Licensing Frontier AI Development: Legal Considerations and Best Practices
AI self-regulation may not be enough. It’s time to consider licensing regimes that apply throughout the AI life cycle.
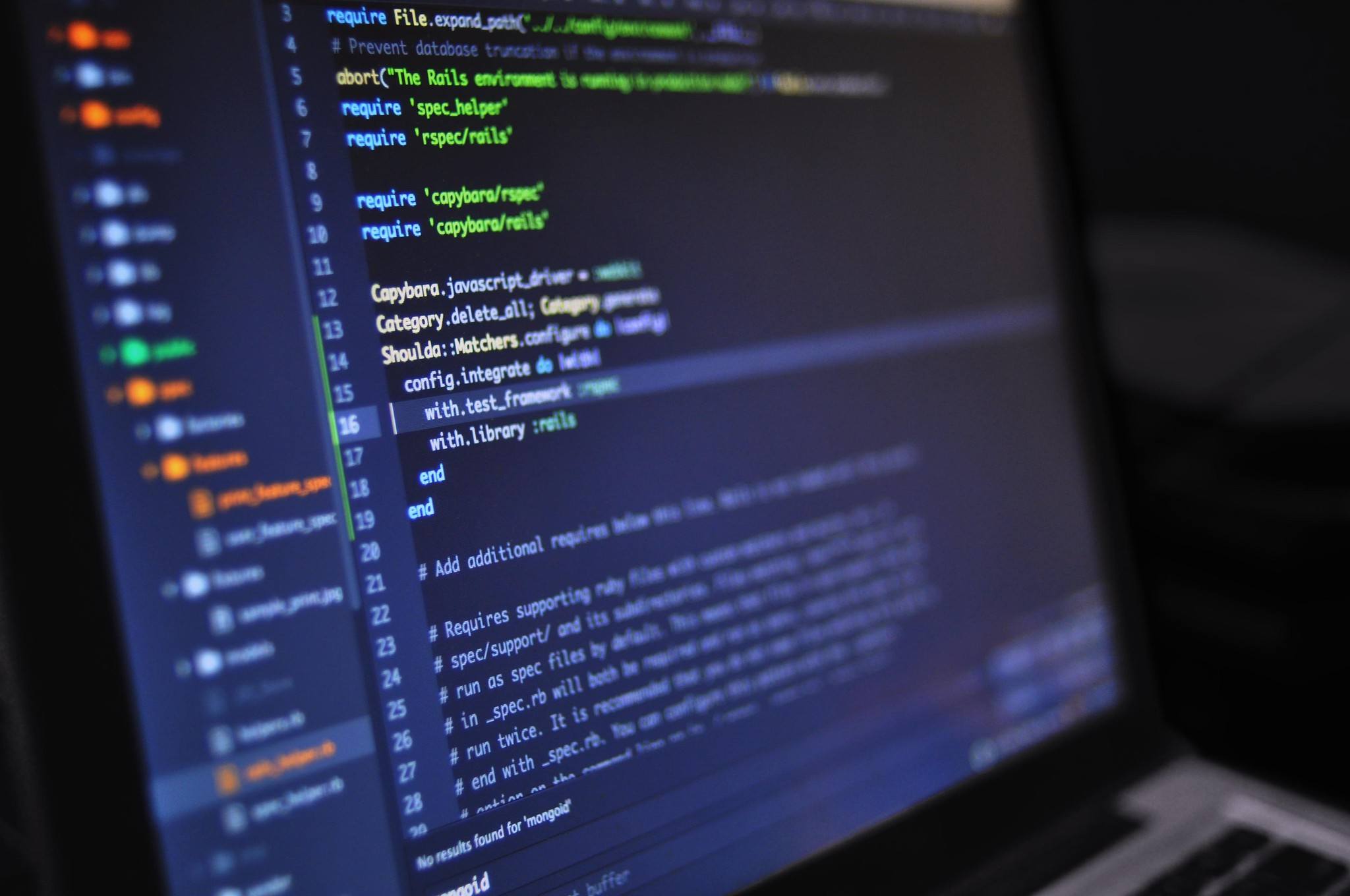
Published by The Lawfare Institute
in Cooperation With
Just before Thanksgiving, OpenAI was thrown into chaos when it abruptly fired CEO Sam Altman, triggering a multiday power struggle that resulted in Altman’s return and the removal of five of the board’s six members. As others have argued recently in Lawfare, the chaos at OpenAI demonstrates the limits of industry self-regulation. Comprehensive and effective regulation of artificial intelligence (AI) will require government action.
Congress and the Biden administration have already taken some steps to respond to the promise and peril presented by AI. In September 2023, the Senate kicked off a series of “AI Insight” forums designed to inform members about the risks, implications, and regulatory possibilities for AI. President Biden signed a sweeping AI executive order in October directing the establishment of new regulatory measures, including red-teaming initiatives, reporting requirements for next-generation models and computing clusters, and studies on risks at the intersection of AI and biotechnology. Regulatory proposals are rolling out across the world. China is leading the pack with continued rulemaking on generative AI, and the EU is working through the final stages of negotiating its forthcoming AI Act.
Many in the United States and around the world have called for regulation of the most advanced and capable AI systems, also referred to as “frontier AI,” to promote their safety and security. Sens. Richard Blumenthal (D-Conn.) and Josh Hawley (R-Mo.) recently put forward a bipartisan framework for AI regulation that advocates for an AI licensing regime for advanced AI models. Experts agree that licensing could be appropriate for frontier models as well. A licensing system would require frontier AI developers and other parties that support frontier AI development to register with a government body, which would in turn require them to follow certain practices while training and deploying their models—as opposed to regulating the models after they are already deployed. This support for licensing regimes reflects worries that the harms of certain advanced AI could be severe, and that a regime ensuring frontier AI is developed in a safe manner might be more appropriate than a regime that relies on a model of regulation that would apply only after harms have already occurred, such as liability.
Licensing of AI models and their developers may be an effective option for frontier AI regulation. However, the impact and efficacy of an AI licensing regime will depend heavily on the details, from the regulation’s (or regulations’) scope and specific requirements to its resourcing and implementation. Regulatory loopholes, underspecification of regulatory requirements, or poor implementation that creates an ineffective or technically incompetent agency or misguided authorities for an existing agency could leave critical gaps and fail to address extant and emerging national security threats. At the same time, sprawling and excessively complex regulatory burdens or overbroad restrictions could lock in the dominance of a handful of powerful companies or unnecessarily halt progress, damaging America’s global AI position and, therefore, its broader technological position.
To properly strike this balance, policymakers crafting an AI licensing regime would need to carefully consider what rules the regime would apply to each major component of the frontier AI lifecycle, from the large computing cluster needed for training through to model deployment. Any effective regime must also include an empowered regulatory body that has the resources and expertise to carefully monitor this rapidly emerging technology.
Which Parts of Frontier AI Can Be Licensed?
The process of developing a powerful frontier AI system has three major stages: hardware acquisition and setup, model training, and model deployment. Policymakers can implement licensing requirements governing each of these three stages.
Creating a powerful AI system begins with the concentration of advanced hardware in large computing clusters of specialized AI hardware. These sites typically include thousands of leading AI chips. While some AI companies own their hardware, many rent access to hardware or form partnerships with companies like Microsoft, Google, or Amazon that offer access to leading-edge computing power through the cloud.
Once an AI developer has secured access to sufficient hardware for frontier AI development, the second major stage is AI training. When a developer first “boots up” an AI system in a cluster, it is initialized with completely random values and possesses no intelligent capabilities whatsoever. The AI system learns to accomplish complex cognitive tasks by churning through massive amounts of training data. By the time training is complete, the AI system evolves from an empty structure of random numbers to a capable program that can help write software, solve math problems, and pass the bar exam.
After the model has been fully trained, the third and final stage of the frontier AI lifecycle is deployment, at which point the model is put into use for its intended purpose. The model can be made available to end users through websites or interfaces that enable access to AI-based applications. Other AI systems, such as Meta’s Llama models, are open sourced and uploaded in full to proliferate across the internet, after which point anyone can download or modify them.
There are many, many different possibilities for the number of licenses, and the nature of licenses, that could be required under a particular regime. An AI licensing system could, for example, require that a single license be granted before frontier AI development even begins and impose requirements throughout the training and deployment process to maintain that license. Alternatively, different circumstances might require separate licenses with more stage-specific terms—perhaps with AI hardware owners requiring a different license from AI developers and deployers. Licenses could also be structured such that the license would be required only to deploy the model, but the regime may impose retroactive requirements on how an AI system was trained—and how safely and securely it was trained—in order to receive that deployment license.
The close collaboration between Microsoft and OpenAI provides an example of how a licensing regime for each stage in the frontier AI lifecycle might function in practice. Microsoft, which provides the computing hardware on which ChatGPT is trained, could be required to have a license for its high-performance data centers that would approve the training of models in those locations. OpenAI would then apply for a development license to train and develop a frontier model in one of Microsoft’s approved data centers. Finally, once the model was trained, OpenAI would apply for a license to deploy it for use by customers. This would allow regulators to attach best practices for safe and secure AI development, several of which we cover in more detail below, at each stage in the frontier AI lifecycle.
Crucially, focusing regulation only on the deployment stage may not cover the risks that could be generated while the AI system is still being trained. For example, a computing cluster with significant security vulnerabilities could train a frontier AI system that is then readily stolen by competing countries. Imposing requirements at strategic points across all three stages of the lifecycle in one form or another will be critical to ensure that frontier AI development is responsible, safe, and secure.
What License Requirements Could Make Frontier AI Development Safer?
Regardless of the precise form of license structure, certain categories of license requirements will be important elements of any licensing system. One such category is cyber and information security policies. As frontier models become increasingly capable—and the associated security threats become increasingly daunting—it will be crucial to ensure that such powerful systems cannot be stolen by state adversaries or non-state hackers or leaked by internal bad actors. AI licensing requirements could bake cybersecurity into each of the stages of the frontier AI development process—for example, by requiring that frontier AI models are developed in secure facilities using only secure hardware. Developers could also have to agree to abide by prespecified best practices for information security throughout the training process, including model access controls, insider threat programs, and hardware-level protections for the model weights.
A second license requirement category that will be an important component of any effective AI licensing regime is the evaluation and red-teaming of the frontier AI models during model training, before model deployment, or both. Rigorous red-teaming and assessment of powerful models has already drawn significant interest from government, industry, and academia. The commitment to these practices was apparent in the White House’s compilation of voluntary commitments, the recent AI executive order, and the Bletchley Declaration from the first global AI Safety Summit. Many frontier AI developers and their partners have started implementing preliminary evaluation frameworks, with several of these developers releasing documents describing their evaluative processes and noting safety and security concerns related to the use of their models. Additionally, several AI companies have noted that they would not proceed with the development of a frontier system if serious issues were identified over the course of safety evaluations during training.
However, the science of AI evaluation is very much in its infancy. Even leading AI developers discover safety issues and novel capabilities after their models have already been trained and deployed. Technical evaluations of the national security risks presented by AI systems are severely underdeveloped, and we still fundamentally do not understand the internal processes of how complex neural networks work. These challenges make robust assessments and red-teaming for dangerous capabilities, such as the divulgence of biological or nuclear weapons information, all the more critical to reduce the risk of catastrophic incidents on the AI frontier.
To address this need, AI licenses for development and deployment of such models should be conditioned on the verified execution of rigorous evaluations to measure the capabilities of AI systems at regular intervals throughout the training process, prior to model deployment, and through continuous testing of deployed models. This effort would require significant research and investment to pioneer new and rigorous methods to evaluate harmful AI capabilities. Licenses to deploy a frontier AI model could then be conditioned on, in addition to establishing an appropriate evaluation process, demonstrating that a model would behave in a safe manner and mitigating any issues identified during the evaluation process.
Finally, even if a model is carefully scaled and thoroughly evaluated, it is possible—in fact, it is likely—that many models will still subsequently raise new and unknown capabilities, vulnerabilities, and safety concerns. Models whose issues were not flagged during evaluation could still be at risk of generating harmful information, enabling catastrophic misuse, or even replicating autonomously once deployed if not properly monitored. Frontier AI licenses could include post-deployment requirements to implement model safeguards and monitoring to identify, report, and address such risks as they appear. In practice, this could involve monitoring the inputs and outputs of models for dangerous behavior while they are deployed.
Who Would Implement These License Regulations?
If a licensing regime is implemented, its effectiveness will rely as much on its successful implementation as on its actual terms. This raises the critical question of who should be empowered to carry out AI license regulations, and how. These questions are particularly difficult for frontier AI models. Expertise in machine learning is concentrated in industry and academia, and is lacking in the federal government—leaving a knowledge gap for regulators who would be responsible for setting up and implementing licensing rules in practice.
What are some practical options for the home of AI licensing? There is no single “clean” fit within the federal government for implementing comprehensive AI regulation, but there are several possibilities. The Department of Commerce has been significantly empowered by the AI executive order and will take the lead on implementing the new Defense Production Act AI reporting requirements, the extensive AI red-teaming efforts directed by the National Institute of Standards and Technology, the new know-your-customer directives for cloud computing, and the establishment of the new U.S. AI Safety Institute, among other regulatory roles. The Commerce Department also has institutional experience related to AI and compute stemming from its role in the development of advanced hardware and software export controls. The relevant institutional knowledge that is emerging and likely to grow within the Commerce Department, particularly concerning red-teaming AI, could be useful for both creating red-teaming and evaluation requirements and executing government-led evaluations of models. The Commerce Department’s administration of the new AI reporting requirements under the Defense Production Act could also enable it to effectively identify AI developers who might fall under any licensing regime.
Another possible home for an AI licensing body is the Department of Energy, which could call upon substantial technical expertise from its National Laboratories network and high-performance computing facilities. The Energy Department also has jurisdiction over nuclear weapon secrets and “restricted data,” which may be relevant in certain AI national security evaluations. This familiarity with high-performance computing could prove useful in regulating and licensing such facilities where they are privately owned, and experience with controlled information could ease the process of implementing red-teaming of models for nuclear secrets and potentially other forms of classified and sensitive information.
Alternatively, rather than tapping an existing department or agency, many have called for the creation of a new agency or an independent commission with the explicit focus of implementing a frontier AI regulatory and licensing regime.
Each of these options has benefits and drawbacks. Regardless of exactly where within the U.S. government a frontier AI licensing body lives, the effective implementation of AI licensing would depend on the properties of that empowered body.
One essential element will be access to technical expertise. Developing and executing AI license requirements like frontier AI red-teaming and hardware security will require in-depth knowledge of the technology. The Office of Management and Budget has already emphasized the importance of attracting such talent for the federal government, and the presence of an AI licensing regime would greatly magnify that need. This expertise can be tapped through federal contractors and public-private partnerships, but it will also ideally come through (and will eventually require) an in-house staff with demonstrated science and technology backgrounds in areas such as machine learning, cybersecurity, and computer engineering. Another key attribute of a successful licensing body will be sufficient financial and computational resources to build and experiment with the software and hardware solutions needed to enforce the requirements of the licensing regime.
Finally, when it comes to AI regulation and enforcement capacity, the most important attribute of all may be speed. The current rates of progress, investment, and excitement about the development of frontier AI are extraordinary, even relative to the general pace of Silicon Valley. To have a chance of keeping up with such a rapidly emerging technology, a regulatory body must be empowered to react quickly to unexpected developments and breakthroughs in a bold and flexible manner.
***
AI licensing will not be straightforward, but this complexity is not an insuperable barrier. Policymakers will best position themselves to craft effective licensing regimes if they carefully consider and define the regulatory structures that will best address the unique process of AI development and require rigorous evaluations of advanced models. The rapid progression of AI capabilities and its expanding role in our society will make calls for regulation louder and stronger over time—especially as national security risks from powerful AI begin to intensify. Now is the time to seriously consider how regulatory tools, like license requirements in the frontier AI lifecycle, could be used to reduce the risks from AI development while ensuring that beneficial systems are still developed and deployed safely across the United States.