Lawfare Daily: Tim Fist and Arnab Datta on the Race to Build AI Infrastructure in America
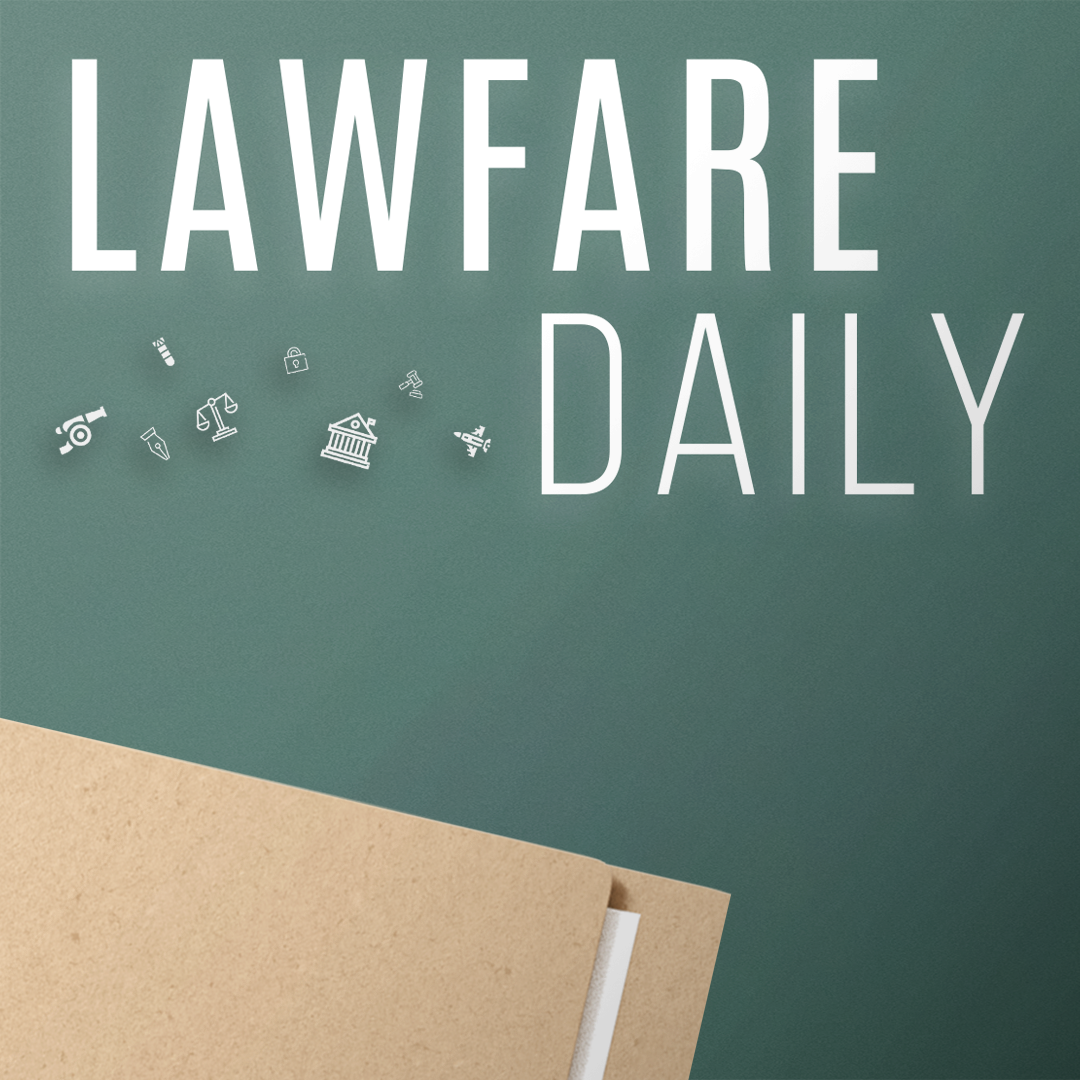
Published by The Lawfare Institute
in Cooperation With
Tim Fist, Director of Emerging Technology Policy at the Institute for Future Progress, and Arnab Datta, Director of Infrastructure Policy at IFP and Managing Director of Policy Implementation at Employ America, join Kevin Frazier, a Contributing Editor at Lawfare and adjunct professor at Delaware Law, to dive into the weeds of their thorough report on building America’s AI infrastructure. The duo extensively studied the gulf between the stated goals of America’s AI leaders and the practical hurdles to realizing those ambitious aims.
Check out the entire report series here: Compute in America.
To receive ad-free podcasts, become a Lawfare Material Supporter at www.patreon.com/lawfare. You can also support Lawfare by making a one-time donation at https://givebutter.com/lawfare-institute.