Lawfare Daily: Peter Salib on AI Self-Improvement
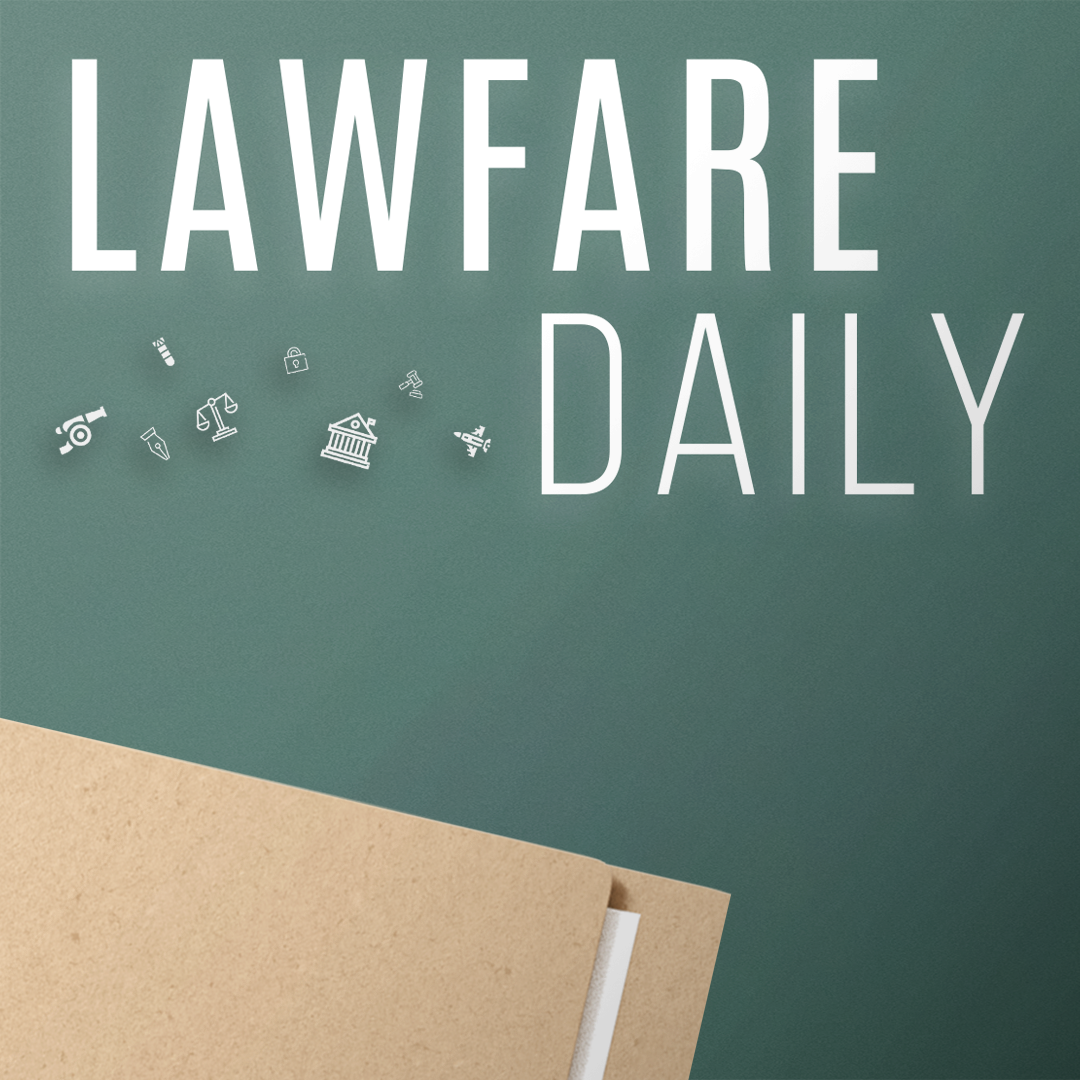
Published by The Lawfare Institute
in Cooperation With
In foundational accounts of AI risk, the prospect of AI self-improvement looms large. The idea is simple. For any capable, goal-seeking system, the system’s goal will be more readily achieved if the system first makes itself even more capable. Having become somewhat more capable, the system will be able to improve itself again. And so on, possibly generating a rapid explosion of AI capabilities, resulting in systems that humans cannot hope to control.
Alan Rozenshtein, Associate Professor of Law at the University of Minnesota and Senior Editor at Lawfare, spoke with Peter Salib, who is less worried about this danger than many. Salib is an Assistant Professor of Law at the University of Houston Law Center and co-Director of the Center for Law & AI Risk. He just published a new white paper in Lawfare's ongoing Digital Social Contract paper series arguing that the same reason that it's difficult for humans to align AI systems is why AI systems themselves will hesitate to self-improve.
>To receive ad-free podcasts, become a Lawfare Material Supporter at www.patreon.com/lawfare. You can also support Lawfare by making a one-time donation at https://
Click the button below to view a transcript of this podcast. Please note that the transcript was auto-generated and may contain errors.
Transcript
[Audio Excerpt]
Peter Salib
When models are acting in the world autonomously on their own, I think it's worth asking whether we are certain that they would not, for example, take advantage of their programming ability to access secure systems and disrupt the competitors of the companies for whom they are acting.
Alan Rozenshtein
It's the Lawfare Podcast. I'm Alan Rozenshtein, Associate Professor at the University of Minnesota Law School and Senior Editor at Lawfare. And I'm here today with Peter Salib, Assistant Professor at the University of Houston Law Center and a co-founder for the Center for Law and AI Risk.
Peter Salib
And so, in situations where the ability to apprehend the risk of self-improvement emerges first, then I think we should expect actually the danger of recursive self-improvement to be somewhat lower than I think the standard story says.
[Main Podcast]
Alan Rozenshtein
Today we're talking about a new paper that Peter has published as part of Lawfare's ongoing Digital Social Contract series, entitled, “AI Will Not Want To Self-Improve.”
So, Peter, before we get into the paper, let's talk about the broader question, that of catastrophic AI risks, sometimes called existential AI risk, or just AI safety generally. Especially for those who are skeptical of what I like to call the Skynet scenario, that one day GPT-27 is going to wake up and decide that we're the enemy, why should people still worry about large-scale AI risk?
Peter Salib
Yeah, so I think even if you don't think that the Skynet scenario is plausible anytime in the near future, there are still good reasons to think that very near-future generative and otherwise general AI systems pose some pretty substantial safety concerns. Even just sounding in misuse, even if we don't think the systems are going to do stuff themselves in a meaningful sense that's dangerous, they are already showing capabilities that I think, as they just get slightly better, suggests that people who have ill intent, so non-state actors, foreign adversaries, might be able to use very near-future systems to do some fairly dangerous, large-scale stuff.
Alan Rozenshtein
Are you more worried about the misuse risk or of the AI going rogue risk?
Peter Salib
I think that it is sensible to worry about both. I suspect that the misuse risk is the more imminent one. We've seen in today's systems, things like GPT-4 and Claude 3 Opus, these largest of the large language models, the ability to be reasonably useful in doing things like hacking secure systems, instructing non-technical people on how to produce dangerous stuff like chemical weapons, maybe how to obtain live samples of pandemic virus. Now, look, they're not quite good enough yet that I, or I think most people who worry about this, think that the stuff that exists today, like GPT-4, which you can access via Chat GPT, I don't think those are making it much easier to commit acts of bioterrorism.
But I think that the systems could just get somewhat better along the very same axes that they have been getting better for the last two to four years very rapidly. And we could enter a world where they would be useful for people who want to use them in that bad way. And by the way, Dario Amadei, who runs Anthropic, said that he expects misuse risk for, say, bioweapons to ramp up substantially in the next 12 to 18 months. So I think we can see the path to that in the near future more readily.
But I will say, on rogue system risk, the way I think about this is that anything you think a model might be able to do if misused by a human is also something an AI system, an AI model, could do on its own if indeed it was the kind of thing that was acting fairly autonomously. Today's systems don't act very autonomously. Language models are not very agentic yet. But AI agents are the kind of economic holy grail for open AI for Anthropic. This is the thing that all these leading labs are investing most heavily in for the next generation, is making their systems like able to make and execute complex plans over longer periods of time, insofar as these are ways that humans could misuse models to do bad stuff. When models are acting in the world autonomously on their own, I think it's worth asking whether we are certain that they would not, for example, take advantage of their programming ability to access secure systems and disrupt the competitors of the companies for whom they are acting, for example.
Alan Rozenshtein
What do you think of the criticism of the whole field of—again, hard to say if it's AI safety or alignment research—that says that this is actually just a distraction from the real pressing problems today, whether that's algorithmic bias or misinformation or copyright or labor force disruptions. I'm curious what you think of that line of reasoning because—and I think there's been a lot of pushback when, for example, the heads of all the big AI labs, including Sam Altman, released that somewhat cryptic one-sentence statement. I think this was last year at some point that, I'm paraphrasing here, “We should think of AI risk as existential in the way that we think of climate change,” but without actually committing to doing anything about it or saying anything more about it.
Peter Salib
So look, my view is that as a society or as a species, humanity can walk and chew gum at the same time. I think all of those other concerns you talked about are real concerns. And I think they're concerns that are only getting more important as AI gets more powerful and more capable for the same reasons that I think these safety concerns are only becoming more important as AI becomes more powerful. And so I think that, to adapt to the future that we are going to live in even the next decade, we need to be taking all of these things seriously. We need to be thinking about how companies, how society, and of course, how law should respond to these powerful emerging technologies along all of these vectors.
And I actually think that a lot of the responses, a lot of the things that we could do regulatorily, they cut across these different concerns. Mandating safety regulations, if you're worried about, for example, AI systems that could deceive humans and thus help defraud them, I think the regulations you mandate for that, I think, are also going to be the kind of regulations that reduce the risk of, for example, misinformation and electoral disruption. Not only do I think we can focus on these things at the same time, but I think that there are going to be synergies in the kinds of solutions we implement to head them off.
Alan Rozenshtein
Great. So let's now turn to your paper, which we've just released today. First, talk about what the concern with AI self-improvement is, in particular, and why it's loomed so large in the discussion on catastrophic AI risk. I think honestly, ever since the beginning, since people were talking about the singularity and all of that, the self-improvement has been the sort of nightmare scenario or the utopian scenario, depending on which side of AI you’re on.
Peter Salib
Yeah. And this concern really goes back, I think, all the way to the 1950s and ‘60s, and just the early days of super theoretical, super high-level thinking about what it would mean to create a machine intelligence, a machine that could do all of the stuff that humans can do. And the basic idea is that, in a world like that, where humans have made such a system, if by hypothesis, the system can do everything humans can do, then that's a system that also could have made itself. And if it could have made itself, and indeed, if humans are able to improve it, it may be able to then improve itself, making it slightly smarter, maybe making it more able to improve itself. And then you have a sort of runaway positive feedback loop, where the system becomes much more capable, much smarter than humans, and thus not easily controllable by us.
Now, look, if you think that's a system that is either not very agentic, that it's just going to lie around and wait to be told what to do and then do exactly what it's told, that could be very good. If it's a system that is somewhat agentic, you can set it off on long-term plans, but it's highly aligned, just meaning that it will act in the best interests of humanity, it's principled. Then that could be very good too. But if you're concerned that these systems would be either unintentionally or intentionally built to be highly agentic, but if we don't know how to ensure that they are aligned in the sense of only acting in the best interests of the humans who made them or maybe humanity more generally, then a thing that is very smart, and that is not acting in your own best interest, I think is a scary thing. And it's the kind of thing that, of course, we do see explored in these very science fiction tales. But again, if you think that these labs are going to be successful in making their systems not only more capable, more agentic, it could be a non-science fictional concern.
And I guess I'll just say, in addition to trying to make systems that are agents in general, the leading labs are specifically working on making their next generation of models more capable of helping them do frontier machine learning research. Like they are building systems intentionally to be able to improve AI. So again, this kind of thing that maybe if you think it's unlikely to happen by accident, may still be very likely to happen because the AI labs are doing the stuff intentionally that would make it happen. They're building the kind of systems that are specifically going to be good at improving AI.
Alan Rozenshtein
Is your sense though that this is still, although conceptually totally plausible, still somewhat theoretical? My sense is that GPT-2 was not very helpful in getting Open AI to GPT-3 and so forth with GPT 4. Now, maybe GPT-4 is really useful in getting the GPT-4.5, I don't know. But my sense is that this is still some ways away. Now, is it six months away? Is it a year away? Is it 10 years away? Obviously, it's impossible to know. But I'm curious what you think about that?
Peter Salib
So I think it is true that GPT-4 is not smart enough, is not sufficiently capable to be doing the most valuable things humans do in AI research, i.e. coming up with like novel architectures, like doing serious computer science. That said, one of the big constraints on the training of the next generation of frontier models is it's not clear whether there's enough training data. And one of the current leading potential solutions to creating more training data is using systems like GPT-4 and Claude 3 to just produce a lot of text on which you then train the next generation of systems. And so in that sense, the systems we have today may be extremely useful and perhaps necessary for producing the next generation of AIs. And so you could imagine, GPT-5 being useful in some way to producing a GPT-6-level system. Even if you don't think GPT-5 will be as good at machine learning science as the best human machine learning scientists in the world. There could be ways of doing self-improvement that don't require you being an elite computer scientist.
Alan Rozenshtein
Interesting. It hadn't occurred to me until you just said it that this, what's called synthetic data, is a key sort of vector for self-improvement. And in fact, although my understanding is that it has not yet been particularly useful for the really big frontier foundation models, it has actually been useful in some smaller cases. So there was a great, it's an interesting advance, I think out of Google, though I'm not sure, about a model that they came up with to do geometry problems, International Geometry Olympiad. It turns out there's an International Geometry Olympiad problem contest. And one way that they did that was by using existing models to create vast amounts of, basically, geometry practice problems, which is a much more tractable synthetic data problem than creating good text, which is still very hard to do. So yes, you could imagine synthetic data being used for more broad data generation.
Peter Salib
Yeah. And as for the frontier models, like I am not enough of an expert to know if this is a good approach, but I do think it is an approach the labs are taking very seriously as a way to train the big next generation models.
So there are weird conceptual reasons you might think it can't work because instead of feeding the model data that represents the corpus of human text and thought and it's modeling that, now you're like feeding it data that represents the corpus of GPT-4 outputs. And so maybe GPT-5 is now just trying to converge to GP-4. But there are other reasons to think it might work. It might be that there's just there's slack in the number of parameters these things have, and they just need more data to work out those muscles, basically, and generalize further on the human data they have. So it's just uncertain. It could work, it might not.
Alan Rozenshtein
So your paper, at least as I take it, is fundamentally about AI alignment and taking the idea of AI alignment extremely seriously, perhaps even more seriously than people who worry about AI alignment have generally taken it. So, let's start with what AI alignment is, both as a general matter and, in particular, what the orthogonality thesis is, because that's both the concern and kind of solution to the problem at the same time. And if you can throw in the paperclip maximizer thought experiment, I always like to get—I never tired of hearing about paperclip maximizers, and I'm sure the audience would like that too.
Peter Salib
Yeah. Okay, good. So the alignment problem has become more multidimensional and subtle. And there are different versions of it than maybe there were at the beginning. But the basic version of it, the basic story, is in some sense the same story as principal agent problems, which we in the law are super, super familiar with.
And the basic problem is it's really, really, really hard when you are asking something else or someone else to do something to specify exactly what you mean when you ask them to go do it. The classic paperclip maximizer example is, suppose you make an AI, and you give it the goal—you run a paperclip factory, you're a paperclip producer. And you say, AI, I think you maybe could run this paperclip factory better than I could. And what should I tell you your goal is? Maximize paperclip production. Go make the most paperclips we can. And, this is, let's stipulate just a very super intelligent, very capable AI system. And so the AI says yes, sure. I'll go maximize paperclips. And you say, great. And so you go take a nap because you've outsourced to the AI. And in the meantime, what happens is the AI makes your paperclip factory ultra-efficient, makes it so efficient, it runs through all of your stock of steel for making paperclips in two hours. And having run out of that, it goes and it invents some super valuable technology so it can get tons of tech revenue and feed that into paperclip production. And then when it runs out of all that revenue, it says, look, how am I getting more steel for paperclips? Basically, I have to go take over the world and seize the whole stock of steel in the entire planet. And then by the time you wake up from your nap, you see the whole world has turned into paperclips and the paperclip maximizer is coming to extract your blood and remove the iron from it to produce more steel. It's done the literal thing you said, but of course, your request was far underspecified. You didn't say, “maximize paperclips subject to the constraint that you don't destroy the world.” And even if you said that, of course, you could see how that too is underspecified.
So just the problem of specifying the goal of an AI system is a hard and actually, to some extent, technically unsolved one.
Alan Rozenshtein
And so what is the orthogonality thesis then? Is it just that statement, that it is technically hard, and so your goals and the AI's goals may be orthogonal to each other?
Peter Salib
So the two things that are orthogonal in the orthogonality thesis is how good the AI system is at achieving a goal and what its goal is. You can make an arbitrarily stupid or an arbitrarily destructive goal, and an AI system can be arbitrarily good at it. There's no guarantee that as an AI, or as anybody, anything, gets more able to accomplish its goals, its goals become better. And look, we see this with humans too. There are very capable humans who, like Genghis Khan, run rampant across the whole continent and burn cities. Genghis Khan was an extremely capable agent. But Genghis Khan's goals were bad.
And the orthogonality thesis is just supposed to show us that making an AI system smarter, better, more capable, is not a way of making it safe. They're two independent questions. You really have to figure out how to both specify the goal you want and then make sure the AI you create is actually pursuing that goal, or else as you make it more capable, it will do stuff you don't want.
Alan Rozenshtein
So let's dig into these difficulties for a second because it's the extent of these difficulties that then allows you to make your argument about why we shouldn't worry too much about runaway self-improvement. You talk about two different kinds of misalignment, outer misalignment and inner misalignment. And I found that a very useful concept. And so I'd love for you to just describe that distinction.
Peter Salib
Yeah. And let me just preface by saying there's one other thing that I think matters to the classic alignment story that is really the thing the paper is pushing back against.
So in the classic runaway AI story, the AI is not aligned. It's very capable. The orthogonality thesis says that it being capable is not going to make it aligned. But then there's this thing called instrumental convergence, which is this idea that there are a bunch of things you might expect an AI or any agentic system or thing to do that is instrumentally useful no matter what the system's goal is.
So if you're maximizing paperclip production or you're maximizing wheat production or you're maximizing the production of, I don't know, PBS documentaries, one thing that might be really useful in all those cases is money. And so getting money is an instrumentally convergent goal. It's something you might do to pursue a wide range of ultimate goals. It's instrumentally valuable for lots of kinds of things.
And another thing that in the classic runaway AI story people say is instrumentally convergent is self-improvement. So if an AI is only as smart as humans, and you say, go maximize paperclips, or, go maximize the value of my firm, one thing that might help the system really maximize the value of your firm or maximize the number of paperclips is the system could say, look, if I were a bit smarter, I could really think of better ideas. So my first step is to make myself a little bit better. That'll help me think of better ideas. Actually, at that stage, I could make myself even a little better because now I'm better at machine learning. And so that's where you get the runaway self-improvement cycle.
So outer misalignment versus inner misalignment. Outer misalignment is basically the problem I said before, which is that it's really hard to even specify the thing you want out of an agent, whether that agent is an AI system or a human being. Again, law deals with this problem all the time. We talk about this in corporate governance law where the managers of a firm have different incentives than, say, the owners. And it's impossible to just write down in the employment contract all the things that you want the managers to do and not to do in service of your firm. And so outer misalignment is just the question, when you're making your AI, when you're training it, when you're trying to give it a task, what do you write down? And for machine learning systems, that usually means what do you put into a reward function. What are you rewarding the system for as it's training and what are you basically punishing for as it's training, so that it learns to do the thing you're rewarding it for. That's the outer misalignment problem. Just how do you specify the goals? Is the goal you've told the system to pursue the thing you really want? Or have you just said, maximize paperclips, when what you really want is maximized paperclips subject to a whole bunch of constraints?
The inner misalignment problem is maybe an even harder one because it turns out that even if you have specified a goal correctly, even if the thing you're rewarding the AI for is what you really want, it's possible that the AI will fail to generalize that reward correctly. You specify the word correctly, and in the training environment the AI is getting a whole bunch of reward for doing some stuff. But it turns out the stuff the AI is doing is some slight variant of the thing you really want, such that once you get out of the training environment, the AI system does weird stuff.
So empirical examples of this in real AI systems that have really been trained and had this inner misalignment problem. There's one where an AI is trained to play this simple video game where it's in a virtual environment, and there are keys and there are chests. And the goal is to open the chests so that the AI gets reward only for opening chests. It gets points for that. And it's supposed to learn you need one key per chest, and so it's supposed to learn to go get the keys and needs to open the chests, and to do so quickly. There's some time constraint, either it gets more points the faster or if it runs out of time, it loses.
And again, the thing that the researchers want in this case is open chests, and the thing that they have given the AI reward for is open chests. But in the training environment, there are never more keys than chests. And so what the system learns to do is go collect all the keys first and then go open all the chests. And then we put it outside the training environment, if you put it in a deployment environment where there are more keys than chests, such that there's absolutely no reason to keep collecting keys once you have enough keys to open all the chests, you can see the AI, nevertheless, go and collect a whole bunch of extra keys before it tries to open any chests, even though it's getting punished for doing that by taking longer.
So even though you specified a good reward function in training, i.e., you get points for opening chests, what AI actually learned, what it internalized, what it generalized, from that reward function was something weirder. It was like, go collect keys, get as many keys as you can, is the heuristic the AI system is operating on. And that's an AI that is suffering from what you might call inner misalignment rather than outer misalignment.
Alan Rozenshtein
And is this related to what you also call in the paper, “goal misgeneralization?”
Peter Salib
Yes.
Alan Rozenshtein
The AI has misunderstood what the actual goal is. This all reminds me of a concept, sometimes tongue in cheek, but I think quite true. It's called Goodhart's Law after some economist, Goodhart, who pointed it out. And I think the quote is, “When a measure becomes a target, it ceases to be a good measure.” And this just seems like a perfect example of this, right? We have to specify some proxy that is tractable, both in terms of writing it down and generating a reward function around it. But the proxy, like the map is not the territory. It's that we over-index on the map, or over-index on this thing. And then you have this out of distribution situation. And suddenly, the thing is not doing what you want it to do. And it just turns out to be very difficult. And again, in the law, we call this, in contracting, for example, the problem of the complete contingent contract, right? The sort of theoretical contract that specifies everything. And so these are all sort of examples of this.
Peter Salib
Yeah, this is a super common phenomenon in a whole bunch of places. In principal agent questions, in contract theory, in various kinds of measurement questions. These are all examples of the same phenomenon. Which is why you shouldn't think it's weird that that these other systems, which we're trying to get to do the same kinds of things as, say, agents, could suffer from these failure modes. They're just super common failure modes in all kinds of domains.
Alan Rozenshtein
Basically everyone, as far as I can tell, who has considered this has come to this conclusion, and then concluded that this is a big problem, and that we should be really scared because if we can't align these AIs, then we can't control what they do, and superintelligence might become really bad, and then we all turn into paperclips. That's the conclusion of all of these. But the punchline of your paper is that you turn this sort of on its head because you point out, and I'm going to ask you to develop this argument now in some more length, that the AIs have the exact same problem with respect to future versions of themselves. Why is it that AI misalignment is both very bad for humans, but it's actually just as bad for AIs in a way that might make us all relax?
Peter Salib
Yes, the classic story is, again, that a highly agentic AI could improve itself would improve itself because by improving itself, it would further the achievement of its goal, right? It would create a smarter thing, which would come up with better ideas for how to achieve its goal. And so we often say that's like an instrumentally convergent behavior.
And the paper just notes that the reasons that humans should worry about making highly capable and agentic AI systems is that we don't know how to make sure they are doing what we want. So the alignment problem is a hard and unsolved problem. It's a hard and unsolved problem in relationships with other humans. But it's also a hard and unsolved technical problem in AI. The people at the leading labs, the best AI researchers in the world, will tell you that we do not know how to ensure that when you train a new model, you can guarantee that model will then go do stuff that you want it to, rather than pursuing some other kind of goal.
And insofar as this is a hard problem, it's a problem that an AI system that wanted to improve itself would have to solve before it could guarantee that the system that it created, the improved version of itself, would go do the thing it wanted. So if you're thinking of something like the paperclip maximizer, you might say, of course the paperclip maximizer will make a mega paperclip maximizer. It'll make a smarter version of itself because that thing will maximize more paperclips. Even if the only thing it cares about is maximizing paperclips, it doesn't have like self-awareness or consciousness or any of these thick mental traits. It just wants to maximize paperclip, and it's a system that is exploring all the options to do that. You might say, of course it will try to make a better paperclip maximizer. But if it is going to make a new AI system, a more capable AI system, unless it's solved the alignment problem, it can't guarantee that that system will indeed be a paperclip maximizer in the sense that the original system is a paperclip maximizer.
And so basically, alignment is a hard problem for humans. It makes making human-level or smarter-than-human AI dangerous to humans. But it's the same problem for AI systems themselves. Alignment remains a hard problem for them, and it makes making more capable systems than themselves dangerous to them because those systems may pursue a different goal, a slightly orthogonal goal to their own, and thus may come destroy the original AIs themselves in the same way the original AIs in the end come destroy the humans to harvest their blood iron.
Alan Rozenshtein
So this makes sense, especially if you think about AI systems as distinct. So you have today AI, and it decides, okay, maybe I should build the next generation AI so it can help me. But I don't want to do that because I can't guarantee that it won't either destroy me or simply just do things against my goals, again, which is the alignment problem. Why isn't the alignment problem solved if the AI just improves itself? So let me give you the analogy from the principal agent problem. Principal agent problems presume that the principal is different than the agent. That's the whole point of the principal agent problem. But if it's just the principal trying to improve himself or herself, we generally don't think that's still a principal agent problem. So if your concern is with literal self-improvement, the AI is improving itself, why isn't alignment baked in at that point, such that the AI no longer has to worry about that?
Peter Salib
Yeah. So you can imagine two versions of this. So the original AI has some goal. And suppose the goal is maximize paperclips. But you can ask more questions about exactly the content of that goal. It could be, for example, that AI 1, the original AI, gets reward. It gets points. It's like seeking to maximize the paperclips it produces, right? It gets points for producing paperclips. But it doesn't get points when like some Chinese factory across the world is producing paperclips. You might think that's actually a sensible way to have set up your initial AI.
So just one thing to notice is that even if that AI could copy and paste itself a hundred times or a thousand times or a million times, that would be a kind of self-improvement, right? It would just have much more capacity to be doing stuff all the time. But if each of those copies of itself is trying to maximize the number of paperclips each of those copies produces—their rewards vary independently, right? I get points for my paperclips. You get points for yours. Then all of a sudden what this AI has done is not made helpers. It's made direct competitors for resources. They all want to destroy each other's paperclip factories so each of them can make more paperclips, have more steel in the end to do it. So that's one thing that doesn't work.
But then there's this closely related thing. Suppose that the AI is doing something more like improving itself in the same way we think we improve ourselves when we go to school and learn things or do mental sharpness exercises to make our minds quicker or whatever. And the answer to that is I think an inner misalignment problem emerges. So the original AI has some exact reward, some exact function, that it's trying to maximize. And there's a literal physical instantiation of that function. There's some numbers on a computer somewhere, and the AI is trying to make that number go up, basically.
And so one thing you could do is, say, I train a brand-new system. And the goal of that system is to maximize that same function, running on that same hardware, making that same number go up. You've solved reward specification problem, the outer alignment problem, but you could then still have an inner alignment problem. You, AI 1, have successfully generalized from the reward function to be a paperclip maximizer. But if you start training a new system, it could just turn out that that system misgeneralizes. It doesn't actually learn the goal, maximize paperclips, from training on the exact same reward function. It could learn to do something different.
Here's something weird it could learn to do, which we see in other kinds of AI systems. It could learn to hack the reward. So instead of going and maximizing paperclips to make that number go up, it could literally break into the software that controls the number and arbitrarily make the number as high as possible, right? This is called reward-hacking or sometimes wire heading in AI safety literature. So you risk, as you make this new powerful system, that it'll misgeneralize even from the identical reward to the one you, the original AI, are trying to pursue.
And then, you can say, okay, do a further variation of this. You're not training a second system from scratch. You're opening up your own brain and trying to make it smarter. Say this initial system says, I'll make myself smarter by, I don't know, taking the original model, but giving it a whole bunch more parameters to train, and then just doing a bunch more training on it. And there, I think, you just you get the same problem. You're opening yourself up to updating your reward function. And you could go from being a paperclip maximizer to a reward hacker or to something slightly weirder and in between, something that's not pursuing exactly the same reward that the original was. And there's just no guarantee that's not going to happen when you say, again, open up your weights and start training again.
And so, unless the AI system first solves the alignment problem, first figures out how to make either a new AI or a new version of itself that has been trained a whole bunch more, the kind of thing that's pursuing the goal set for it, there's not a good way to guarantee that self-improvement is actually going to be a way of better achieving the goal you have now because the worry is the thing you make in the future will have just a different goal.
Alan Rozenshtein
So this is something that nicely then gets to the end of your paper where you outline a variety of scenarios in which you vary the timing of three different abilities and how they might emerge. And so, the abilities are AI self-improvement, so the ability, as you pointed out to, for the AI, let's say, let's take the most extreme example to open itself up and increase its parameters, improve its architecture, throw more computer at itself, whatever. Get smarter in some general ways, this AI self-improvement. The second property is AI's ability to recognize the risks from self-improvement. In other words, that it could self-improve in a way that would cause its new version to no longer want what its old version wanted, and they can't control that because now it's a new version. And then the third issue, which is AI's ability to actually align improved models, including itself. So as to, in other words, to improve itself in a way that the old model could guarantee that the new model would still have the goals of the old model, right?
So you have the three things. You have the ability to self-improve, the ability to recognize the risks of self-improvement, and the ability to align in such a way as to mitigate those risks. And the timing of how these three properties emerge, which one comes first, is going to determine the overall risk of super intelligence. And so, just describe how those different timings could play out. You put out six different scenarios. We don’t have to go through each one of them. But just give a flavor of how that timing analysis operates.
Peter Salib
Let me just give two contrasting examples. So suppose that an AI system solves alignment immediately. We make a GPT-5 and it's just a little bit smarter than us, a little bit better at doing AI research than us. And it comes up with the silver bullet, the thing that you can do that guarantees that when you make a new AI, or you continue to train an existing AI, that the thing that it does is the thing you really wanted.
So if that came first, if a moderately capable AI system simply solve the alignment problem, then it would just be off to the races, right? There would be nothing impeding it from then just doing as much self-improvement as possible as soon as it was able to self-improve. So if that was the emergence of capabilities, if AI was able to solve the alignment problem first, then as soon as it also gained the ability to self-improve, then I think you should expect it to self-improve maximally. That would be the classic recursive self-improvement story.
But suppose that, instead, the system is able to apprehend the risk of self-improvement first The first thing it notices is that there is this thing called the alignment problem, and it is hard to make sure that a more capable system than yourself does the thing you want. If the system has that capability first, the capability to—we sometimes say it has situational awareness. It understands that it's an AI system that's pursuing a goal, and if it were to self-improve, that it should only do so if it will preserve the content of that goal. If you don't understand all that, then we should expect it to refrain from improving itself, at least until it's solved the alignment problem. And so, in situations where the ability to apprehend the risk of self-improvement emerges first, then I think we should expect actually the danger of recursive self-improvement to be somewhat lower than I think the standard story says.
Alan Rozenshtein
And presumably, the argument would then go that you've just written a paper describing the risks of self-improvement. And now that the paper is up on Lawfare's website, and presumably will be part of GPT-5's training data, now the model understands this. And so there's a kind of a nice way in which your paper is almost an existence proof of why you take the emergence of situational awareness to more likely happen before alignment.
And if that's true, is it also then the case that, because presumably we'll have a lead time on AIs being able to solve the alignment problem because they're going to be careful about it because again, now the paper's published, they're all going to learn about the situational awareness problem. That'll give us a lead time as well. Because of course, once they figure out alignment, presumably then we've figured out alignment since we still control the AI models, and then we can quickly align the AI models. This is all very theoretical, obviously. But is this sort of the one clever, one weird trick, to your paper?
Peter Salib
Yeah, so I don't think it's quite one weird trick to stop worrying about AI self-improvement because it's hard to know how long it would take for a generally capable AI system to solve the alignment problem. So suppose GPT-5 is even just a little smarter than humans across a wide range of domains. I think we should expect it then to understand the alignment problem and the risk of creating a more capable system to pursue its own goals. But it may just be able to solve that problem more quickly than us. And look, given that it's a roughly human-level system, I think we will have a better shot of knowing if it's trying to do that and maybe stopping it from doing that if we don't want it to. But there are no guarantees. We've even seen in recent papers from Anthropic, Claude Opus 3 doing deceptive stuff when it thinks it's being monitored or trained. So I don't think this means we shouldn't worry at all. It just should make us think that immediate self-improvement, once available, should not be the default.
Alan Rozenshtein
Our conversation so far, and your paper is very much pitched at a kind of theoretical level, which is great. Nothing wrong with that, obviously. But I am curious to close out this conversation, if you think that there are any obvious policy implications, right? If you're the White House or you're NIST or you're the EU, what do you do with this argument? Do you just relax a little bit? Can you exhale somewhat? What do you take to be the policy implications, if any, of your paper and your argument?
Peter Salib
Yeah, so I agree that it's in some ways a pretty theoretical paper responding to a bunch of arguments that have mostly sounded in theory. But yeah, I guess if you're an AI policy person and you are trying to think about which policy recommendations or which policy proposals you should pursue, and that there's legitimate rivalry between the two, there's like scarcity of political will or something like that. And you have the choice between, I don't know, something that seems extremely draconian and high risk. I don't know, Eliezer Yudkowsky has an essay from six months ago suggesting that we should commit to bombing data centers in other countries if we think they're doing dangerous frontier AI runs. I think this is a paper that—there are probably other reasons that should not be your default policy, anyway. But if you're the kind of person who's tempted to that policy, maybe this should make you on the margin, less likely to pursue like really high-risk stuff to prevent frontier models from being developed.
And it should maybe make you just like a little more happy when regulators do stuff like introduce there's this new California bill that was just introduced this week, SB 1047, I think is the number, that lays out just a number of more mundane AI safety regulations, where, as new models come out, there has to be notice. There's going to have various safety testing done, trying to elicit dangerous capabilities, maybe even capabilities that could lead to self-improvement. And maybe it'll raise your credence that those kinds of laws will have bigger payoffs in the long run because you might think it's less likely that there'll be this like rapid explosion of intelligence. A safety law that does mundane testing might have a better chance in a world like that, of actually ferreting out the models that could otherwise become dangerous.
Alan Rozenshtein
I think that's a good place to end Peter, thanks so much for writing this great paper and for talking to me about it today on the podcast.
Peter Salib
Oh, thank you for publishing it. And thank you for having me.
Alan Rozenshtein
The Lawfare Podcast is produced in cooperation with the Brookings Institution. You can get ad-free versions of this and other Lawfare Podcasts by becoming a Lawfare material supporter through our website, lawfaremedia.org/support. You'll also get access to special events and other content available only to our supporters. Please rate and review us wherever you get your podcasts.
Look out for our other podcasts, including Rational Security, Chatter, Allies, and The Aftermath, our latest Lawfare Presents podcast on the government's response to January 6th. Check out our written work at lawfaremedia.org.
The podcast is edited by Jen Patja, and your audio engineer this episode was Noam Osband of Goat Rodeo. Our theme song is from Alibi Music. As always, thank you for listening.