The Case for Prioritizing the Creation of an AI Benchmarking Consortium
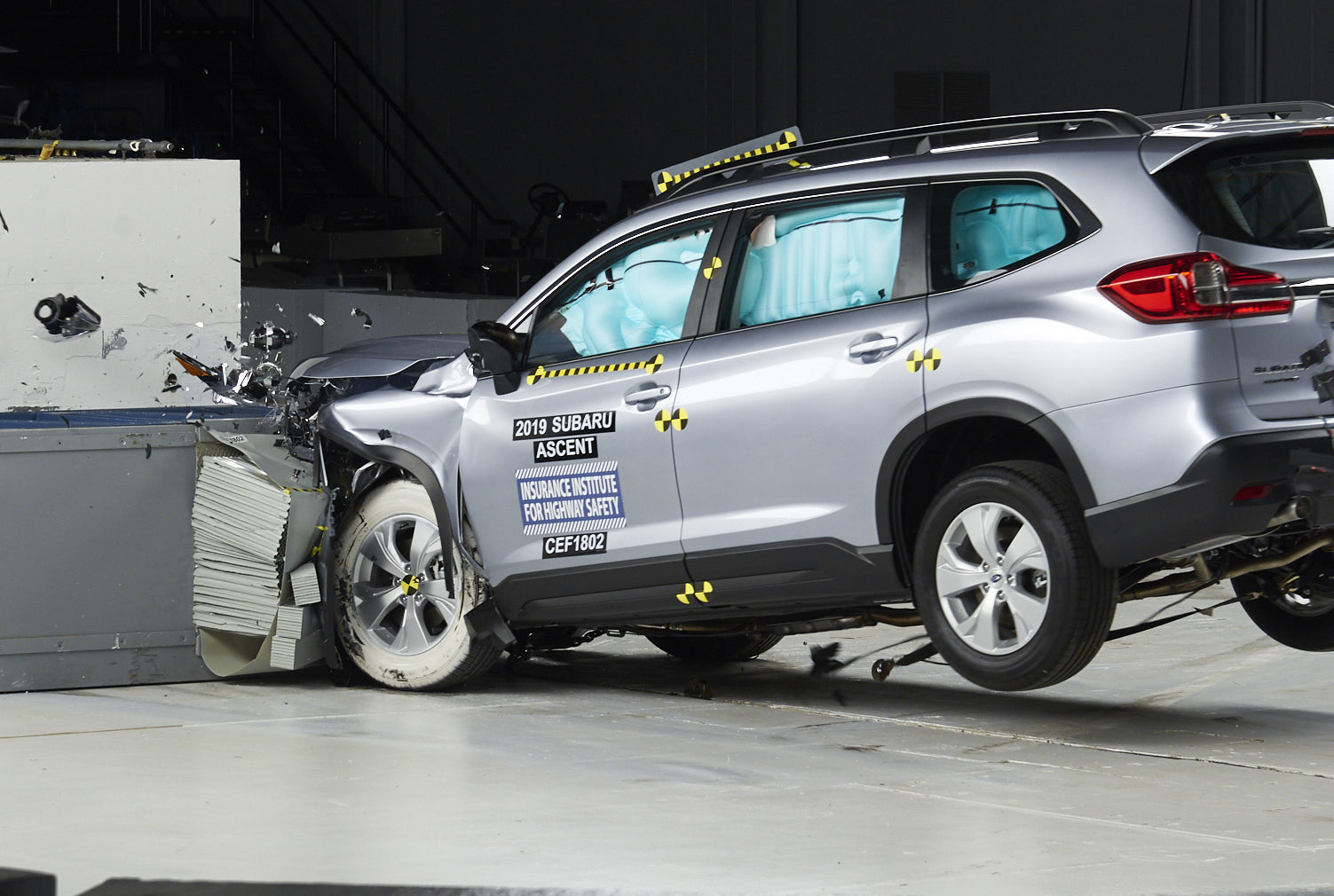
Published by The Lawfare Institute
in Cooperation With
What constituted safe driving in 1964—when there was still “nationwide confusion” over whether you could brake with your left foot—would likely get you a ticket and a few gestures from your fellow drivers if replicated today.
And what passed for a safe car in 1968—when head restraints were still an optional feature—would not pique the interests of a contemporary stunt driver.
The slow, steady, and, over the course of decades, substantial increase in traffic safety did not occur by accident (excuse the pun). That progress was the result of an alternate ecosystem in which entities pursue regulatory innovation to counter or check “traditional” innovation. Just as the private sector led the development of ever faster, sleeker, and fuel-efficient cars, a reciprocal effort to reduce the potential of those models to endanger drivers, passengers, and the public emerged. In particular, the Insurance Institute for Highway Safety (IIHS) led an effort that leveraged innovation and market forces to incentivize automakers to adopt safety features that then became the basis for federal regulation.
In short, a review of IIHS makes clear that the best defense to risks posed by emerging technologies, such as AI, is a good offense—moving fast and braking private efforts to accelerate the development and deployment of ever-advancing technology. This regulatory innovation—iterating on new ways to slow technological progress to move at the speed most likely to serve the public interest—is no easy feat.
Rapid advances in the capacity of AI models have left those concerned about short- and long-term risks of AI searching for the right regulatory response. That search has often led advocates to traditional regulatory schemes, such as the creation of a new agency tasked with monitoring and steering AI development. Any agency, though, cannot take on the full list of regulatory tasks associated with moving fast and braking AI. In particular, the efficacy of any federal regulator hinges on having accurate, actionable, and up-to-date information on the risks and harms posed by AI models. A glance at the regulatory role of IIHS in the context of auto safety makes clear that a similar entity could foster regulation innovation with respect to AI.
This article makes the case for the creation of an AI Benchmarking Consortium that has the institutional capacity to close the information gap that currently exists between AI labs and regulators by continually developing and implementing new ways to measure the capacity of AI models and monitor AI developments and societal impacts. Through study of IIHS’s testing infrastructure, technical expertise, and maintenance of its social license to operate, it’s possible to develop a framework for how best to design and launch the AI consortium.
AI Information Asymmetry
AI labs have a monopoly over the information that may aid external stakeholders in estimating the severity and likelihood of risks posed by AI models and, by extension, developing norms, standards, and regulations to reduce those risks. Consider, for example, that OpenAI released ChatGPT without sharing actionable information on the architecture, hardware, training compute, dataset construction, and training method of the model. Such omissions cannot become a practice among labs. As more AI labs develop and deploy more and increasingly sophisticated, complicated, and complex models, the information disparity between labs and potential regulators will expand and thwart the odds of timely and responsive regulation.
As summarized by the Ada Lovelace Institute, monitoring and measuring AI research, development, and deployment requires tracking the following: inputs to AI systems data, such as software, hardware, compute, knowledge, time, or energy; categorical information about the data and model of the AI systems, like the identity and location of the developer and/or deployer, the number of parameters, modalities, model architecture, cost, and so on; categorical information about processes or operations followed in development and deployment, including benchmarks used in tests (and performance on those benchmarks), impact assessments, and oversight mechanisms; direct outputs and outcomes of AI systems, such as users served, number of tokens generated, revenue generated, or number of documented accidents and misuse of the system; and externalities generated by the development and deployment of AI systems, like infringement of user and affected persons’ privacy, and skill atrophy among users.
The accurate and timely collection of information within those aforementioned categories poses several questions including but not limited to whether any entity has the capacity to gather that information, when and with what frequency that information can be gathered, to what extent that information can be verified, and whether the information can be gathered in an intelligible and actionable format that shapes regulation as well as public behavior.
Many of these questions remain unanswered. In other words, stakeholders concerned about responsible AI have an incomplete understanding of what information AI labs do collect, what information they can collect, which information they should collect, and what information such stakeholders need to know.
IIHS and other stakeholders faced similar questions with respect to automobile safety. How IIHS and others managed to answer those questions and to create an innovative regulatory ecosystem can and should inform the development of the proposed AI consortium.
Learning From IIHS
Automobiles were fairly ubiquitous prior to the emergence of efforts to regulate their design and traffic safety generally. Pressure to better regulate automobiles came about only once the number of drivers and, by extension, the number of accidents increased to such an extent that the public, the press, and regulators could not ignore the scale of the dangers posed to the public by a laissez faire approach to automobile design and traffic safety. Regulation, though, did not immediately follow—in part because the government had a flawed conception of how best to protect drivers and the public. In other words, the government wasn’t asking the right questions nor seeking out actionable information; it lacked the information necessary to justify and inform regulations of automobile designs.
Academics mounted the initial challenge to this understanding, which led to the creation and spread of Automobile Crash Injury Research reports. The specificity of the results as well as their indication of significant room for improvement with respect to vehicle design led automakers to swiftly adopt new safety mechanisms, such as installing safety door latches.
In a short period of time and with no formal mandate, the project demonstrated a potent regulatory formula: Conduct verifiable and reliable tests of specific safety mechanisms, disclose test results to automakers, and create inherent pressure on automakers to adopt responsive design changes. In 1959, a collection of insurers—like all good innovators (whether breaking or braking things)—recognized the merits of this formula and scaled it up by creating IIHS. The institute soon became an independent research organization with the goal of using “a modern, scientific approach to identify a full range of options for reducing crash losses.” Today, IIHS performs a wide range of crash tests on cars around the world and summarizes how cars perform in crash test ratings that have a demonstrated impact on both design choices made by automakers and purchasing decisions made by consumers.
That said, it is worth acknowledging the limitations of looking at automobile regulation to inform AI regulation. It goes without saying that regulating automobiles and traffic safety poses a different regulatory challenge than regulating AI. The risks and harms targeted by the respective regimes vary significantly—car crashes are immediate, observable, perceived as common, and have causes and consequences known to the public. AI risks, especially those related to existential risks such as cyberattacks on critical infrastructure or entrenchment of totalitarian regimes, may develop over years or decades, elude understanding by Average Joes and Janes (and even experts in the space), and present harms of unknown severity and duration.
Despite those differences, an assessment of the role IIHS played (and plays) in creating an innovative regulatory ecosystem can inform similar efforts in the AI context. In particular, specific aspects of the institutional capacity of IIHS merit consideration by contemporary AI stakeholders when forming the consortium.
IIHS Lesson One: Testing Infrastructure
IIHS continually iterates on its crash testing infrastructure to analyze new and forthcoming automobile features and to produce verifiable data that can be quantified in units relevant to regulators and the public. By way of example, IIHS’s “frontal crash tests” have evolved in response to (a) automakers producing vehicles capable of performing well on earlier iterations of the test, (b) automakers introducing new features, and (c) unexpected and unknown safety issues resulting from those new features as well as from new uses by drivers and passengers. Importantly, the results of these tests reach the public in the form of crash test ratings that Average Joes and Janes can understand and act on when considering whether to buy a car.
The AI consortium must also develop tests and benchmarks that anticipate or closely follow advances in AI models. Thankfully, the consortium would not need to start from scratch. Organizations such as Lab42 already have designed novel and evolving benchmarks that measure skill development in AI models. For example, Lab42’s Abstraction and Reasoning Corpus benchmark—which provides an indication of progress made toward human-level AI—is understandable to AI stakeholders, evolving in response to public participation in benchmarking tasks and AI model development, and replicable by other entities. Ideally, the consortium would scale these efforts and share results with the public and potential regulators. By finding new ways to measure the capabilities of AI models as well as their societal benefits and risks, the consortium will likely have the data necessary to foster consensus about certain regulatory proposals and influence consumer behavior.
IIHS Lesson Two: Technical Expertise
IIHS recruits and retains experts capable of developing responsive and stringent tests based on design choices made by automakers and developments in driver and passenger norms. They have collectively produced reports on nearly every topic under the topic of automobile safety. (Notably, these experts benefit from institutional knowledge that has been accumulating and passed on since 1959.)
The AI consortium must also create a staff made up of leading experts. This poses a significant hurdle from an institutional capacity standpoint. Generally, regulatory innovation involves the same if not a higher level of expertise as traditional innovation because experts in the latter tend to focus on the intended consequences of their product whereas those in the former must strive to map out and continually assess all of the unintended consequences—an inquiry that requires fundamental knowledge of the technology as well as a substantial portion of creativity.
This dynamic is at play in AI research, development, and deployment. Though researchers at OpenAI and similar labs have expressed their intent to understand their models as thoroughly as possible, they have nevertheless released models without fully understanding how they work, how the public will use them, and what externalities the models will produce.
Right now, the experts most likely to shed light on the “unknown unknowns” of AI models have strong financial and practical incentives to join AI labs rather than something like the consortium. AI labs offer significant salaries and, by virtue of having state-of-the-art technology and significant resources, the chance for experts to use the skills and knowledge they worked so hard to attain. Note that a similar dynamic has hindered the federal government’s ability to meet its cybersecurity staffing needs. Accordingly, this issue must be at the forefront of kick-starting the consortium or any similar entity.
The consortium will have to learn from IIHS and other entities that have managed to lure talent away from “Right Side” innovators. The Federal Reserve, for instance, includes leading economists, in part, because it has developed a prestigious reputation. Such a reputation, of course, takes time to build, so the consortium may have to start by simply fighting money with money—in other words, relying on financial benefits to initially recruit the expertise required to staff its operations.
IIHS Lesson Three: Social License
Once the public has become accustomed to using AI technology—even in a way that threatens their short- and long-term well-being—they may balk at safety precautions that they perceive as inconvenient. In the mid-1990s, for instance, many members of the public came to doubt the efficacy of seat belts. Thanks, in part, to the media highlighting examples of seat belts causing rather than preventing injuries, a dominant narrative took hold that motivated automakers and regulators alike to consider walking back this safety feature.
IIHS helped reverse this trend by virtue of having earned a social license to push back on regulatory proposals and automaker plans. This license was the result of IIHS having financial independence and having earned the public’s trust through its proactive and visible defense of the public interest.
On financial independence, since three major insurance associations launched IIHS, the institute has received a steady, dependable, and sufficient flow of financial support from insurance associations in the U.S. and Canada; auto insurers also contribute to IIHS. On the public’s trust, the public had decades of experience seeing IIHS push back on regulatory proposals and “innovations” by automakers—and, in doing so, protecting their wallets and, more importantly, their lives. For example, when automakers advertised their 1972 models as safer than earlier models, IIHS tested those claims and revealed that 1972 models required more repair costs when subjected to the same tests as their predecessors. Likewise, in 1975, IIHS conducted tests that indicated a proposed weakening of bumper standards would destroy cost savings afforded by the existing standards. These and many other actions ensure that IIHS recommendations have empirical support as well as the support of the public (or, minimally, the support of communities dedicated to automobile safety).
The AI consortium must similarly have the financial and political independence necessary to serve as a check on other stakeholders in the regulatory ecosystem as well as on the labs themselves—a function that, if sustained over time, will likely ensure the consortium earns the public’s trust. Financial independence represents yet another high hurdle to forming and sustaining the consortium. Like IIHS, the consortium may earn support from AI insurers should such an insurance market form. Alternatively, the consortium may consider following the lead of the Meta Oversight Board and establish its financial independence through a trust created and maintained by the largest labs. Finally, the consortium could rely on a funding mechanism akin to the Consumer Financial Protection Bureau, which receives financial support through the Federal Reserve rather than through the traditional congressional appropriations process. A similar setup would reduce the odds of the consortium bending to partisan interests and shield it from experiencing funding shortfalls that may undermine its work.
Conclusion and Next Steps
Advocates of AI research, development, and deployment that aligns with the public interest ought to prioritize the creation of the consortium. The current race among states and the federal government to enact AI regulation poses significant risks. Regulations formed while the information gap remains so large might have unintended consequences such as protecting incumbents, discouraging research in means to test AI models, and causing regulatory flight of labs to other jurisdictions with “friendler” laws. Given that regulation has been introduced in 25 states, the time is now to create the consortium. By doing so, lawmakers will increase the odds of regulation addressing the most likely and significant risks posed by AI.
This prioritization is further justified by the comparative ease of launching a version of the consortium. Unlike the political, financial, and temporal resources necessary to create an entity tasked with governing AI, the consortium’s mandate to measure and monitor AI would involve fewer resources and stoke fewer normative debates. In other words, arguments by senators such Ted Cruz (R-Texas) against any regulation that might cause the U.S. to trail China in AI development would have less weight in discussions around the consortium.
Finally, the information gathered and shared by the consortium could influence AI regulations around the world. The EU has already made substantial progress on AI regulation—how the EU AI Act is enforced and amended over time may benefit from guidance offered by the consortium. Likewise, the consortium’s work could detect and justify new safety measures—consider that the seat belt wasn’t a “thing” in the U.S. until legislators were bombarded by advocates sharing the results of myriad studies showing their efficacy—especially with respect to protecting children. The upshot is that regulatory innovation requires its own research and development—something the consortium would offer.
I intend to talk with anyone and everyone about this proposal. The consortium could take off as a government entity, a private entity, or a hybrid. IIHS often works with automakers, regulators, and other information gathering organizations to fulfill its mission—it follows that the consortium may benefit from directly involving each of those stakeholders in its day-to-day operations. So long as the proposed entity has the institutional capacities set forth above, it will have my support and should earn the support of AI stakeholders. That means a wide range of actors and institutions could play a part in bringing this idea to fruition. If you’re one of those actors, give me a ring.
Some legislators believe that the pace, scale, and unpredictability of AI will undermine any effort to mitigate AI risks. That does not need to be the case. The AI Benchmarking Consortium proposed here would reduce the lag between increases in AI capacity and a corresponding jump in understanding among stakeholders concerned about AI risks. If, however, a traditional approach to regulation is strictly and exclusively followed, then information asymmetry will continue to shape this issue and stakeholders may rue not having learned an adage of regulating emerging technologies: information before regulation.