Sovereign AI in a Hybrid World: National Strategies and Policy Responses
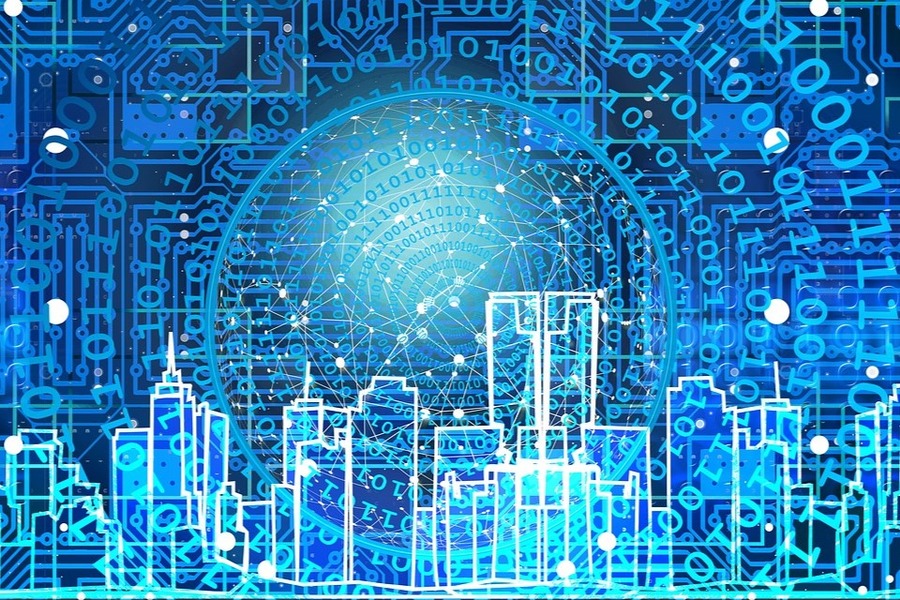
Published by The Lawfare Institute
in Cooperation With
OpenAI’s release of ChatGPT in November 2022 triggered an ongoing global race to develop and deploy generative artificial intelligence (AI) products. ChatGPT also raised concerns among governments that the sudden and wide release of access to OpenAI’s AI chatbot—and the subsequent launch of several competitive large language model (LLM) services—could significantly impact their economic development, national security, and societal fabric.
Some governments, most prominently the European Union, took quick action to address the risks of LLMs and other forms of generative AI through new regulations. Many have also responded to the emergence of the technology by investing in and building hardware and software alongside—and sometimes in competition with—private-sector entities. In addition to or instead of regulating AI to help ensure its responsible development and deployment, governments building AI are market participants, rather than regulators, working to achieve strategic goals such as promoting their nations’ economic competitiveness through the technology.
Taiwan, for example, launched a $7.4 million project in 2023 to develop and deploy a homegrown LLM called the Trustworthy AI Dialogue Engine, or TAIDE. Built by enhancing Meta’s Llama open models with Taiwanese government and media data, one of TAIDE’s goals was to counter the influence of Chinese AI chatbots—required by Chinese law to adhere to “core socialist” values—and protect Taiwan with a domestic AI alternative more aligned with Taiwanese culture and facts.
Model development, however, is just one of many government AI strategies. For example, earlier this year, the French government invested approximately $44 million to retrofit the Jean Zay supercomputer near Paris with about 1,500 new AI chips. Jean Zay, owned by the French government, had already been used by Hugging Face (an American AI company) and other organizations to train the Bloom open-source, multilanguage LLM. The upgrade is part of a broader French government strategy to develop domestic AI computing accessible to French researchers, start-ups, and companies.
These and other government efforts to develop generative AI models and build AI infrastructure are new, and their nature and scope are still not fully clear. What is emerging, however, is a picture of governments challenging the binary nature of U.S.-China competition in AI and asserting themselves in ways that could influence and potentially reshape the global AI space. How much impact governments’ AI-building efforts will have—and even, in some cases, how genuine they are—remains to be seen.
Independent of the outcomes, various national governments’ AI strategies and tactics present both challenges and opportunities for the U.S. As it seeks to maintain leadership in AI, the U.S. must navigate these dynamics while shaping international governance, enhancing economic cooperation, and supporting the success of open, democratic, and rule-based societies.
Digital Sovereignty → Sovereign AI
The Taiwanese and French cases of government support for AI are two examples of what some call “sovereign AI”: a national government’s policy of placing the development, deployment, and control of AI models, infrastructure, and data in the hands of domestic actors. The concept is an offshoot of digital sovereignty—the idea that national governments should assert control over the development and deployment of digital technology because of how fundamentally it shapes significant political, economic, military, and societal trends and outcomes.
While sovereign AI encompasses regulatory measures to protect against potential harms such as bias, privacy violations, and anti-competitive behavior, governments’ sovereign AI efforts have emphasized investing in, producing, and distributing AI technologies. These ventures underscore a point that France, Germany, and Italy made during the AI Act debate in late 2023 and that former Italian Prime Minister Mario Draghi has since echoed: AI regulation that stands alone or impedes AI innovation could severely hamper a nation’s AI success.
Various national governments have pursued two main sovereign AI strategies: developing AI models and acquiring AI computing infrastructure. Both of these direct approaches are often part of a larger, more holistic effort to create a national AI ecosystem that includes regulation, strategic investments in research and development, promoting homegrown AI companies financially and politically, and developing and attracting top domestic and international AI talent.
The direct approaches to sovereign AI are windows into the moving and blurring lines between the private and public sectors as governments deploy industrial policies to participate in the digital arena rather than leaving this space to private-sector competition. These approaches also highlight both the risks and opportunities that governments see in AI, offering insights into how the U.S. government might work with allies and partners developing sovereign AI while preventing sovereignty efforts from becoming overly protectionist.
Sovereign AI Models Emerge
One of the most high-profile ways governments have advanced sovereign AI is by supporting the development of government-aligned generative AI models. Among others, as outlined in Table 1, the governments of Japan, the Netherlands, Singapore, Spain, Sweden, Taiwan, and the United Arab Emirates (UAE) are directly catalyzing the development of sovereign AI models.
Table 1. Sovereign AI models.
Country | Model Name | Model Type | Open or Closed | Training Data | Disclosed Budget | Infrastructure Used |
Japan | Pretrained from scratch | Open | Japanese text, English text, code, and math | Undisclosed but likely significant | Fugaku supercomputer (Fujitsu’s A64FX microprocessor, an Arm-based CPU) | |
Netherlands | Pretrained from scratch (not yet released) | Intended to be open | Publicly available Dutch data | ~$15 million | SURF’s Snellius supercomputer (Nvidia A100 and H100 GPUs) | |
Singapore | V1 pretrained from scratch, V2 based on Llama 3 | Open | Southeast Asian languages | $52 million | Amazon Web Services (AWS) (Nvidia A100 GPUs) | |
Spain | Family of foundation models | Some pretrained from scratch; others based on open-source models (not yet released) | Intended to be open | Spanish and the co-official languages of Spain (for example, Basque) | Undisclosed | MareNostrum 5 (Nvidia H100 GPUs) |
Sweden | Some pretrained from scratch; others based on Llama 3 | Open | Swedish, Norwegian, Danish, Icelandic, English | Mostly undisclosed except for ~$560,000 provided by the Swedish government | Berzelius supercomputer (Nvidia A100 GPUS) | |
Taiwan | Based on Llama 2 and Llama 3 | Open | Fine-tuning with local traditional Chinese and English datasets (government and local business data) | $7.4 million | Taiwan National Center for High-Performance Computing (Nvidia H100 GPUs) | |
UAE | Pretrained from scratch | Open | Primarily English-language sources | Undisclosed but likely significant | AWS, with the most recent model trained on ~256 Nvidia H100 GPUs |
Singapore’s Southeast Asian Languages in One Network—or SEA-LION—project is an instructive example of how governments are shaping sovereign AI models and the role that these models are beginning to play in the global AI arena.
In 2023, the Singaporean government funded and launched SEA-LION with a budget of roughly $52 million. At its core, the project focuses on developing and making available AI models tailored to 11 Southeast Asian (SEA) languages, including Thai, Vietnamese, and Bahasa Indonesia.
Singapore trained the first SEA-LION LLMs (SEA-LION v1) from scratch. These models are relatively large and capable—ranging from 3 billion to 7 billion parameters (the number of parameters correlates with a model’s sophistication) and trained on 980 billion tokens (each token is roughly the equivalent of a word). In comparison, GPT-4—the most advanced public OpenAI model at the time—has approximately 1.8 trillion parameters and about 13 trillion tokens. The second set of SEA-LION LLMs (SEA-LION v2) was built on the open-source Llama 3 8 billion parameter model, which the SEA-LION team enhanced with SEA language data (continued pretraining) and trained to understand and follow instructions in these languages (instruction tuning). A third version of SEA-LION based on Google’s Gemma 2 LLM was released at the beginning of November.
Though benchmarking shows that GPT-4 performs significantly better overall than SEA-LION v1, the latter—despite its relative size—demonstrates superior performance in sentiment analysis of SEA languages (that is, the emotional tone or attitude expressed in text). Like SEA-LION v1, SEA-LION v2 performs very well against and generally exceeds the SEA language capabilities of other open-source LLMs.
Sovereignty is central to many of these government-led LLM projects. For instance, the Singaporean government initiated SEA-LION in response to a “strategic need to develop sovereign capabilities in LLMs.” Though what governments mean by “sovereignty” is sometimes unclear, a main goal of SEA-LION and the most commonly stated sovereignty objective of other sovereign LLM projects is protecting and promoting national languages, arguably threatened by the potential dominance of global LLMs trained primarily on the English language.
Sovereign AI models are also intended to protect countries’ cultures, given that societal norms, national histories, and other information that contributes to a country’s culture are embedded in language. The SEA-LION developers, for example, have expressed concern about a “West Coast American bias” in and the “woke” nature of American LLMs, suggesting misgivings about the data that trains U.S.-based LLMs as well as the guardrails placed around those models.
Though the topic of protecting culture in AI is sometimes abstract, Taiwan provides a concrete example in the context of its political culture. In 2023, Academia Sinica, Taiwan’s national academy, beta launched an LLM (unrelated to TAIDE) intended to improve how LLMs work with traditional Chinese text. When asked, “What is National Day?” the model answered, “October 1”–China’s National Day, not Taiwan’s. The model’s developers attributed this mistake and others like it to unexpected use cases and model hallucinations, and the mistakes were likely exacerbated by the use of training data from China.
With these types of concerns in mind and as shown in Table 1, many of the countries developing sovereign models are training or fine-tuning their models with datasets consisting of national languages generated in-country to align their models’ output with national cultures. For example, Sweden’s GPT-SW3 models were trained in part on data from the Litteraturbanken, a project of the Swedish Academy and others that makes freely available a significant part of the country’s literary corpus. In addition to using particular datasets, sovereign AI model developers also aim to protect culture through the process of developing models. For example, by focusing on training techniques that protect individuals’ privacy, some European developers claim they are aligning their models with “European values.”
A third stated sovereignty goal, as articulated by Japan’s Fugaku project documentation, is to produce LLMs that do not rely on foreign technology. This concern about getting cut off from foreign models—suggested as well by the SEA-LION team—is essentially the flip side of being overwhelmed by them. Australia’s national science agency highlighted this potential issue in a recent report (the Australia Foundation Models Report), stating that building sovereign AI models might be necessary if foreign models are “made too costly, inaccessible or abruptly changed in some way.”
A fourth sovereign AI goal is the advancement and support of a country’s economy. SEA-LION, for instance, aligns with Singapore’s National AI Strategy 2.0, which aims to position the country as a regional AI leader. The hub strategy is based on the idea that, by developing and releasing sovereign models, countries highlight AI projects that appeal to researchers and engineers and signal a commitment to AI that can attract investment. This approach also leads to the establishment of resources such as research centers and accelerators, further enhancing the country’s appeal as an AI hub.
As shown in Table 1, a counterintuitive commonality among the sovereign AI model projects is that they are generally open source. At a high level, one might imagine that a sovereign technology strategy would lean more toward a closed approach to maintain control over how it is built and distributed. Scratching the surface of these open-source strategies, though, demonstrates that they are potentially effective ways to bolster governments’ sovereign AI goals.
Open sourcing encourages the wide distribution of a model, which likely helps promote the languages and cultures represented by the model by making it much less costly for organizations and individuals to use. This strategy exists in the commercial world, where many AI companies have open sourced their models. For the most part, they are freely available to third parties to use and modify, making them potentially more attractive and broadly adopted than closed models.
Open sourcing could also soften the impact on a country that’s been cut off from foreign models or had its access to foreign models modified in significant and detrimental ways by providing access to substitute models. AI companies often adopt open-source strategies for precisely the same reason; they use open source to avoid closed model providers’ moves to change their models, modify their terms of use, or discontinue access to the models.
In addition, building and deploying open-source AI can grow a country’s soft power, enhancing and extending its international influence. SEA-LION, for example, explicitly invites collaboration from foreign governments, corporations, and civil society. Through this collaborative approach, countries like Singapore position themselves as AI leaders, fostering regional partnerships and expanding their global reach in AI development. Singapore is not alone in this endeavor. Spain, for instance, intends to use its new model to build cultural and commercial ties to Spanish-speaking Latin American countries. For its part, the UAE has launched a $300 million foundation to serve as a mechanism for supporting global partnerships around open-source generative AI development and the customization and adaptation of the UAE’s Falcon models across various sectors worldwide.
Sovereign AI also potentially serves as a mechanism for more countries to have a seat at the table on the international governance of AI, not as interested observers or consumers but as developers and deployers of AI with expertise in the technology and a more direct stake in governance outcomes. To date, international AI governance efforts have largely been the domain of countries—like the members of the Group of Seven—that are considered leaders or at least significant participants in the development of AI. A country developing its own sovereign AI model would demonstrate both the technical expertise and vested interest in these discussions, even if it were not traditionally part of these intergovernmental organizations’ technology governance efforts.
Despite many commonalities, sovereign AI development efforts do not necessarily fall into clear categories. Each of the examples in Table 1 varies in size, government support, goals, and other characteristics. And there are other examples of government-supported LLMs that are potentially as or more impactful. For instance, the French and German governments supported Mistral’s and Aleph Alpha’s venture financing rounds in 2023 and have provided the companies with political support to avoid falling behind in the AI race. Similarly, the Canadian government led Cohere’s most recent venture financing round.
Building Sovereign AI Infrastructure
Another way that governments are bolstering sovereign AI is by providing supercomputing infrastructure to support AI model development. For example, the recently released Modello Italia LLM—open source and trained mostly on Italian text—was developed on the Leonardo supercomputer, one of the world’s most advanced and high-performing computers. Leonardo is hosted by Cineca, a nonprofit consortium supported largely by the Italian government and funded by the EU, Italian government ministries, and other entities, including Italian academic institutions.
As with sovereign AI models, there are many examples of governments pursuing this AI compute investment strategy, including Brazil, Canada, Denmark, the EU, France, India, and Japan—each one at different stages of executing their sovereign compute strategy, as outlined in Table 2.
Acquiring and managing AI supercomputing capacity positions governments as core technology suppliers to enterprises, public-sector agencies, and other organizations seeking to develop and scale their AI models. These facilities potentially allow governments to become development platforms, perhaps replacing or at least competing with private-sector actors serving the same role.
Table 2. Sovereign AI infrastructure.
Country/Region | Name & Location | Investment Amount | Nature of Investment | Private-Sector Partners | Status |
Santos Dumont supercomputer; Petrópolis | ~$320 million | To be determined (the Brazilian government’s goal is for Santos Dumont to be among the world’s top 5 computers) | To be determined (possibly Eviden, a French supercomputer company owned by the French IT company Atos, given existing relationships) | Announced in July 2024 | |
Canadian AI Sovereign Compute Strategy; to be determined | ~$1.5 billion | To be determined | Most to be determined; one could be Nvidia | Government consultation underway | |
Gefion Supercomputer; Copenhagen | ~$100 million (15 percent from Denmark’s government & 85 percent from the Novo Nordisk Foundation, a non-governmental organization) | Nvidia DGX SuperPOD with a total of 1,528 Nvidia H100 GPUs | Eviden; Digital Realty (U.S.-based data center company); Nvidia | Operational as of October 2024 | |
EU | Existing EuroHPC AI-capable computers (nine current) and other proposed projects may apply | Up to ~$1 billion with up to an additional ~US$1 billion provided by participating EU member states | To be determined | To be determined | Calls for expression of interest underway |
France | Jean Zay Supercomputer; near Paris | ~$44 million | 1,456 Nvidia H100 GPUs, among other upgrades | Eviden | Expected completion in 2024 |
IndiaAI Compute; to be determined | $250-300 million (author’s estimate) | 10,000 GPUs | To be determined | Invitation for empanelment underway | |
Japan | ABCI 3.0 (AI Bridging Cloud Infrastructure); near Tokyo | ~$226 million | ~3,000 Nvidia H200 GPUs (author’s estimate) | HPE and Nvidia | Upgrade operational in 2024 |
Despite national governments’ recent focus on private-sector cloud providers and their central role in training and deploying AI models, government computing infrastructure projects are fairly common and have been at the foundation of supercomputing efforts since the mid-20th century. Today, according to the Top 500 list—a ranking of the world’s most powerful supercomputers—eight of the top 10 supercomputers are government owned or affiliated (Microsoft and Nvidia own the two exceptions). The U.S. government owns three, three are owned by the EU, the Swiss government supports one, and one is funded by the Japanese government. (China has reportedly stopped participating in the Top500 list to maintain the secrecy of its supercomputing advancements.)
Nevertheless, a 2023 Organization for Economic Cooperation and Development report warns that building and enhancing AI-focused computational resources has been a “blind spot” of most national AI plans—creating significant AI semiconductor ownership gaps. As demonstrated by Table 2, many countries have started to acknowledge and fill these gaps. For example, the Brazilian government recently announced that it plans to spend more than $320 million on a new AI-capable supercomputer that is intended to be among the top five supercomputers on the Top500 list.
Canada’s approach to AI infrastructure reflects this trend and suggests the many ways governments can control and provide AI computing power. Still early in its process of developing sovereign AI computing capacity, Canada appears to be deciding whether to fund an upgrade of its government-owned infrastructure (perhaps by increasing the number of the Narwal supercomputer’s AI chips), acquire and build completely new AI-capable supercomputers, or leverage existing private-sector infrastructure for AI development (generally similar to the U.S. government’s National AI Research Resource Pilot and aligned with India’s sovereign compute strategy).
Regardless of the outcome, the Canadian government’s articulated goals for its AI sovereign compute strategy have much in common with the stated goals of other governments building sovereign AI supercomputers.
Canada cites sovereignty as a primary driver of its efforts—similar to France and other countries building government-funded AI infrastructure. However, the contours of this sovereignty are not immediately clear. At its core, the concept reflects a desire to control AI infrastructure domestically, which may stem from a government’s concern that its nation will become overdependent on foreign compute providers. The motivations explicitly stated by the Canadian government center on controlling the allocation of compute capacity in the national interest.
For example, one of the Canadian government’s articulated goals is to lower barriers to AI compute resources for researchers, start-ups, and other less well-resourced organizations. This focus on expanding access represents a strategic push to build a more inclusive AI ecosystem and to place bets on innovations that could have, among other benefits, significant economic impacts and might otherwise go unfunded. With control over AI compute resources, a government can have more say in how to allocate them rather than relying on other factors farther beyond the government’s reach, including the cost and availability of private-sector compute (though both hurdles could, in theory, be addressed through regulation).
Just as important as the control-oriented component of sovereignty is Canada’s goal to promote itself as a global AI hub—a common theme among governments advancing AI. For example, in Canada’s case, the government believes it has the AI talent and companies to lead globally in AI. Nevertheless, the government assesses that Canada’s AI computing capacity lags behind other AI leaders, which puts its talent, companies, and other assets at risk by making them less productive and/or more susceptible to the pull of other AI hubs.
As is the case with governments developing sovereign AI models, very few governments building AI infrastructure mention national security, defense, or intelligence objectives in their public statements—but they likely exist in the background, given the impact of AI in each of these realms. For example, the French Armed Forces Ministry recently announced plans to build Europe’s most powerful classified supercomputer to support defense-oriented AI development and deployment, underscoring AI’s critical role in national defense and perhaps giving a sense of how other governments, though mostly silent on national security, defense, or intelligence objectives, are considering these domains in their AI development plans.
Several factors complicate the compute strategies of Canada and other countries. Among others, the costs involved in acquiring AI computing infrastructure—described below—and keeping up with the quickly evolving AI state of the art are significant. These costs may have influenced the British government’s recent announcement that it would cut approximately $1 billion from its budget to fund new AI computing infrastructure at the University of Edinburgh, a public research university based in Scotland.
Even sovereign compute projects that do go forward do not appear large enough to replace private-sector infrastructure in any meaningful way—at least for large model training, which is compute intensive. Just in the first six months of 2024, Microsoft, Amazon, Google, and Meta collectively spent more than $100 billion in AI and broader cloud infrastructure. In contrast, the project in Table 2 with the largest budget—EuroHPC—is 2 percent of that amount (roughly $2 billion divided among potentially dozens of initiatives). Similarly, France’s Jean Zay upgrade involving 1,456 Nvidia H100 GPUs is less than 6 percent of the new 25,000 “advanced” GPUs that Microsoft intends to install in its French data centers by the end of 2025. This gap may narrow as market dynamics change, but for now hardware and other costs remain a major hurdle to many governments’ sovereign AI infrastructure projects.
How Sovereign Is “Sovereign AI”?
While the concept of sovereign AI is gaining traction, achieving true sovereignty in AI remains elusive. Many of the so-called sovereign AI models and infrastructure projects rely on foreign—and especially American—technology. For example, the UAE’s Falcon model was trained on Amazon Web Services (U.S.) infrastructure, and Singapore’s SEA-LION model depends on GitHub (U.S. subsidiary of Microsoft), Hugging Face (U.S.), and soon IBM (U.S.) for distribution. Even in the context of LLMs built on open-source models, the leading provider of those models is Meta (U.S.), and many major open-source AI projects have a strong nexus in the U.S.
This dependence on foreign technology is particularly acute with respect to advanced semiconductors, which are sold primarily by Nvidia, a U.S. company—though other AI chip companies are beginning to compete in training and deploying AI. As a result, projects like the Jean Zay supercomputer AI upgrade, which emphasizes that French supercomputer provider Eviden managed the project, ultimately depend on Nvidia’s AI chips for success.
The reliance on U.S. advanced chips also highlights the potential challenges to sovereign AI posed by the evolving nature of the U.S. government’s AI technology export and foreign investment controls, as well as its increasing focus on monitoring the training and potentially limiting the export of generative AI models. These regulations are focused primarily on blocking Chinese military access to advanced AI technology and on mitigating the risks of state and non-state actors’ use of AI for harmful purposes such as designing biological weapons. However, to counter efforts to circumvent AI chip export controls, for example, the U.S. government at times has slowed and proposed restrictive rules on the export of advanced chips to countries like the UAE, seen by some as potential conduits of AI technology to China.
U.S. regulations impacting sovereign AI efforts could have a range of outcomes. On the one hand, they could result in deeper American AI partnerships with some countries (and a corresponding distancing of those countries from China’s digital technology ecosystem), as may be happening with the UAE. U.S. government restrictions could also lead to more trustworthy and rights-respecting AI development by partner countries as AI technology exports become tied to cybersecurity commitments and other safety and security guardrails deployed by partner countries. On the other hand, U.S. AI restrictions could encourage countries to exclude the U.S. from domestic AI development more than they otherwise would. Among other things, some countries might align more closely with China, which may be advancing its AI efforts despite U.S. restrictions reportedly by building its own chips, continuing to enhance its supercomputing capacity, and developing training methods that rely on less computing power.
These issues highlight that pursuing sovereign AI strategies is often more about managing dependencies than eliminating them. In reality, what most countries working toward AI sovereignty are doing is building a Jenga-like AI stack that gives them enough control and knowledge of AI technology to understand and react to changing technology, market, and geopolitical conditions but falls short of complete control akin to what India and others have over their satellite navigation systems. As the Australia Foundation Models Report observes about AI models: “Sovereign capability doesn’t necessarily mean the whole AI model is developed and managed from within Australia; it’s about our ability to manage how the model is used.”
As the Australian analysis suggests, most governments that pursue sovereign AI won’t have full control of the technology (for context, neither do the United States and China—the world’s AI leaders—though both are much closer to full control than almost any other nation). These governments may, for example, envision their sovereign model as one of several options used by organizations rather than the dominant solution. In this way, they would aim to complement, not replace, larger AI models while retaining the strategic autonomy that comes from being involved in AI development and diffusion. Similarly, sovereign AI infrastructure development will generally not outpace that of countries such as the U.S. or global cloud companies, but such infrastructure could nevertheless offer benefits such as the ability to direct compute resources to strategic initiatives.
Notwithstanding the elusiveness of true sovereignty, governments pursuing sovereign AI can potentially influence longer-term outcomes in positive and negative ways.
For example, sovereign AI could establish countries as attractive hubs for AI-focused capital and talent that can help them compete in the global AI arena. In addition, sovereign AI models could serve as high-profile examples of how to develop an LLM ethically, and sovereign AI infrastructure might support greater and more equitable resource access to AI. Furthermore, the emphasis on open-source development could promote more accessibility and innovation in AI technologies.
However, pursuing sovereign AI also presents risks. A future in which, for example, countries require the use of particular government-approved models over others (similar to existing Chinese government regulations) could lead to a super-charged form of technological fragmentation that hinders international collaboration and exacerbates geopolitical tensions, promotes censorship and propaganda, and threatens human rights, including privacy and freedom of expression.
A Hybrid Global AI Ecosystem
The current picture of sovereign AI is one of a hybrid ecosystem. Governments are investing in domestic AI capabilities, seeking to control and leverage AI models, infrastructure, and other resources to serve national interests. But at the same time, the underlying technologies and knowledge that power these AI systems—including open-source models, AI computing infrastructure, and AI research—are largely international, often interdependent, and, in some cases, under the effective control of one or a handful of third-party countries. This hybrid ecosystem reflects the complexities of modern technology development; while some nations seek autonomy, the pragmatic reality is that they also benefit from and contribute to collective advancements in AI.
What is the role of the U.S. government in this ecosystem? So far, the U.S. has taken significant steps to exclude certain countries—most prominently China—from full participation. At the same time, the U.S. has worked with allies, partners, and other stakeholders to cooperate internationally on global AI safety research and governance. For their part, U.S. companies have been leaders both in advancing U.S. AI innovation and in disseminating AI technology and know-how around the world. Collectively, the U.S. government, U.S. corporations, and other U.S. organizations, including academia, have contributed significantly to the creation and continued growth of this hybrid ecosystem.
Maintaining this collaborative approach is crucial to American interests. The strength of U.S. global leadership is deeply intertwined with its network of allies and partners in the defense, security, and economic arenas. These relationships are a core strength that comes with the responsibilities of support and partnership, which means helping to advance the technological and economic development of allies and partners—in particular, actual and aspiring open, democratic, and rule-bound countries. What’s more, though AI chip export controls and other U.S.-led restrictions have hampered China’s AI development, its companies remain viable alternatives in any vacuum left by the U.S.
Alternatively, a laissez-faire approach to sovereign AI development driven perhaps by the desire to democratize the technology could be as harmful as a lockdown policy. Developing and deploying without good governance could lead to catastrophic outcomes, and it would certainly result in missed opportunities to, among other things, cooperate internationally in AI research and development. Democratizing AI is not simply about releasing technology in the wild for anyone to use for any reason. Instead, it involves creating and supporting institutions, norms, and standards that provide the scaffolding for building and distributing AI responsibly, as the U.S. has done across three consecutive administrations. That framework, in turn, contributes to maintaining and enhancing an open, rule-bound, democratic, secure, and prosperous international digital environment, which reflects the congressionally defined U.S. international policy on cyberspace.
The most attractive approach, then, is for the U.S. government to find solid footing at the center of the hybrid AI ecosystem. It is already on its way there for two reasons.
First, the U.S. government is deeply experienced in developing and deploying AI systems through its network of supercomputers and numerous scientific, defense, development, and other AI efforts. Since 2016, the U.S. government has coupled this hands-on expertise with the development of AI governance focused on best practices, institution-building, and strong intra- and inter-government coordination, and private-sector engagement. The U.S. government has also been involved in supporting and enhancing the domestic AI infrastructure ecosystem, most recently by forming a White House task force on domestic AI compute infrastructure. As such, it stands as a capable partner to other governments aiming to develop AI in their national interest.
Second, the U.S. government has already deployed the language and policy frameworks for AI cooperation with other countries aimed at helping allies and partners meet their AI goals while also advancing American interests. For example, the State Department’s recent cyberspace and digital policy strategy sets forth digital solidarity as a viable alternative to concepts like sovereign AI. Unlike sovereignty in the digital space—introduced and still promoted by China—solidarity focuses on advancing an American foreign digital policy grounded in shared goals, building mutual capacity, and providing mutual support. The strategy, required by Congress in 2022, echoes and expands on the principles of the Clean Network, an initiative of the first Trump administration intended to protect U.S. and allied nations’ digital networks by establishing a coalition of trusted partners based on security and digital trust standards. In the sovereign AI context, this will involve steering many sovereign AI projects toward solidarity and demonstrating that doing so is mutually beneficial.
Going forward, the U.S. government will need to ensure that it continues to work with allies and partners as it attempts to mitigate the risks of international AI diffusion, enhance its benefits, and bolster U.S. leadership in AI. On their end, partners and allies will gain from working with the U.S. closely on AI development and deployment efforts and steering clear of attempts to lock out U.S. technology and other activities that prevent international AI cooperation.
To address these complex issues and foster cooperation while respecting national sovereignty, the U.S. should form an AI Cooperation Forum (AICF) to maintain solidarity among allies and partners around AI development. This forum would serve as a platform for nations to develop their own sovereign AI capabilities while sharing knowledge and best practices through regular meetings and working groups. The AICF would also support member countries in building domestic AI ecosystems by fostering collaborative AI investment and development projects and by enabling technology transfers in a manner that respects each nation’s autonomy while addressing collective security risks and other shared concerns. The AICF could also help facilitate AI cooperation and alignment with—and participation in—other multilateral organizations like the growing network of AI safety institutes.
Of course, these are not easy issues, and the assertion of unilateral control on AI can often seem like the most straightforward and effective way to head off catastrophic outcomes or achieve economic and other advantages. Nevertheless, the incoming Trump administration, with the support of Congress, will need to collaborate with other nations that see AI as a technology that’s as much theirs as it is that of the U.S. To ensure American and broader economic progress and societal security, the administration will need to pursue AI engagement with partners and allies across the diplomatic, commercial, and security spheres. Navigating these evolving dynamics will be essential to the United States’s leadership of and success in the global AI ecosystem.