Let’s Bet on the Next Big Policy Crisis—No, Really
Prediction markets and other geopolitical forecasting methods are the stuff of science, finance, and statistics, not fantasy. Yet decision-makers in the U.S. government, except for the intelligence community, have yet to embrace experimentation with these types of markets.
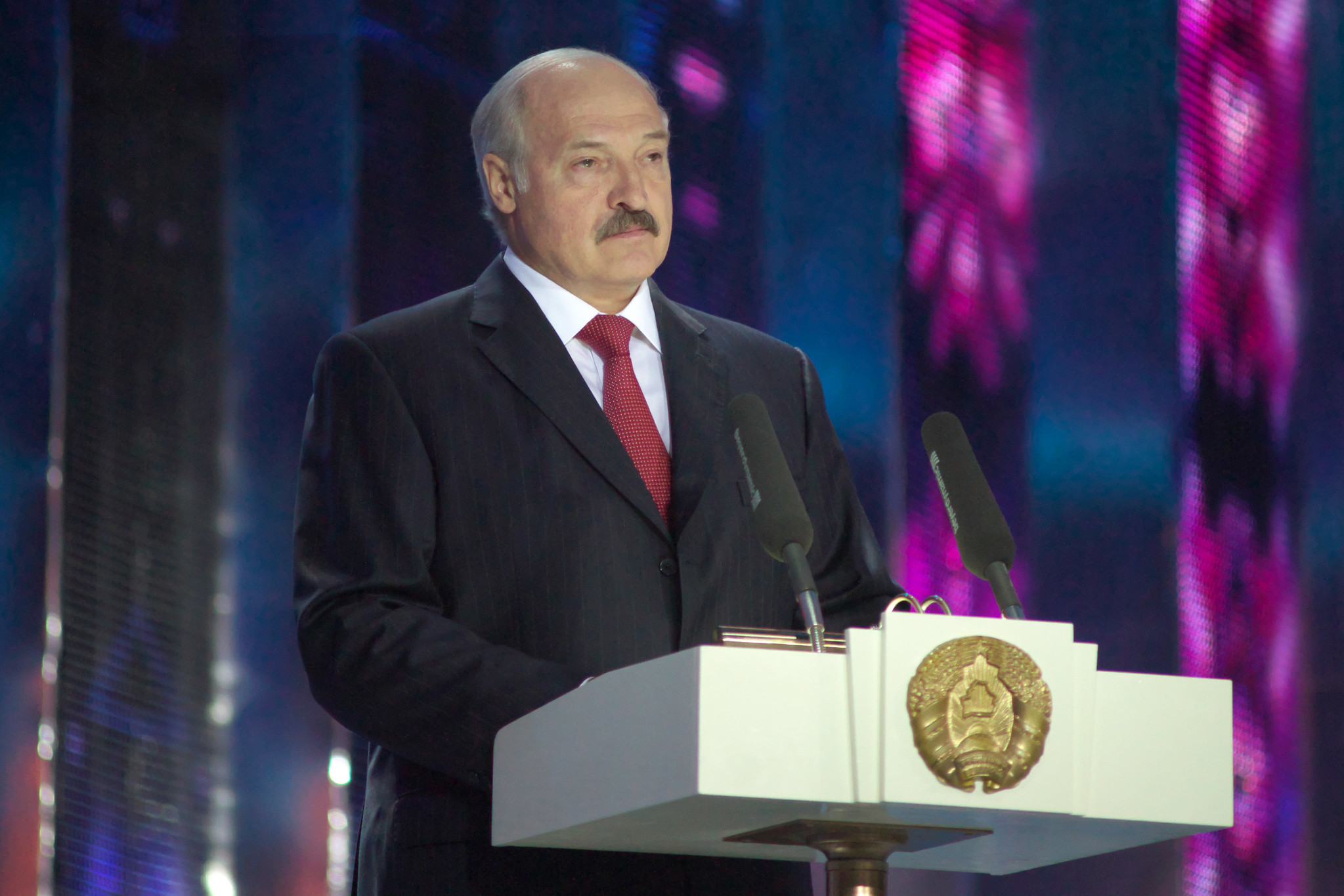
Published by The Lawfare Institute
in Cooperation With
Will Belarusian President Alexander Lukashenko—“Europe’s last dictator,” who ordered the hijacking of a plane to apprehend a dissident journalist—still be in power in 2024?
Nobody knows, of course. But it’s a question that the U.S. government definitely wants the answer to. Lukashenko’s regime is infamously personalistic; Belarus would likely see several changes if he were no longer in charge.
But imagine if Lukashenko’s odds of remaining in the presidency were ascertainable. Would government policymakers plan differently? Would CEOs change investment strategies in eastern Europe? Would military strategists watch the Belarusian-Russian border more warily?
Sure, this situation is entirely hypothetical. But it turns out that the idea of predicting developments in foreign policy is not as far-fetched as it may seem.
Nearly two decades ago, a handful of innovators at the Department of Defense’s research arm, the Defense Advanced Research Projects Agency (DARPA), wanted to see if they could more accurately forecast future geopolitical trends. They could do what the U.S. government had always done to predict overseas developments—throw money at researchers to study a given region, rely on open-source analysis or even deploy covert operators on the ground. But if there was a better, cheaper way to forecast the future, they wanted to find it.
What emerged from this effort was a “futures market” for geopolitical events: a Policy Analysis Market (PAM) designed to help DARPA forecast military and political instability in several countries in the Middle East by aggregating expert knowledge.
It was a fascinating, edgy experiment—and so it immediately flamed out. Two senators got wind of the PAM and blasted it in a press conference, casting it as a “federal betting parlor on atrocities and terrorism.” The program was promptly killed and John Poindexter, the office’s then-leader, suddenly found himself retired.
It was a cautionary tale—one that essentially destroyed the idea of governments using prediction markets as a tool to try to better forecast aspects of the future.
Except, that’s not at all what happened.
Today, if you ask most people around Washington, D.C., about the saga, a handful have heard of the DARPA project. What is less well known is that the prediction market ecosystem is alive and well—and perhaps more popular now than ever before. These markets are deployed by companies, universities, “crypto bros” and, yes, even some parts of government. They’re designed by commercial companies, public interest groups, intelligence agencies and private investors. And they have the potential to become a powerful, useful new tool for policymakers—if they are willing to accept both the rewards and the risks that come with them.
What is a prediction market?
In a prediction market, participants place bets on what they believe the outcome of a particular event will be within a given timeframe.
It may be easier to understand a prediction market when compared to more traditional financial markets. A trader on Wall Street who forecasts that Tesla will rise in value over time should purchase the stock, or futures in the stock, anticipating a profit.
The difference with a prediction market is that instead of buying up a stock that will increase by an unspecified amount—as in the stock market—participants are instead betting that a specified event will occur before a specified end date. Participants then purchase and sell contracts based on their estimates of the likelihood of future events—such as which horse will win the Kentucky Derby or which candidate will win in a political primary. As time goes on, an efficient prediction market will see the price of a contract correlate with the likelihood of a particular outcome.
Let’s say that someone wants to bet that Lukashenko will still hold the Belarusian presidency on Jan. 1, 2024. And let’s say in this market that an individual bet or “contract” is worth a dollar. If a contract is currently trading for less than a dollar, a correct prediction will yield a profit. So at, say $0.75, investors would want to procure additional contracts based on the expectation that they’ll get back $1 for every $0.75 put in—if they’re revealed to have been correct on Jan. 1, 2024.
Of course, everyone wants to win. If it seems likely that Lukashenko will retain control, more people will bet on that outcome. And as more people do so, the closer the purchase price will get to $1. (The price in prediction markets adjust automatically, in contrast to traditional sports betting where an odds-maker sets a trading price.) Conversely, if someone believes the conventional wisdom is wrong—and that Lukashenko will for whatever reason not hold the office of the presidency in 2024—they have reason to purchase contracts accordingly.
This explanation of a prediction market glosses over much of the specifics, but, fundamentally, the market relies on the idea that by repeatedly soliciting the opinions of many incentivized players who are rewarded for being accurate and penalized for being inaccurate, the market will accurately reflect the likelihood of a particular outcome, and also reflect how that likelihood changes over time. It is, in effect, a way to crowd-source expertise through an efficient market mechanism.
What could a prediction market mean for decision-makers?
Asking if one may predict whether Lukashenko will maintain his grip on power is an interesting question in its own right. But it’s the possibility of putting that information to practical use that has policymakers and intelligence analysts alike fascinated.
What might U.S. decision-makers do differently if they knew Lukashenko would be out of office in 2024? Arguably, a post-Lukashenko Belarus—whose people are already souring on closer ties with the Kremlin—might turn away from Russia and toward the West. Though speculative, to be sure, the expectation might lead the European Union to prepare to pursue closer integration with a new Belarus or, conversely, to prepare for the eventuality that Russian President Vladimir Putin might seek to mass troops on the border if there was worry that Lukashenko might fall—sparking a regional conflict.
Policymakers interested in Lukashenko’s odds of remaining in power wouldn’t have to rely exclusively on the formal intelligence process: They could launch a public prediction market and allow people across the world (including those in Belarus, Russia and nearby states) to bet on what will happen. Then decision-makers could take advantage of better information to plot out potential courses of action for the United States and its allies to take—saving time, material and political capital in case the United States needed to respond quickly.
Admittedly, that’s a lot of unknowns. But this is where the market comes in: As 2024 grows closer, and interested parties ask this question of a responsive prediction market, they’re likely to see the market reflect the likelihood that Lukashenko will still be in power. And if the answer seems like a no, it’s more than reasonable for policymakers to accelerate the development of plans for a world without him.
There are policymaking applications in the domestic and private sectors too. Interested parties could have discovered warning signs of significant lethargy among disengaged youth in the 2016 election if they had questioned beforehand what percentage of the U.S. eligible voting public was planning to vote. Cybersecurity industry leaders could ask whether the U.S. government will enact a mandatory breach notification law for major cyber incidents before Dec. 31, 2022—and, if it seems likely, shift strategies appropriately. Similarly, the insurance industry could take advantage of better information to price risk more accurately. If, say, the industry had a better way of predicting the rate of future incidents of catastrophic infrastructure failures, it could adjust its actuarial tables accordingly.
In other words, the value to intelligence analysts, policymakers and industry CEOs alike lies in knowing the likelihood that a certain event will happen so that they can deploy resources, shape policy or invest appropriately.
What happened to geopolitical prediction markets after the DARPA fiasco?
Congressional uproar notwithstanding, there’s a robust market for prediction markets—even geopolitical ones—in the United States today. In fact, over the past several years, these markets have expanded wildly in their forms, accessibility and reach.
Today, there is significant demand for internal corporate prediction markets and crowd-forecasting. Google, Ford, Yahoo, Hewlett-Packard, Eli Lilly and a number of other prominent corporations have operated or continue to operate a corporate market. Some of their questions may delve into geopolitics, but in most cases employees bet on subjects such as whether deadlines will be met, what products will take off and what earnings statements will be.
Prediction markets have become more accessible in recent years, as various companies offer prediction-markets-as-a-service to people or companies without the capacity, resources or expertise to create the markets themselves. Cultivate Labs and Good Judgment are two of the most well-known companies that have designed and operated markets for clients ranging from private corporations and universities to the British government. (Note: Some of the newer geopolitical forecasting offerings aren’t technically “markets” at all—and instead use nonmarket methods to aggregate expert opinions, sometimes with excellent results.)
Unlike internal prediction markets, public prediction markets are legally more complicated—most types of public betting on politics or policy are a dicey legal proposition in the United States. The political prediction markets that do exist and that enable U.S.-based users to bet small amounts of real money—such as PredictIt and the long-running Iowa Electronic Markets—operate under “no-action” letters issued by the Commodity Futures Trading Commission (CFTC), given their academic bent and research goals. More recently, Georgetown University built out its own crowd-forecasting platform—which is not strictly a prediction market but rather a way of surveying and pooling expert opinions—specifically for geopolitical futures. Similarly, Metaculus offers a platform for a quasi-prediction market, in which the currency of exchange is prestige points, and anyone can submit a question for inclusion in the market.
The development of blockchain technology has led to other opportunities for prediction markets. Today, there are a handful of what creators are pitching as “decentralized” public prediction markets backed by cryptocurrency. Examples include Augur, Gnosis and Polymarkets. (Note: We make no claims as to whether decentralized prediction markets that trade in cryptocurrency are legal for U.S.-based users.)
And it may be that prediction markets are poised to enter a new era of popularity. Just this past week, a prediction market that operates as a true financial exchange opened its digital doors. Kalshi—a San Francisco-based startup currently operating in beta—is the first fully regulated (CFTC-approved) prediction market. Because Kalshi is regulated, more significant amounts of money can be wagered than in many other markets, enabling them to build out a new asset class of events futures. The implications for this are obvious: An asset class like this could serve as an alternative or a supplement to more traditional insurance, allowing companies and individuals to hedge against crop failures, cyberattacks or floods.
Finally, of course, there is the U.S. intelligence community. Far from backing down following the DARPA snafu, the various scientific and intelligence agencies seem to simply have become more cautious with their rhetoric while launching several futures-modeling programs that are—or that incorporate elements of—prediction markets. For example, in 2010, the intelligence community started a prediction market for top-secret-cleared government employees on its classified networks. From 2011 to 2015, the Intelligence Advanced Research Projects Activity (IARPA)—the intelligence-minded sister of DARPA—ran the Aggregative Contingent Estimation (ACE). ACE was a project designed to “dramatically enhance the accuracy, precision, and timeliness of intelligence forecasts … [by means of] techniques that elicit, weight, and combine the judgments of many intelligence analysts.” Today, IARPA still runs the Hybrid Forecasting Competition, which “develop[s] and test[s] hybrid geopolitical forecasting systems.” And DARPA didn’t miss out either—though it seems to have pivoted to more quantitative, computer-based models.
Are prediction markets accurate?
While prediction markets and other methods of geopolitical crowd forecasting are growing in popularity, the question naturally turns to whether these advancements can translate into potential predictive power.
The answer is complicated. In short: sometimes.
Proponents are adamant that these markets are more accurate than many other methods of future forecasting, such as polling—a snapshot of a random sample at any one moment in time—or soliciting expertise—a notoriously unreliable method of predicting just about anything. But there is limited empirical, scholarly evidence to say how effective these markets are; when they are likely to work; when they are likely to mispredict; and which types of market mechanism or other crowd-forecasting methods work best.
And anecdotal evidence is mixed. Prediction markets had recent success by predicting the results of the 2020 U.S. presidential election more accurately than polls. But they also infamously forecasted a high likelihood of finding weapons of mass destruction (WMDs) in Iraq in 2003 and incorrectly predicted the appointment of Chief Justice John Roberts. These markets didn’t see Brexit coming—or the election of Donald Trump in 2016. The same information that suggests that an experimental prediction market was more accurate than intelligence reports alone also warns decision-makers: not so fast.
But the value of prediction markets should not be discounted. There is reason to think that, as a theoretical model, prediction markets are sound. They share many of the same characteristics as other financial markets for which researchers have much more robust evidence. And to be sure, even more well-developed markets are imperfect—the efficient capital market has its critics—but they generally operate more effectively than most nonmarket models. As markets become increasingly popular, more information is expected to become available—though, even now, there are several excellent books on the subject.
People are interested in these markets because early results are promising. The fact that interested parties are still learning—and that reputable experts and engineers differ on how settled the theory of accurate prediction markets is—should indicate the need for increased research and dissemination of new results. (While there are at least dozens of large, active prediction markets today, there is limited public awareness of these markets and most of the data and learnings from the markets seem relatively self-contained.)
In short, in the messy business of forecasting the future, prediction markets are not a bad way to go about things. As they mature, more data may prove that point empirically—or not.
Why the hesitancy to embrace the market?
It’s a compelling promise: that a financial or otherwise gamified market mechanism (such as one in which people risk only prestige points or fictional money) could generate actionable information for policymakers, market analysts or intelligence officers.
So why hasn’t the United States, beyond the intelligence community, taken advantage of their applicability for policymaking? We have a few theories, augmented by the ideas of several researchers.
The first is moral hazard—the same factor that killed one of the early DARPA markets. There is an incentive for someone to bet on a contract and then create a real-world effect that results in a profitable outcome: Imagine an assassin bets on the death of a prominent politician and then makes it happen. At a less dramatic level, there’s still the problem of allowing people to profit from others’ misfortune—though, of course, this is not unique to markets.
The second is the opportunity for policy manipulation. If a market were used as a direct tool to shape policymaking, those with a stake in the outcome would be incentivized to direct the market toward that outcome. Think about foreign intelligence operatives wanting to derail U.S. policy, companies affected by new regulations, or even U.S. decision-makers seeking “public” support for their policies. Moral hazard and manipulation can to some extent be mitigated by market structures and scope, but they are undoubtedly among the thorniest challenges of applying these markets to policymaking.
There are likely other reasons to explain the lack of widespread use of prediction markets by policymakers, such as the legal uncertainty—or outright illegality—of betting on politics, sports and events in the United States; the experimental nature of prediction markets; and the U.S. government’s well-documented struggle to incorporate innovate research initiatives into existing processes and functions.
A final reason may be, as some observers have suggested, entrenched expectations and elite bias—high-ranking people in government and corporations do not or cannot accept that democratized decision-making may be superior to their judgment.
So, what’s next?
Along with our colleagues at the R Street Institute, we have undertaken a broader project assessing how to improve our ability to measure cybersecurity. As part of that project, we speculated that prediction markets might be one novel and creative way of creating data about cybersecurity and cyber policy. We expected to find few viable examples of comparable markets in existence.
Instead we learned of a thriving new industry poised to take off—one that, if policymakers in Washington, D.C., are willing to take a chance on it, might enhance good decision-making in the modern era.
In our next post, we intend to examine in more detail our initial premise: how a prediction market for cybersecurity might fare. For now, however, we observe only that these types of markets are in much wider use than is generally known, and that the U.S. government—except for the intelligence community—is among the only types of decision-makers who have yet to embrace experimentation with them. That needs to change.
The Delphic Oracle was famed throughout the Ancient World. Nostradamus is still remembered today for his alleged capabilities. Predicting the future is a powerful, seductive pull on the mind: Everyone wants to know what is next—not least, those who make policy for nations. But prediction markets and other geopolitical forecasting methods are the stuff of science, finance, and statistics, not fantasy. And as much as we are still learning about how—and when—they work best, we are convinced that they will one day have a notable place in the tool kit of government and military decision-makers. One that might warn U.S. policymakers of imminent disruption in Belarus. One that just might tell us much more about our own country. That prospect both excites and terrifies. And yet it may lie just ahead.