Lawfare Daily: Helen Toner and Zach Arnold on a Common Agenda for AI Doomers and AI Ethicists
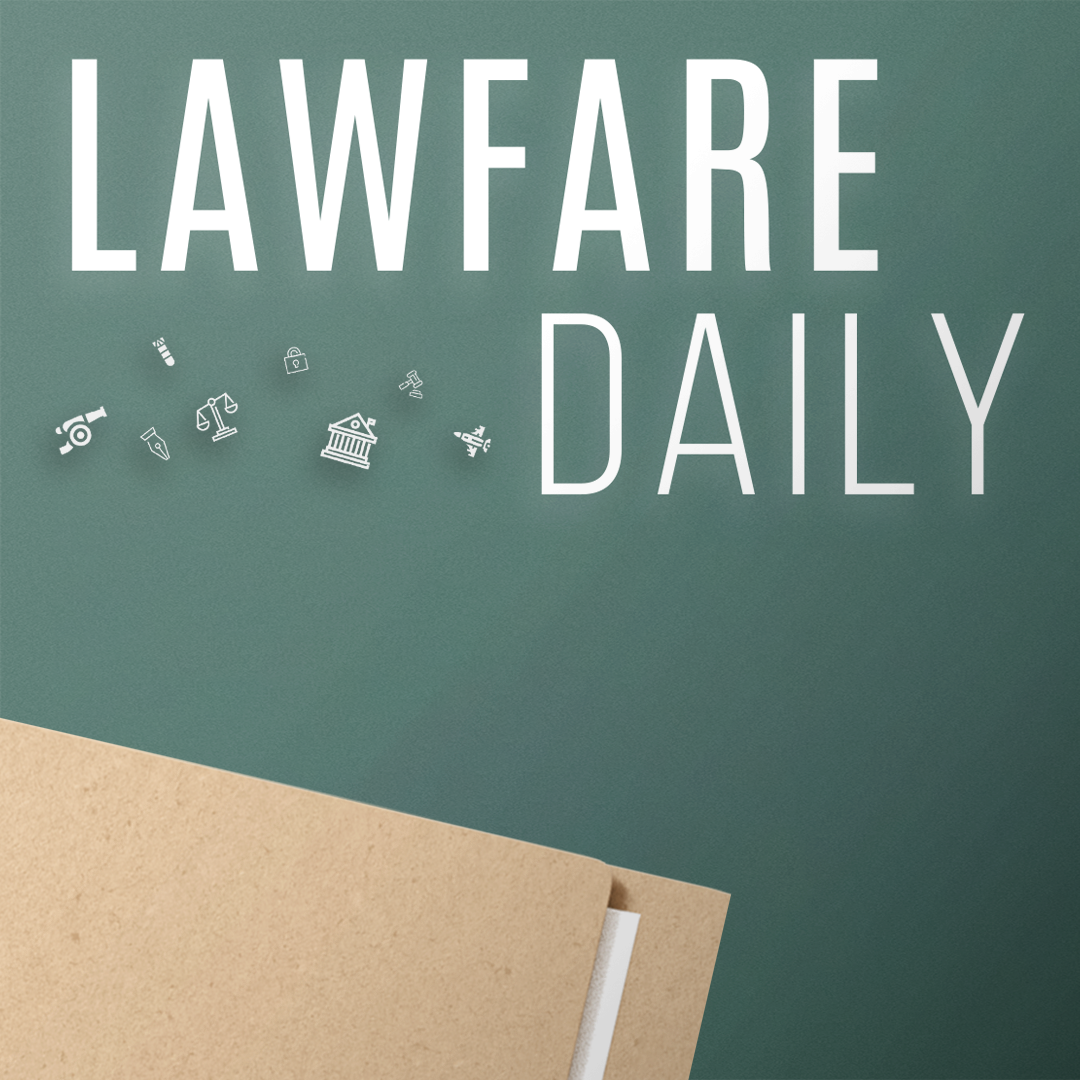
Published by The Lawfare Institute
in Cooperation With
Helen Toner, Director of Strategy and Foundational Research Grants at Georgetown University's Center for Security and Emerging Technology (CSET), and Zach Arnold, Analytic Lead at Georgetown University's Center for Security and Emerging Technology, join Kevin Frazier, Assistant Professor at St. Thomas University College of Law and a Tarbell Fellow at Lawfare, to discuss their recent article "AI Regulation's Champions Can Seize Common Ground—or Be Swept Aside." The trio explore the divide between AI "doomers" and "ethicists," and how finding common ground could strengthen efforts to govern AI responsibly.
To receive ad-free podcasts, become a Lawfare Material Supporter at www.patreon.com/lawfare. You can also support Lawfare by making a one-time donation at https://givebutter.com/c/trumptrials.
Click the button below to view a transcript of this podcast. Please note that the transcript was auto-generated and may contain errors.
Transcript
[Introduction]
Helen Toner: I definitely think that the sort of feuding or kind of disparate perspectives between these different groups also had an effect because I think it meant that once policymakers started calling in experts and saying, okay, what do we do? What problems should we worry about? How do we fix them? If they're getting, you know, five different answers when they call five different people, that's very difficult. It makes it very difficult to kind of coalesce around a certain set of solutions.
Kevin Frazier: It's the Lawfare Podcast. I'm Kevin Frazier, assistant professor at St. Thomas University College of Law and a Tarbell Fellow at Lawfare, joined by CSET's Helen Toner and Zach Arnold.
Zach Arnold: When things have gone wrong with these systems, we want to know that that's happened. We want to understand, to the extent we can, what contributed to those failures, and we want to diffuse that information back out to the community of model developers and others. You know, that, that kind of just makes sense as a matter of good practice. And it's not something we have in any systematic way in AI right now.
Kevin Frazier: Today we're discussing the landscape of AI governance advocacy and potential paths forward based on Zach and Helen's recent Lawfare article provocatively titled “AI Regulations Champions Can Seize Common Ground or Be Swept Aside.”
[Main PodcasT]
Helen, your recent Law Review article describes two camps in AI regulation advocacy, the doomers and the ethicists. Who are these folks and what are their respective priorities?
Helen Toner: We should probably start by saying that, you know, we talked about two camps because that makes it easy to write an article to go back and forth between two perspectives. Of course, in reality, the world of AI policy advocates and commentators is much more multidimensional and complicated. But the simplification we offered was between, I think maybe a better term that I would use if it weren't really wouldn't get really unwieldy in a written piece would be people focused on current harms from AI. So, meaning looking at what kinds of things are we already observing? We're observing nonconsensual deep fakes. We're observing algorithmic bias affecting people who are subject to decisions being made by algorithmic systems or AI systems that are somehow making decisions that are not working well for the demographic group they belong to. These other, you know, lots of different examples of ways that AI systems are already causing harm in the world today, and there's a significant community based around thinking about what, you know, what are those harms? How do we prevent them? And so on. In a separate community, which, you know, so we call that first community, the ethicists in the piece for brevity.
the separate community, sometimes known as doomers though I think that that term really, it's very unclear who exactly counts as a doomer. But certainly, there is a community focused on looking at more, sort of future focused risks, anticipated risks, speculative risks. People have different words for this, but this is more thinking about, you know, we've seen this trajectory of AI getting more advanced over the last 10 years, 20 years, 50 years, and what happens if we project that out? If we think that these systems might keep getting more advanced, might at some point be, you know, as capable, as smart as a human, maybe more capable or smarter. Obviously, all of these definitions, what is smart, they're all very contested. but the basic idea is you extrapolate out the trends, you have much, much more sophisticated computers and machines that can do things we currently think of as only things that humans can do.
There's a whole community, you know, that thinks about what are the risks that come from that? Does that lead to human extinction? Maybe. Does that lead to other really bad outcomes like total disempowerment of humanity? Maybe. And so, that is sort of the other camp that we describe here. And as we get into in the piece, in some ways, these two camps, you know, have a lot in common in that they're looking at this technology and saying, oh, we have concerns, we think there's a need for greater involvement from government, from civil society, from others, but in practice, they often come to, to loggerheads.
Kevin Frazier: What's really interesting to me is I agree that there's certainly a spectrum, right? Of some people have a portfolio of risks they're concerned about, THey may be 30 percent an ethicist and 70 percent a doomer. So there's certainly a spectrum, but I do think it's worth pointing out how many people, if you just get on X right now, in their tagline, their little byline will identify explicitly as a Doomer or an e/acc, an Effective Accelerationist. So the willingness to self-identify is kind of interesting and with that sort of self-identification and the entrenchment of some of these camps. Zach, is the difference between these groups more akin to the Jets and the Sharks, which is to say serious and perhaps irresolvable, or is it more like a dispute between members of a boy band, which is to say fleeting and flexible?
Zach Arnold: Well, I think, you know, our piece is arguing that maybe the boy band scenario we could get a little closer to. In practice, you know, there are serious divides between folks who tend to embrace, you know, this doomer label, which among other things makes it convenient to write about them, and those who are, you know, focused elsewhere, right? I think we point out in the piece that in the piece, there's you know, sociological divides, political divides. A lot of the concerns of sort of the ethicist camp, as we term them are, you know, focusing on racial bias, gender bias, marginalization of disempowered groups, and how AI is affecting it and could affect that in the future. On the other hand, you know, there's among the doomer contingent, again, to the extent you can draw lines around it, a lot of them are, you know, coming out of Silicon Valley, maybe a little more sort of libertarian or unclassifiable in their political leanings. So there is kind of, you know, some political and ideological valence to this.
There's also, I mean, to be frank, in my opinion, there's a little bit of just sort of, this for many years was an academic debate, you know, vigorously contested and now that debate is spilling into DC, spilling into, you know, all sorts of places and all sorts of discussions as AI becomes, you know, an economic and political force. So, you know, the aphorism about, you know, academic politics are so contentious because the stakes are so small, I don't think applies here, at least not anymore, but you still do see some of that vigorous debate. And I think part of our motivation in writing this piece was to say, step back, you know. The fundamental frame that you all are in is not as different as you might think and there is common ground that is a little bit underexplored, certainly at a minimum relative to, you know, other groups who are increasingly coming into this debate that was, you know, previously kind of the doomers and ethicists fighting it out and have a very different perspective altogether, you know, whether let's do very little or let's do nothing on the regulatory front.
Kevin Frazier: And what's interesting is that for so long, or for as long as AI has been a policy concern, we heard this narrative that, oh, you know, it's not necessarily a partisan issue, and we can't really map this on to blue and red categories. But you all kind of suggest that there may be some increasing signs of these camps moving into particular partisan communities. Helen, can you describe a little bit more about how you see maybe a partisan home for each of these groups or maybe an increasingly receptive audience among some partisan communities?
Helen Toner: I'm actually not sure that I would agree that it's becoming more partisan. I'm unsure, and it, you know, AI policy touches so many different topics that there are definitely places that are that are slightly more partisan than others. One way in which this maybe is relevant is kind of what Zach touched on of what are the communities that these different groups are coming from. So you do have some advocates who are clearly coming from more of a left-progressive perspective, and they're going to tend to center issues that are more, you know, familiar to the left-progressive camp, so things like climate or labor rights or those kinds of questions. Whereas you also have, you know, others coming from more of a sort of tech/Silicon Valley, maybe somewhat national security-inflected area. But I think it's interesting when you look at the specific, you know, policymakers and policies that are being proposed here, I don't think it breaks down particularly clearly on partisan lines, which I think is a good sign for the space cause obviously that's, you know, down that road lies dysfunction and paralysis.
But if you're, so you know, you look at things like in, in Congress and I think some of, you know, the work that's being done by, you know, Blumenthal and Hawley in the Judiciary Committee of the Senate, for example, or looking at, you know, you certainly have, you know, the Ted Cruzes of the world who are just anti all regulation in every form, but that doesn't seem to have been taken up as a kind of rallying cry for the Republican Party as a whole on AI. If you look at, you know, for example, yeah, again, Hawley or Mitt Romney proposing frameworks or others, Jay Rinaldi in the House being very active on these issues. So I think it could just be, you know, wishful thinking on my part, but I like to think that we're not actually, it's not at all clear that we're getting into kind of real partisan entrenchment here. Though, of course, you know, we'll see what happens with the upcoming election and continued progress in the tech.
Kevin Frazier: It is a really interesting space to see the evolution of, I guess it was the fall of 2023 when Senator Schumer and I believe Chair Khan, each may have suggested that they had a P(doom). We're not hearing so much about p(doom)s anymore, and innovation, it seems, has become a much more frequent moniker of certain senators and certain members of the House. So, Zach, earlier you were mentioning that some new players have come to the fore in a lot of these regulatory debates, and I think that's maybe most evident actually in California right now in the ongoing debate about SB 1047 and Big Tech, at least partially being opposed, if not vehemently opposed, depending on who you ask, to what others regard as light-touch regulation. So how has the intervention of Big Tech kind of changed this regulatory paradigm and maybe made things a little bit more complex?
Zach Arnold: Yeah, I mean, from, from where I sit in D.C. and, you know, the folks working on SB 1047 out in California I'm sure could give you a different earful, but, you know, we've seen from my perspective, what was really a specialist domain in some ways a very obscure domain. I mean, particularly before sort of ChatGPT and the advent of sort of generative AI and the public consciousness, this has become sort of a major battlefield for, you know, economic, major economic and political interests as its economic and political significance has grown as a technology, right? It's no longer the case that it's sort of a handful of enthusiasts or folks with, you know, the foresight or the inclination to think about AI. You now have, you know, tens of millions of dollars, if not more, going into lobbying in D.C. You have major corporations sort of fully mobilized, developing very coherent regulatory or anti-regulatory agendas.
This is, and I think again, part of the motivation of the piece is just to underscore, this is not a space where sort of the, you know, the ethicists and the doomers, as we simplify them, are sort of the only actors. You know, one's going to win over the other in the end and get its way. The trends right now, to be frank, looks like both of them might end up marginalized by, you know, very sophisticated, very powerful political and economic actors who have their own set of interests that don't necessarily correlate with the regulation of any risks, whether those are risks happening right now, or risks that might emerge in the future. I think SB 1047 is sort of the culmination of this sort of phase where you see regulation, you know, very much championed by, you know, I, in my view, one of these two camps, but nonetheless sort of people with a orientation toward this is an issue one way or another, we need to regulate, coming up against in some ways sort of single handedly conjuring into being a, a large, very well organized trans-partisan, to Helen's comments, and, you know, potentially victorious opposition. You know, we'll see in the end what Governor Newsom does. But I think this has been sort of, among other things, fascinating to watch sort of the development of, in many ways, sort of normal high stakes political showdown in an area that just hasn't quite seen anything like that yet.
Kevin Frazier: It is staggering when you consider, as Helen was pointing out, you know, Senators Hawley, Blackburn, and Romney all being concerned about AI on the kind of R side of things. And then on the D side, Majority Leader Schumer, Senator Blumenthal. This is a hot topic. And if you were just to have that list of players on a sheet and say, all of these people are concerned about AI, you would be, you know, I'm no Nate Silver, but I would bet on the idea that some legislation would have come across President Biden's desk by now with respect to AI. But instead, all we're looking at is this EO. And I wonder when we're thinking about the lack of success so far. How do you all kind of diagnose why we've seen such stagnation on the Hill? Is it just because of this opposition between the ethicists and the doomers not being able to agree? Or is the larger factor this new formation of, as you were pointing out, Zach, this concentrated and focused effort by Big Tech to perhaps oppose any meaningful regulation? And Helen, I guess I'll turn that to you.
Helen Toner: Yeah, I think I would probably say all of the above. I think certainly there was an effect you could see in the months after ChatGPT came out, and there was this enormous wave of public attention, this enormous wave of policymaker attention. And from, you know, my perspective, kind of inside the field of AI policy, that was pretty surprising to people who had been paying attention to how the tech had developed. You know, by the time ChatGPT came out, that was not technologically super new. There was a little bit new in there. The interface was new. The fact that it was freely available to the public was new. And the fact that it wasn't prone to some of the obvious failures that previous chatbots had had trouble with. But I think there was a little bit of a, like readjustment period where people who had been working in this space, they weren't actually necessarily ready to have their big moment in the spotlight. I think that was one factor.
I definitely think that the sort of feuding or kind of disparate perspectives between these different groups also had an effect because I think it meant that once policymakers started calling in experts and saying, okay, what do we do? What problems should we worry about? How do we fix them? If they're getting, you know, five different answers when they call five different people. That's very difficult. It makes it very difficult to kind of coalesce around a certain set of solutions. You know, I think they made some progress towards here are some potential ideas for things we could implement, but then as soon as there was a real chance of that getting passed, you know, that's when the Big Tech lobby kind of mobilized against that.
So I think it is kind of multifactorial, which is always the boring answer, but one thing we're really trying to do in the piece as well is point out that I think AI conversations, I think there's, AI policy conversations, there's two failure modes that I really think we need to move past. One is trying to handle everything all at once, and so saying, oh, we shouldn't pass this bill because it only handles problem A. It doesn't handle problems B, C, D, and E. And, you know, if you try to, if you apply that standard, then you're never going to pass anything because, you know, the chances of Congress really agreeing on a comprehensive bill that figures out everything at once is, minuscule. So the one problem is that, you know, trying to handle everything at once problem.
I think the other problem is there's lots of ways to slice things up in AI. So, people will often kind of get into like different sectors, you know, how are different sectors being, being affected by AI or different sort of manifestations of problems. And so something we really tried to do in the piece was, I sort of think of it as policy building blocks that are pretty basic and pretty fundamental, and that you would really want to have in place at this point because they're, you know, the absence of them means that the field is really just totally empty and really lacking. So, the kinds of things that we're suggesting, I think, didn't necessarily emerge as top priorities because they're not necessarily, you know, if you're thinking about a particular issue, a particular type of harm, you don't necessarily first come to these kind of building blocks. But if you're looking at how do we build a broad coalition, how do we make recommendations that can get a lot of consensus? I think that's where you start to come to more of the kinds of things that we that we talk about in the piece.
Kevin Frazier: Seeing this sort of analysis by paralysis that you're calling to attention, right, where there are so many bills now. I mean, if you go on any AI legislation tracker, you are scrolling for pages of just all these different avenues you can go. And I think as you were pointing out also, Helen, the difficulty of some of these pieces of legislation being framed as litmus tests. Right. If you're not this, if you're not for this piece of legislation, then we don't want to work for you at all, or with you at all. And that kind of approach just doesn't fly in D.C., right? Folks don't want to be pushed into a corner. Ultimately, especially as long as we have the filibuster, you're going to have to get to 60 senators. And getting 60 senators to agree on anything is difficult, but if you're going to make it a sort of litmus test approach, then you're almost certainly not going to get there. So turning to some of your regulatory low hanging fruit, I guess the first one I'd love to dive into is this idea of investment in AI measurement science. And just saying those words doesn't, I think, evoke a whole lot for a whole lot of people no offense, but Zach, can you explain what are we talking about here?
Zach Arnold: Yeah, no, we're really going for, you know, the fame and fortune when we're pushing AI measurement science there. But I do think, you know, Helen's point of thinking of all these recommendations and measurement, maybe more than any one of them as sort of building blocks as fundamental infrastructure for any sort of meaningful AI regulation, whether you're concerned on the present risk or on the future risk. That's the approach we take. And I think, you know, measurement sounds very abstruse, but basic questions, is this model safe? You know, is this model biased, whether that's along a racial dimension, whether that's along a gender dimension? You know, will this model act reliably in a certain context when integrated with another system? Can we trust it? These are very basic questions that we do not have great answers to at this point. I mean, there's lots of very high-quality work being done in the field of AI measurement and evaluation, but it's early, early days. And, you know, even when there are promising approaches being developed, being released, there's a lot of work that needs to be done to sort of standardize them, to diffuse them comprehensively through society and make sure that everyone who should be working to those standards is set up with them.
And so, you know, this is another theme that's come up, I think, in some of the more thoughtful commentary on SB 1047 in California. Folks are saying, you know, hey, you're trying to get us to evaluate ahead of time, you know, whether these models are safe or else we'll come in for these big penalties. We don't even really know how to answer those questions yet. And that, that's an objection that I think in some cases is cynical, and in some cases is not. Like, I really empathize with it. We need better answers to those sorts of fundamental questions and that's where public investment in this common good of measurement and technique can be really critical. And we see that in itself as a form of regulation, right? You know, you want to oppose SB 1047 because we don't have, you know, the building blocks for that kind of regulation? All right, advocate for this one. You know, that's our argument in a nutshell in some ways.
Kevin Frazier: No, well, yeah, I'm not certain it's going to sell on TikTok, but I do think this AI measurement science is, is quite important. And in particular-
Helen Toner: Kevin, you didn't grow up dreaming of becoming a metrologist? That's what this is, metrology. We got to, it has to make a comeback,
Kevin Frazier: You know, to be honest, that was never the goal. I think going into vexology, actually the study of flags, that was, that was my other pursuit, speaking of random jobs. But this AI measurement science, as you were pointing out, Zach, this focus on investment too, it's not necessarily, hey, we need to agree tomorrow on this specific eval or this specific approach to auditing, but just investing in that science to begin with is the sort of R&D we've seen the government fund in other contexts. And so I do agree as you all have framed the conversation too, if you're an ethicist then you're excited about knowing more about these models and what harms they may cause to specific communities. And if you're a doomer, you also want to know what are the odds of this model ending the world. So in both contexts that that measurement science makes a lot of sense.
So, I guess your kind of second focus here would be on the importance of an effective ecosystem of third party AI auditors. So, Helen, can you distinguish between measurement science, and evals, and this idea of auditing and how a consensus may form behind this approach to creating an ecosystem of auditors?
Helen Toner: Sure. Essentially, I think the distinction is that the role of auditors or third party, independent third-party evaluators is to be testing claims that companies are making themselves about their AI systems. And so this is less about sort of the fundamental research of, well, how do we determine, you know, which model is smarter than which other model or which risks it might pose, and more about kind of a, governance or an access question of, an even an information question of who is able to actually make determinations in a given case. And so of course, you know, auditors, people hear audit, they think financial audit, where you have someone independent going in and verifying that a company, you know, when the way that they're describing how they did their books is accurate. You know, they've gone through and they've done everything in a legitimate way. But there's roles for, you know, third party certifiers, third party evaluators in lots of different industries in terms of going in and checking that things have been done according to some standard or going in and checking that the claims being made by a company are legitimate.
And in general, I think that a huge problem for AI policy right now is, sort of the information asymmetry between folks in industry who are working on extremely advanced systems and testing them and kind of making things up as they go along and determining for themselves what they think is appropriate. And regulators in the general public who are kind of only really able to see what's released via press releases, you know, occasionally you have a whistleblower come out and give you some additional information. But I think part of what I see the role of a third-party ecosystem being is giving you more ability to get access to some of that internal information.
An alternative way of doing it, of course, is to just literally send in government regulators. The challenge of that is whether they can be nimble enough, whether they can be sophisticated enough technically, whether they can, you know, hire and fire the right number of people to keep up with a growing or shrinking industry. So, you know, in a lot of other spaces, we've seen this idea of you have some kind of third party, set of third-party bodies where companies get to choose who they're going to work with. The government gets to choose who is, sort of counsels good enough, as a very flexible mechanism that kind of balances out some of these information and power asymmetries that you have by default.
Kevin Frazier: And what seems so important to me about y'all's approach is you're not just calling for audits, period, right? Because this idea of auditwashing for lack of a better phrase, has been very real in the social media context. I think there's plenty of folks who would say, you know, a human rights audit of you name the platforms, content moderation strategies, is a little sketchy sometimes as to the quality of the results. Aand so, can you describe why you all see the public company accounting oversight board? And for folks who aren't in admin law, we love to call that peekaboo. Helen, can you tell us more about why you think the PCAOB may be a good model in this context?
Helen Toner: Yeah, so this is a recommendation that we took from a paper by Deb Raji and collaborators looking at third party ecosystems in other industries and what we could learn from AI. And essentially, you know, the core idea here is just that you, rather than having, again, you know, the government going in directly to companies and trying to handle things themselves, you want to have, you know, independent third party, you know, market driven, ideally companies that are, that are handling this. But you also want to have quality control, and so I didn't actually know what peekaboo was the way people pronounce that acronym. I love it.
My understanding of PCAOB's role in the financial industry is that they are setting standards. They are looking at companies that are, you know, selling their auditing services and saying, are you doing an adequate job? Do you get the stamp of approval? That if a company uses your services, then they're fulfilling their duties. So it's essentially just a question of roles and responsibilities and saying, okay, the government shouldn't be literally going in and doing this auditing work, but they can be setting the quality standards and making sure that private companies are kind of living up to those quality standards if they want to offer auditing services in this space.
Zach Arnold: You know, this point about scrutiny of audits, whether it's audits that are now happening mostly internally or as third-party auditors start emerging is really important. And kind of dovetails with another one of our big points in the piece, which is sort of transparency, not necessarily just to government regulators of auditors, but transparency of the broader public, right? Disclosure of these audits could be a really big deal. It's something that we see in a lot of other industries. And it's one sort of very important category of information that is getting strategically disclosed, partially disclosed, not disclosed at all right now. Having sort of a deeper public information ecosystem in this auditing aspect and in several others that we talk about I think will be really important, just getting that sunshine in.
Kevin Frazier: Yeah. And in particular, I think a huge issue that's going to have to be addressed is how are these audits going to be done in a way where it's not just transparency theater, but produced in a sort of language and tone that's actually understandable by the public. I mean, already I'd say the number of people who could describe a neural network to you probably isn't that high. And when we see audits on, for example, oh, you use this training data, but not that training data, my hunch is that the average Joe or Jane, isn't going to say, ah, yes, now I know not to use that model. And so having some government entity that can say, hey, not only do you need to be robust in your audits, but here's how you should produce it in a way that may actually facilitate public discourse is going to be really interesting and a huge challenge. But we've got to start somewhere as Helen pointed out, we can't just wait for the Omnibus AI bill. So finally, one third issue that you all suggested may be ripe for consensus is tracking AI related incidents. So Zach, I'll, I'll turn back to you and ask you to kind of detail why you think this may be a good area.
Zach Arnold: Well, I think on one level, it's sort of common sense, right? You want to be able to learn from failures. You want to be able to learn from mistakes. And in a lot of other areas of, you know, sophisticated technologies that can go really badly. We've recognized for a long time that this is sort of a critical element of regulation. You know, think about any time a plane crashes, you have government inspectors on the scene, you know, very quickly. We have, you know, technologies that we've developed for this particular context, right? Blackbox technologies and so forth. Detailed reports are produced and so forth. That's maybe a longer-term vision, but I think the general point that when things have gone wrong with these systems, we want to know that that's happened. We want to understand to the extent we can what contributed to those failures and we want to diffuse that information back out to the community of model developers and others. You know, that kind of just makes sense as a matter of good practice, and it's not something we have in any systematic way in AI right now. There's a lot of sort of civil society and nonprofit efforts that are really good. The AI Incident Database is one that I've had the pleasure of working with a little bit, but in general, you know, I think both supporting those civil society efforts, but also sort of putting government scale resources, government scale investigatory powers and just some, some more durable infrastructure around those efforts is really important.
And again, is an approach that encompasses all sorts of, you know, potential concerns about AI, right? Like, whether it is a model that has been found to have misdiagnosed a lot of patients of a particular race, or it's a model that's just sort of acting unpredictably in a way that, you know, could potentially lead to bigger problems as models become more sophisticated. Right, like, you can imagine implications for many different kinds of AI risk. On both fronts. So we want all that information coming into one place and sort of being useful to, to people of all sorts of perspectives on the issue.
Kevin Frazier: So a lot of this boils down to just information gathering, which makes a ton of sense if you're in a novel field, if you're dealing with an emerging technology, it helps to understand what you need to govern before you actually go to that next level of questions about maybe steering it in particular normative directions and things like that. So Helen, obviously, it's a great article. It's on Lawfare, so it's top of the top. And you all are wicked smart individuals. So tell me what the response has been: are senators just clamoring for implementing this, these ideas? Have you received any outpouring of support from civil society? What's the response been like?
Helen Toner: It's been really encouraging. I think, you know, the first sign of success is I think we've seen a lot of positive uptake, you know, for retweets and, you know, positive comments, nice emails from people on both sides of, you know, the doomer-ethicist split, if we're going to call it that. That has been really validating to see different people who are known to represent pretty different poles in the debate saying, yes, we agree, these are good building blocks. That's a good place to start. I think right now, a lot of the discourse around AI policy is really focused around SB 1047. And I think the, the piece doesn't draw explicit links to that. So I think we'll wait and see how it kind of percolates into the broader debate. My sense is at the federal level, a lot of people are at this point just waiting for the election. So I'm hoping that this can be something that we can build on and point to over the years to come.
I also do want to just call out if I can, you know, a couple of extra things in there that are, that we recommend that do go beyond just sort of gathering information. One that's really important, and again, really nerdy sounding is building capacity in government and in the public sector to do to make good regulations on AI, to handle AI issues well. So this is about, you know, how well can they hire a technical talent? What kind of education is available to people in these roles? And another is looking at liability and accountability and so can we do better than, I know you know, a topic that, that Lawfare has done a lot of great work on is software liability, cybersecurity liability, not in my experience. I'm not a lawyer, but my impression is those are not known as fields where liability law is functioning, you know, at its peak. And so can we do better than that when it comes to AI in terms of setting some guidance or, or ensuring that, that liability is allocated a little better through the different actors in the supply chain. So yeah, I think really positive response so far, excited to kind of pick this up and move it forward as there's more energy for, for AI policy in the future.
Kevin Frazier: And I'd be remiss if I didn't call out Gabe Weil's scholarship on software liability in particular. He's written pieces for Lawfare that curious listeners should check out. And Chinmayi Sharma out of Fordham has also written on this topic. So for those of you who are saying I am inspired to find this strange bedfellow coalition and make it real, that may be two places to start. And Zach, speaking of kind of the future work that is ahead of anyone interested in AI regulation, regardless of who wins the 2024 election, are there any other areas that you all have turned your focus to now, or if you were to write the piece again today, would you add any new proposals?
Zach Arnold: I mean, I think, you know, having, again, like Helen was saying, having developed this piece as the debate over SB 1047 has been evolving, as, you know, the bill itself has changed quite a bit, and seeing, like I was describing earlier, a lot of reactions that I, you know, I'm not sure entirely in good faith, but see the reason behind, of it's so early, we don't know what we're doing, how can we like put a strongly prescriptive regulation in mind, has just got me thinking again along the direction in the piece about you know, how do we develop regulation that is flexible, that iterates on itself, that develops the information that it needs to become stronger, right? And I do think, I mean, like we say in the piece, you know, this is not a comprehensive agenda for AI governance. And I'm not sure we're at the point yet where we could articulate one for all the reasons we describe. But continuing to think about again, how do we build the foundation through regulation? Not just waiting for, you know, some scientists or activists out there to create the preconditions for, you know, the ground rules for AI, but how do we use regulation? How do we use government investment and these other public mechanisms we have to sort of build that vessel so that we can, you know, go ahead? So maybe that's just a little bit more of the same. But again, I think that that line of thinking has, you know, as I've been watching California, just been over and over for me.
Helen Toner: And if I can jump in there as well, I think that in my mind, the huge challenge for, for AI policy over the coming years will be, to put a finer point on something Zach was saying, to keep pace with the field, not just as it's moving, you know, fast in one obvious direction where we all know where it's going and it's just a question of how quickly it gets there, but also there's just so many dimensions of uncertainty of what kinds of systems are going to be capable of, what kinds of things? Where will AI be deployed in, you know, which kinds of sectors? What kind of harms will we see? And, you know, if you listen to some of the best funded, most advanced AI companies are telling you, yeah, we might have artificial general intelligence in three years. You know, if that's true, we better be getting our skates on, you know, and ready to figure out how to handle that. And we better be, you know, the idea behind some of these, these policies is that they would help us figure out if that is true along the way. On the other hand, you know, other people have very different expectations. And so I think that the challenges for policymakers is to figure out how to be responsive and adaptive and knowing as quickly as possible what kinds of things to try and whether they're working. So that I think is, you know, one big underlying motivation for, for many of the ideas that we presented here.
Kevin Frazier: Well, and if AGI does come about, Helen, I will let you take the skates. I'm going to go ahead and like find a mansion to defend and protect, you know, whatever I can do. I may be more aggressive than just finding a pair of skates, but I'll leave those to you. But on a more serious note, I do think you all are really hitting on a point, and again, this is where I transition into shameless self-promotion. My own take on this is the need for some sort of research regulation cycle where we've really not focused quite yet on the importance of research on understanding the technology itself before getting to some of those headier, more specific regulatory approaches. And so it was very refreshing to see you all propose these tangible set of solutions that hopefully we can see a coalition form behind, and regardless of who wins in 2024, the website linking to your great work will continue to be available for anyone who's curious and decides that now's the moment for hitting some of these low hanging targets. So thank you to you both. And I think we'll go ahead and leave it there.
Helen Toner: Thanks so much, Kevin.
Zach Arnold: Thanks for having us.
Kevin Frazier: The Lawfare Podcast is produced in cooperation with the Brookings Institution. You can get ad-free versions of this and other Lawfare podcasts by becoming a Lawfare material supporter through our website, lawfaremedia.org/support. You'll also get access to special events and other content available only to our supporters. Please rate and review us wherever you get your podcasts. Look out for our other podcasts including Rational Security, Chatter, Allies, and the Aftermath. Our latest Lawfare Presents podcast series on the government's response to January 6th. Check out our written work at lawfaremedia.org. The podcast is edited by Jen Patja. Our theme song is from Alibi Music. As always, thank you for listening.