Unlocking AI for All: The Case for Public Data Banks
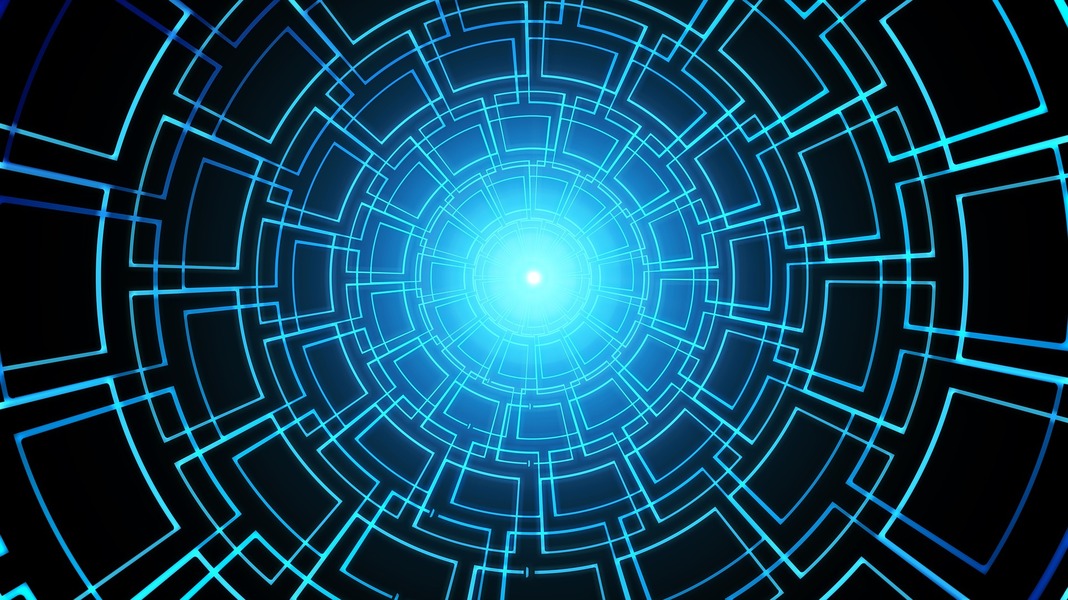
Published by The Lawfare Institute
in Cooperation With
The data relied on by OpenAI, Google, Meta, and other artificial intelligence (AI) developers is not readily available to other AI labs. Google and Meta relied, in part, on data gathered from their own products to train and fine-tune their models. OpenAI used tactics to acquire data that now would not work or may be more likely to be found in violation of the law (whether such tactics violated the law when originally used by OpenAI is being worked out in the courts). Upstart labs as well as research outfits find themselves with a dearth of data. Full realization of the positive benefits of AI, such as being deployed in costly but publicly useful ways (think tutoring kids or identifying common illnesses), as well as complete identification of the negative possibilities of AI (think perpetuating cultural biases) requires that labs other than the big players have access to quality, sufficient data.
The proper response is not to return to an exploitative status quo. Google, for example, may have relied on data from YouTube videos without meaningful consent from users. OpenAI may have hoovered up copyrighted data with little regard for the legal and social ramifications of that approach. In response to these questionable approaches, data has (rightfully) become harder to acquire. Cloudflare has equipped websites with the tools necessary to limit data scraping—the process of extracting data from another computer program. Regulators have developed new legal limits on data scraping or enforced old ones. Data owners have become more defensive over their content and, in some cases, more litigious. All of these largely positive developments from the perspective of data creators (which is to say, anyone and everyone who uses the internet) diminish the odds of newcomers entering the AI space. The creation of a public AI training data bank is necessary to ensure the availability of enough data for upstart labs and public research entities. Such banks would prevent those new entrants from having to go down the costly and legally questionable path of trying to hoover up as much data as possible.
Moving Toward a Competitive Market
To realize the full social and economic benefits of AI, regulators should prevent excessive concentration of the resources and talent to develop and deploy AI models. Competitive markets generally carry a number of benefits and innovation occurs at a faster clip when more companies engage in experimentation. As companies become larger, they tend to invest less in research and development (R&D). Instead, larger firms often acquire smaller firms in an attempt to simply purchase R&D capacity. But simply purchasing another firm does not appear to make up for the larger firm’s lack of investment.
Job creation also increases under competitive conditions. When an employer achieves more power in a labor market, it generally hires fewer people. In most years, dominant companies have fired more individuals than they have hired. Socially beneficial business practices such as higher wages or higher quality products may also take hold in a faster fashion when companies have to compete with one another on more than just price.
The unique attributes of AI make competition even more important in this market. AI is one of the most transformative technologies yet to be developed—if understanding and control of the technology is confined to a few hands, then consumers may have no choice other than to accept whatever risks and preferences the leading companies embed within their models.
Given the choice between imprecise and inaccurate AI (“bad AI”) or no AI, more than a few individuals and enterprises alike will find themselves opting for the former. Even though distrust of AI is already prevalent among employees and employers, many corporations are nevertheless doubling down on ways to integrate AI into their operations. Likewise, despite consumer concerns with corporate use of AI, there is no real evidence of consumers avoiding corporations that have leaned into the technology. And, although personal adoption of AI has been hindered by questions about its pros and cons, use of AI among the public is still on the rise.
Public officials may have to make the same choice, especially if allies and adversaries are finding ways to direct even bad AI toward national ends. In short, the government does not want to show up to a gunfight with a knife, even if the gun misfires every now and again. Of course, in an ideal world, the government would have its choice of many different AI tools—yet those tools may never come into existence in a hyper-concentrated AI market.
Possession of the key resources and expertise by a small number of private actors may also prevent the government and nonprofits from conducting AI research focused on public interests rather than private profit. Such an outcome would likely result in some positive, yet costly uses of AI going unexplored, such as the development of AI tools to tutor students in resource-scarce school districts.
A more competitive AI market and its positive externalities turns largely on the four key ingredients of AI development: expertise, compute, energy, and data. Expertise to build, test, and fine-tune models. Compute to build bigger, more capable models. Energy to sustain testing and fine-tuning of models. And data to train and fine-tune more sophisticated, accurate models. The costs to procure those resources form high barriers to entry. Increasing access to those core components is unlikely to come about without direct and sustained government intervention given the costs and coordination associated with the supply of each ingredient.
On expertise, even back in 2018—years before the current and ongoing explosion of investment in AI labs, AI researchers could make upward of $1 million per year. An increase in supply of AI expertise in the intervening years may have only slightly reduced the expense required to recruit and retain the best employees. Big Tech companies and startups alike are still fighting over a relatively small pool of experts. Government investment in education may help increase the supply of experts in the long run, but that’s hardly going to help today’s research and nonprofit organizations.
On compute, “many companies spend more than 80% of their total capital raised on compute resources,” according to venture firm a16z. The costs to train ChatGPT-3 may have exceeded $4 million depending on just how expensive the underlying hardware may have been. The scarcity and expense of popular computer chips—graphics processing units—explains why only the U.S. and China, two well-resourced nations, have a substantial share of the world’s total compute and why much of the world amounts to a “compute desert.”
Proposals to pool compute for public use have experienced some success. New York state, for one, is investing more than $400 billion in the Empire AI Consortium, a “computing center in Upstate New York to be used by New York’s leading institutions to promote responsible research and development, create jobs, and unlock AI opportunities focused on public good.” Calls for a National AI Research Resource (NAIRR) have also been well received. Both the Empire AI Consortium and NAIRR, if realized, would leave researchers and nonprofits with meager computing resources. The scale of investment in public computing must be orders of magnitude larger for the resource to fulfill its purpose.
On energy, training ChatGPT-3 may have required as much power as is annually used by 130 U.S. homes. Access to reliable and cheap energy, then, is a key factor in the ability of a lab to develop a frontier model. Though AI research is less energy intensive, research outfits will still need to prioritize finding sufficient energy to run sophisticated tests. The cumulative demand for energy may soon result in higher costs as supply struggles to keep up. Department of Energy officials are already working out ways to ensure sufficient energy supply for AI development for decades to come.
On data, upstart AI labs require enough data to train and fine-tune a model. Determining what constitutes enough data and identifying how to obtain that data is key to addressing this specific aspect of the barriers to a more competitive AI market. The government can play a key role in identifying, accumulating, and storing quality training data—and is doing so. For one, as a result of the Open Government Data Act, the government already houses more than 300,000 datasets on Data.gov. What’s more, the government has developed processes and procedures for lending that data to qualifying researchers. This data supply and operational capacity suggest that the government has the capacity to ease competitive barriers related to data.
The Role of Data in AI Development and Research
AI models are trained on incredible amounts of data. The median size of training datasets in 2013 was 1 million data points. By 2023, the median increased 750 times over. Development of the most capable models mandates an even greater amount of data. In general, the more advanced the model, the more data involved in the training process. MIT Media Lab Research’s Shayne Longpre notes that “foundation models really rely on the scale of data to perform well.” More than 3 million words went into the training of BERT—one of Google’s large language models. Relatedly, OpenAI “exhausted every reservoir of reputable English-language text on the internet” in developing its models.
But not just any data will do. Models need “high-quality and diverse training data,” which is increasingly difficult to come by. Whereas OpenAI and other labs had relative success in scraping the internet for such data, those same data sources have become physically or legally inaccessible. A recent review of the most common sources of content indicated publishers, platforms, and the like are instituting safeguards against data harvesting by web scrapers deployed by labs. In some cases, web sources have proactively implemented those preventive measures via the Robots Exclusion Protocol, which blocks bots from scooping up the site’s data. Other sources have deployed an anti-scraping tool developed by Cloudflare to shield their content. Increased familiarity with and enforcement of copyright laws and related legal protections of data may also hinder future scraping efforts.
Small AI firms and AI researchers may not survive in this new, more scarce data ecosystem. The viability of future use of the publicly available datasets that have long been essential to smaller AI efforts is up in the air. An audit of many such datasets revealed that the provenance of the data is often not disclosed, which could raise legal and ethical issues for labs and researchers. Absent sufficient public data, AI outfits may have no choice other than reaching formal arrangements with data owners. If licensing agreements between labs and data owners become the norm for obtaining massive datasets, larger firms will likely take a financial hit but ultimately be okay. The smaller firms, however, may face an existential crisis—the supply of publicly available training data may run out as soon as 2026. To make matters worse, investment in AI development is waning as concerns about an AI bubble spread. Put differently, “the primary impact [of diminished data supply] is on later-arriving actors, who are typically either smaller start-ups or researchers.”
Other sources of data are poor substitutes for the quality data increasingly behind data walls. Back in 2023, some AI researchers, including Kalyan Veeramachaneni, principal research scientist with MIT’s Schwarzman College of Computing, hoped that synthetic data might replace human-generated data in training and fine-tuning AI models. Subsequent research, however, indicated that overuse of data created by other models can result in “model collapse.” This diagnosis is as dire as it sounds. Model collapse is “a degenerative process whereby, over time, models forget the true underlying data distribution, even in the absence of a shift in the distribution over time.” In other words, model collapse may lead to the sort of unreliable, inaccurate results that consumers, corporations, and civil society have long feared. Small AI efforts and AI researchers cannot compete nor conduct quality research if synthetic data forms a sizable fraction of their training and fine-tuning data.
The increasing shortage of nonsynthetic data doesn’t quite mean the end of upstart AI companies and AI research. The good news is that fine-tuning AI and conducting AI research requires comparatively less data than training a new model. In fact, at some point, labs will hit diminishing returns as they acquire and use more data. The key, then, is not to provide smaller AI companies and researchers with all data but only enough data to achieve their stated aims. Provision of this smaller set of data is, of course, an easier policy challenge for those keen on reducing concentration in the AI market. It may soon be the case that progress in data and computer science renders the amount of data available to AI developers and researchers less and less important.
In the interim, though, public authorities should consider creating data banks exclusively available to qualifying AI labs and research entities. As mentioned above and explored in more detail below, unlike other actors in the space, public authorities already have extensive databases and operational capacity to run such an effort. With some slight retooling, that data and those processes could at least partially reduce one barrier to AI competition.
A Public AI Data Bank
Creation of public AI data banks (PAIDs) by subnational, national, and international authorities can address the anti-competitive effects of the largest labs exercising substantial data advantages. PAIDs would allow startups and researchers to use quality data—otherwise unavailable to the public—subject to specific conditions.
The idea of governments accumulating and sharing data with private and nonprofit actors is not new. More than 300,000 datasets are available via the federal government’s open data site, Data.gov. Some federal agencies have likewise shared specific data with external entities. Though better than nothing, the government did not gather and make public this information for the purpose of facilitating AI R&D. For example, according to the website, Data.gov “is designed to unleash the power of government open data to inform decisions by the public and policymakers, drive innovation and economic activity, achieve agency missions, and strengthen the foundation of an open and transparent government.” The facilitation of AI R&D is notably missing from this list. The good news is that many public and nongovernmental actors have already started trying to address that omission. The challenge is ensuring greater coordination among these disparate efforts.
An emerging, albeit small EU public data collective serves as a model for PAIDs. Rather than simply share as much data as possible, the EU efforts involve gathering data that would specifically assist with the development of AI in the public interest. The Alliance for Language Technologies aims to “increase the availability of European language data” with the goal of “uphold[ing] Europe’s linguistic diversity and cultural richness.” This data can serve a number of publicly beneficial functions. First, access to more, quality data in languages spoken by a relatively small group of people can aid labs in creating models that reflect the full diversity of the EU community. Second, researchers may be able to use the data to create benchmarks that assess whether commercial models adequately and accurately share information in different languages. This latter effort may be particularly important before public authorities adopt any commercial model—if the model scores poorly on the aforementioned benchmark, then the authority may need to search for an alternative or fine-tune the model.
As is the case with the Alliance for Language Technologies, the success of data banks hinge on collaboration. More than 16 countries have pledged to help create “a central platform for European language resources and collect high-quality data sets.” Community-driven initiatives to create publicly accessible datasets have followed or plan to follow a similar approach. Calls for the creation of bottom-up data trusts predated ChatGPT’s emergence in late 2022. The Mozilla Foundation acted on those ideals when it formed Common Voices, “the most diverse open voice dataset in the world.”
Those interested in scaling the efforts of Mozilla and others need to answer several questions. First, which entity would host the data bank or banks? Government possession of potentially sensitive data as well as data initially gathered by private sources might raise red flags. Centralization of important information in the hands of the government may result in unanticipated uses of that data by other government actors. An independent data authority that has legal and informal distance from the government might serve as a preferable alternative.
Second, which individuals and entities could use the data bank? At what point does a small AI startup become a dominant player that no longer needs assistance in acquiring essential development resources? May any research outfit tap into a PAID? If that research outfit begins to commercialize its work, will it receive access to fewer datasets? Should PAIDs issue calls for proposals to conduct specific research or develop specific models and reward access only to applicants?
Third, what strings will attach to the use of PAIDs? Will a PAID receive a fraction of all future income derived from models trained and fine-tuned on PAID data? Will research informed by PAID data be made public, or will researchers have the authority to keep their findings private?
These questions and surely many others warrant significant attention and deliberation. But determining how best to answer these questions puts the trailer before the SUV. Before these conversations even begin, the threshold matter is agreeing that concentration in the AI market merits regulatory intervention. Other strategies to foster competition, such as preventing compute stockpiling by a few entities, also should be on the table and may be pursued concurrently.
***
Concentration is concerning in any market. Corporations have proved adept at transforming economic power into social and political power. Leading AI labs have rapidly distanced themselves from competitors—latecomers now find it harder and more expensive to recruit talent, obtain compute, and acquire data. Regulatory inaction will only heighten these barriers to entry. Prior delays in identifying and breaking up new concentrated markets fill history books—Presidents Theodore Roosevelt, William Howard Taft, and Woodrow Wilson had to break up trusts because of decades of acquiescence by each branch; Presidents George W. Bush and Barack Obama had to bail out banks because they had been permitted to become too big to fail; Presidents Donald Trump and Joe Biden have struggled to rein in social media platforms that seem to operate beyond legal bounds. Whoever wins the impending election will have to decide if fostering AI competition sooner rather than later is a legislative priority. If so, public data banks may ease the ability of upstart AI companies and AI researchers to break into this space.