kind of work in progress with my colleague Claire Dennis at GovAI and a number of other collaborators here as well, where we're trying to kind of lay out the motivations for benefit sharing and these three, you know, these kind of potential approaches and some challenges, to kind of break down three, the three main approaches here.
The first you mentioned is kind of sharing AI resources, right? AI development resources. So this could look like trying to more broadly accelerate the distribution of the inputs to AI development so that a broader swath of the global population and a broader kind of number of local economies can contribute to and shape and benefit from the development, fine tuning, evaluation of AI systems kind of, you know, at the forefront of their capabilities and make sure that they're able to apply them in ways that are locally beneficial and in line with, kind of, the aspirations of a diverse range of, you know, populations around the world.
So what are those inputs and resources? They’re, include things like compute, so, so computing resources. The compute is extremely valuable and scarce. If you look at just like Nvidia stock price over the past couple years, it's reflective in just the insane demand for, you know, frontier AI chips that are a major kind of input into AI development. Currently, as I mentioned those, access to those chips is quite concentrated in a small number of countries and with a small number of actors that are at the forefront of development of these systems, and that kind of prohibits, you know, lots of other actors from having the same kind of access to computing resources to develop systems.
Another input here is technical talent. Another thing that's increasing, you know, super scarce as well. You can see in various, like, news articles that at some of the leading AI companies you know, salaries can be in excess of a million dollars. There's lots of super high demand for technical talent. And ensuring that there's opportunity for kind of capacity building and training and skill development and technical assistance and technical transfer, those types of things to, you know, across the world is another kind of approach here.
Data and, you know, to train and fine-tune AI systems is another input. As well as just like information about training algorithms and procedures and kind of emerging techniques to get the most out of AI systems and be able to adapt them to local, kind of, context and use cases that will be especially beneficial.
So there's, yeah, a number of like, like within some of those, you know, there's of course, lots of thorny challenges associated with potentially sharing access to these things. But there is some plausible paths forward here. For example, like, in terms of compute sharing, you know, there's been a number of kind of regional like compute banks, or a national kind of compute bank proposals, put forward to kind of broaden access to computing resources, to researchers.
And there's been a number of proposals for trying to incentivize the development of applications. So, not just kind of the development of these, like, frontier, you know, general purpose models, but trying to do some market shaping and incentivize the development and provide the resources necessary to local economies to develop tools in line with, kind of, the, you know, needs of various populations around the world that are quite diverse and won't always be reflected if in the technology, if it's kind of just shaped by a number of the, you know, leading kind of actors that have quite a concentrated hold on some of these resources in the first place.
Kevin Frazier: Yeah. And I think it's so important too, to point out that AI as it's used in the United States or as it's used in Europe is probably going to look entirely different than how it may be used in, let's say, Kenya or Ethiopia or Thailand or many members of the Global South, where access to quality healthcare, for example, access to reliable tax information or legal information. That sort of low hanging fruit that AI is already showing a lot of promise in detecting, for example, medical diagnoses and making improvements inside sort of those basic medical tasks or basic legal tasks. That's already available. We're already seeing big improvements there. And if AI were made more broadly accessible, then it can be realized in a real fashion.
And to your point too, admittedly, when I picked up your paper and I saw benefit sharing, you know, there's a big degree of like, yeah, okay, sure. We'll see when we actually share benefits. But to your point, already in the U.S. we've seen examples. For instance, SB 1047, which Lawfare listeners have become well aware of, had a proposal for creating CalCompute, an idea of publicly available compute resources. In New York, they're creating the Empire AI compute. I guess New York is the Empire State and they thought that would be a creative name.
So these proposals are real, but to your point, there are just so many chicken and egg issues, right? If you send a bunch of compute to Uruguay, you gotta, if you build it, will they come is a big question. Does the tech talent suddenly just show up in Uruguay to make use of all of the compute? How do you stagger that sort of talent development with the provision of compute?
And I don't expect you to have answers to all of these questions. So is it sort of an attempt to shift the Overton window with respect to benefit sharing that you think is valuable to map out some of these different approaches?
Sam Manning: Well, I think it's, you know, most of what we're, you know, what I'm trying to think through, what some of my colleagues are trying to think through in this work is really just trying to play a clarifying role in like, okay, what are potential options for this, for executing on this kind of somewhat ambiguous topic that has been, you know, floated out a lot, but like, it's starting to become time here to actually really consider implementation. And making sure that if it's the case that we are to, you know, experience huge benefits from this technology, we still have, you know, over 700 million people around the world that live on less than $2.15 a day.
And if it's, if there's ever a time to try to, like, rebalance, you know, this and provide and try to correct some of the inequalities that exist in the world, why not take advantage of this, you know, while we're on the cusp of this, you know, technological revolution that can not just provide economic gains, but also, like you said, be really useful for use cases that have lots of positive externalities, like in healthcare and education in energy and agriculture and all of these things? And so that's kind of at least one of the motivations. There's others.
Some of the challenges. Yeah, so, they're very real and that's why I think we're hoping to just build a little bit of clarity about options and potential trade-offs and challenges. So, this interdependence between resources is definitely one of them.
It's probably not the case that equally distributing kind of frontier AI chips around the world is in everyone's best interest because not everyone may, you know, it might not be everyone's aspiration to, kind of, train your own frontier AI system from scratch, right? And also like this interdependence of, even if you were to do that, okay, well I still need to know how to, yeah, I need to have the skills to develop this thing. I need to have like the training data available in my language, and et cetera, you know, many other things.
And there's other, there's kind of a number of other challenges here as well. I mean, one that like really comes out is just geopolitical tensions and national security kind of tensions with this concept of benefit sharing.
So, in the U.S., we have, there's, you know, as I'm sure Lawfare listeners also know, right, there's these export controls around semiconductors for example. And there's, you know, I think this is something, you know, these frontier AI chips are especially scarce. Countries with a technological lead have a lot of interest in kind of preserving that lead, especially against some of their, kind of, geopolitical rivals. And so any kind of benefit sharing scheme that or approach here that is centered on distributing access to resources or access to models, for example, is going to contrast with a lot of other incentives.
And so I think it's important, you know, one of the other things that I'd love to talk about is how to kind of align some of these incentives a bit more and see how can some of these things, these sharing mechanisms actually be beneficial, not just for, you know, intended kind of recipients, but also for folks that are, you know, companies that are already benefiting quite a lot from these tools as well.
Kevin Frazier: So I want to circle back on those sort of incentives that we may see a line to make benefit sharing real. Let's quickly run through the other two benefits or the other two avenues for benefit sharing.
So second, you mentioned the possibility of sharing access to advanced AI systems, which again, some folks will say, oh my gosh, you're going to give you know, ChatGPT-4 away to, name that country, or name this country, and think, oh gosh that sounds problematic. But let's hear the positive use case for why this may be a good approach to benefit sharing,
Sam Manning: You know, lots of people around the world already are benefiting quite a lot from being able to use AI tools as mostly, you know, as consumers in their daily life, right? There's been broad, you know, and kind of widespread adoption of technologies like ChatGPT that, you know, signal this is providing some utility to folks and it is a good thing.
At the same time, there's also costs associated with accessing and using leading AI systems at scale. So for example, you know, to access like the most advanced models, you usually need to pay some type of subscription fee. And then in order to use the API, right, so to programmatically, like, call the model and build software around it that might enable more kind of entrepreneurship and business that might actually be fit for your local economy and kind of have lots of other positive spillovers, you need to then pay for the, you know, by token there, you know, so by kind of, yeah you're paying for the compute used to kind of run these systems, right?
And those can be constraining in terms of access. And so there's a number of ways that you could, you know, try to facilitate broader access to these systems to make sure that a broader swath of the global population is able to use these systems.
A couple of layers here, like I've just talked about sharing model access and there's a number of ways you can do that. You can do it through just like R&D to make inference costs more efficient. You could do it through government subsidies or differential pricing around the world, for example. You could do this through just making sure that open source development is able to kind of make, serve models in a way that is quite useful to folks.
All of this, kind of, hinges on having the requisite underlying, kind of, digital, fundamental digital infrastructure to use AI systems right into and to be able to build on top of them and access them in a way that allows you to benefit, you know, in line with kind of your aspirations. And this is a major kind of divide across the world where it's still the case that about a third of the global population isn't connected to the internet.
This is kind of one core right now. This is kind of one core, you know, need in order to use these systems and benefit from them the most, right? And so any kind of approach to benefit sharing. We outlined, sort of, these three distinct approaches: sharing access, sharing AI development resources, and then sharing like financial benefits as well.
But underlying all of them as a main kind of influencing factor is going to be how, it's going to be addressing kind of infrastructure gaps, you know, in terms of fundamental digital infrastructure to make any of those more effective at reaching some of the goals of benefit sharing, you know.
Kevin Frazier: And what I appreciate too about this line of inquiry is getting away from a narrative of AI takers and AI makers that's been advanced by some folks that we're just going to accept that AI is a product of some places and for some people, whereas everyone else will just be on the receiving end, waiting for AI to just show up or manifest. So, being more proactive about how can we make sure AI is furthering the common good for lack of a better phrase.
And thinking also of this financial benefit sharing, I think is maybe the most provocative or perhaps the most controversial. So what is this third approach to benefit sharing?
Sam Manning: Sure. Yes. So this third approach to benefit sharing has to do with distributing financial proceeds generated by AI development, right? And this could take the form of, for example, cash transfer programs that distribute AI driven profits, like, directly to individual recipients. It could involve distributing financial assets such as company shares or stock options, for example. Either way, this could be mediated through, for example, taxation and distribution via international organizations or kind of like coalitions of state governments, for example.
One of the more concrete benefit sharing proposals is one called the windfall clause, which was authored by a previous guest of yours, Colin O'Keefe and a number of other colleagues as well., where, in which kind of leading AI companies, AI developers, you know, valuable companies in the ASFI chain would commit to distributing windfall profits, you know, profits that were kind of unprecedented, you know, in history and kind of above some very high profit threshold.
So if it's the case that you were to develop these incredibly transformative technologies that generated tons of economic value, beyond some threshold, you would commit to, yeah, distributing these profits. And then, you know, beyond that, I think like the details of implementation are still, you know, needing to be worked out in many ways. But I think this is kind of one, one possible, at least, consideration.
I agree that there's, you know, lots of challenges associated with trying to actually implement something like this. One is, you know, it really, it would be kind of extremely expensive at sufficient scale, you know, to distribute financial gains. You'd have to, you know, assume that there's just this quite either, you know, monopolistic or like oligopolistic kind of like, AI developer that's capturing lots of profits in order to, you know, be able to then distribute them at sufficient scale around the world.
There's also just not very strong, you know, incentives, either for companies or for countries for that matter, to be distributing financial benefits in this way right now, I think, you know, many countries are, at least in the U.S., you know, isn't fulfilling its commitments to foreign aid, and these could be much, much smaller than what might be needed in future benefit sharing scenarios, for example. And so, yeah, this is one thing that we, at least, we discussed in the paper, but of course, yeah, there's many I think, it, yeah, there's lots of assumptions you need to put in place for this to be kind of a viable option and then challenges to it as well.
I think if it were the case that we ever reached a scenario where kind of a windfall clause-type distribution were necessary or were triggered, that kind of implies we've already gotten to a world with quite a lot of inequality. And so, you know, considering other options here to sort of pre-distribute the opportunity to benefit from AI development and the opportunity to kind of shape it and direct kind of technological development, in kind of accordance with local and diverse, kind of, aspirations, I think would be a good approach.
Kevin Frazier: Yeah, I think what's really exciting here is you all again are taking the companies at their word in terms of saying you want to distribute these benefits. You've said that time and again. Here's how we can potentially make this real. But now I want to give you the chance because I'm sure some listeners are thinking, Sam's research is fascinating. Each of these proposals sounds like they could take off in a certain universe, but that universe doesn't seem like one we live in currently.
So you mentioned, and you hinted at earlier, that there are some potential ways we can see the incentives of governments, of companies, of publics writ large, unite behind benefit sharing. What are those, some of those incentives that might say, hey, actually, I know this is unprecedented, or I know this is going to be difficult, but here's why it's actually in your interest, you, the United States, or you, OpenAI, to actually make sure one of these proposals becomes real.
Sam Manning: Yeah, so I think there's a few different kind of high-level motivations that one could have for this concept of benefit sharing or, you know, accelerating the spread of AI's economic and societal benefits. And so some of them are more, rely on quite a bit of altruism.
You know, I mentioned this economic motivation. We have hundreds of millions of people suffering from extreme poverty around the world. Ensuring that these benefits are shared with those most in need is a moral imperative, maybe an altruistic, kind of, -ly motivated driver for considering benefit sharing.
There's also a motivation around kind of enhancing self determination that we've talked about a bit. So, you know, benefit sharing could promote sort of, you know, self determination and AI development more broadly around the world by providing these resources so that countries can play a more active role in developing, understanding, and shaping the future of AI here.
Additionally, there's I think also an international cooperation motivation around benefit sharing. And so I think as these systems become more capable and both their benefits and their risks become more salient to a wider range of actors around the world, the need for international agreements on AI governance may, you know, become increasingly necessary right in, now and in the coming years.
I think it's not always the case, however, that many countries would see it as in their immediate national interests, especially those who are not quite, who are not heavily benefiting from the technology already, to agree to kind of international governance, you know, frameworks that imply different, you know, safety standards or, yeah, you know, risk mitigations and things like this that can help, kind of, govern the technology for good and reduce, you know, some of the risks that cross countries while they're not quite, you know, it's unclear if they're kind of benefiting from the technology as much as they could be. And so benefit sharing could be a thing that helps bring more countries into the fold, perhaps, on safety standards, sharing benefits here as a means to doing that.
And I think when you think about some of the downsides from AI technology around, for example, you know, misuse and things like this, things that are in, you know, responsible scaling policies that folks worry about at these different companies. These are cross country international risks that affect the global majority countries as well as actors in the Global North, and so it's in our interest to develop safety standards and governance frameworks to ensure kind of the responsible use of this technology.
I think, even if you're, you take that motivation, you're still the U.S. government, you're thinking, okay, well, you know, my main concern here is retaining our technological advantage, particularly against some of our geopolitical rivals, right against, you know, for example, you know, China, in terms of AI competition. I think it's the case that countries like the U.S. have an opportunity to kind of shape, you know, democratic-led kind of governance frameworks that include benefit sharing to bring, to build up more of a kind of a stronger kind of alliance of countries that are willing to commit to, whether it's AI governance standards or just kind of basic kind of, you know, principles of human rights around the application of AI, for example, that can help to, you know, reduce the risks of kind of a splintering of AI governance agreements between these sort of more democratic or human rights oriented governance frameworks and those that might be more authoritarian flavored and led by some of, you know, these leading, you know, the U.S.’s sort of geopolitical rivals.
And so I think there's by, you know, I don't think there's a one to one kind of trade off here where benefit sharing is costly to, you know, national security or to continuing to benefit from these technologies, you know, yourself.
Kevin Frazier: Well, and I also think it's just really fascinating to sort of frame that almost in a, let's say to modify the term Cold War and refer to it as a sort of artificial war, right?
How do we prevent these sort of proxy battles about, oh, take our AI model, but not their AI model and try to get ahead of the geopolitical instability that that sort of arrangement of each sphere of influence fighting for its AI model could develop. And then obviously that leads to a panoply of other public issues and national security issues. So really interesting to see how benefit sharing may indeed be a key national security interest of a lot of different countries.
And before I let you go, though, Sam, I need to know a couple things, and in particular, I'm very interested to know, you've covered benefit sharing, you've historically done research on labor displacement, what is next on your agenda? What are you thinking about, given your sort of perspective analysis of AI?
Sam Manning: Yeah, I think there's a lot more clarifying work to be done on this concept of accelerating the distribution of AI’s benefits in a way that is incentive-aligned with lots of different actors. And so work on that, I think, is going to be, you know, it's kind of still on the docket here, and there's lots of work to be done to kind of clarify options, think more about implementation and weigh these tradeoffs between different actors.
I think there's also this kind of glaring gap in some of this benefit sharing work, where there's currently not, there's not many explicit kind of forums for diverse stakeholders and perspectives especially those from global majority countries, from around the world, to actually clearly say, here's what we would like from any type of benefit sharing, you know, scheme, you know, approach, that kind of is part of some type of governance framework. And so I think work trying to figure out, trying to figure out how can we actually better include some of these views in this work in the implementation, is going to be quite important.
And then, yeah, you mentioned, so most of my work is spent on thinking about the labor market impacts of AI. And so, there's lots of interesting things that I'm working on there. One of them is, we didn't get to talk about this much today, but one of them is, you know, we have all these measures of what share of jobs in the economy are going to be impacted by these increasingly capable systems, for example, and some of them have quite high, you know, estimates.
For example, you know, in a paper I wrote recently, early this year we did this analysis and we kind of found that about 80 percent of workers in the U.S. have at least 10 percent of their tasks, their work tasks kind of primed to be impacted by these systems in some way. It doesn't always have to be due to automation, but there's gonna be some disruption and change and productivity, you know, impact from the systems.
I think I'm interested in digging a lot more deeper into the implications of that. So one thing in particular is recognizing that, like the impacts of this technology on a worker, and the impacts of this productivity gain and potential disruption will be quite different across different types of workers, right? And there's lots of data that we can get about these, kind of, characteristics of workers that might make them more vulnerable to harms from disruption, as opposed to more likely to benefit or kind of be okay through a transition to more capable systems.
So, trying to investigate some of that more by looking at, okay, well, we know that older workers, for example, when they experience a technology shock and experience disruption to their work and maybe need to gain new skills, we know that's especially costly for older workers than it is for younger workers who are kind of just starting out. And so where is this overlap between this characteristic and potential for disruption from AI?
The same is true for workers that have, kind of, like, lower levels of savings. You know, where, if you're a policymaker trying to think, how can I help to make sure that this transition is smooth for workers? You can transition to new work. Some of the most vulnerable people there would be folks who just don't have the savings and the ability to smooth a shock to their income through a transition.
And so how can we better clarify, like, this is the site, the set of workers that are both most exposed to this technology, while also being having these characteristics that make them quite vulnerable to, you know, these impacts if they were to have to find a new job or to kind of learn new skills and make a transition.
Kevin Frazier: Well, count me among those who are keen to stay abreast of the next great report from Sam Manning, but unfortunately we'll have to leave it there and good researching to you, sir.
Sam Manning: All right. Thanks, Kevin.
Kevin Frazier: The Lawfare Podcast is produced in cooperation with the Brookings Institution. You can get ad-free versions of this and other Lawfare podcasts by becoming a Lawfare material supporter through our website, lawfaremedia.org/support. You'll also get access to special events and other content available only to our supporters.
Please rate and review us wherever you get your podcasts. Look out for our other podcasts including Rational Security, Chatter, Allies, and the Aftermath, our latest Lawfare Presents podcast series on the government's response to January 6th. Check out our written work at lawfaremedia.org. The podcast is edited by Jen Patja, and your audio engineer this episode was Goat Rodeo. Our theme song is from Alibi Music. As always, thank you for listening.