Lawfare Daily: Kevin Frazier on Prioritizing AI Research
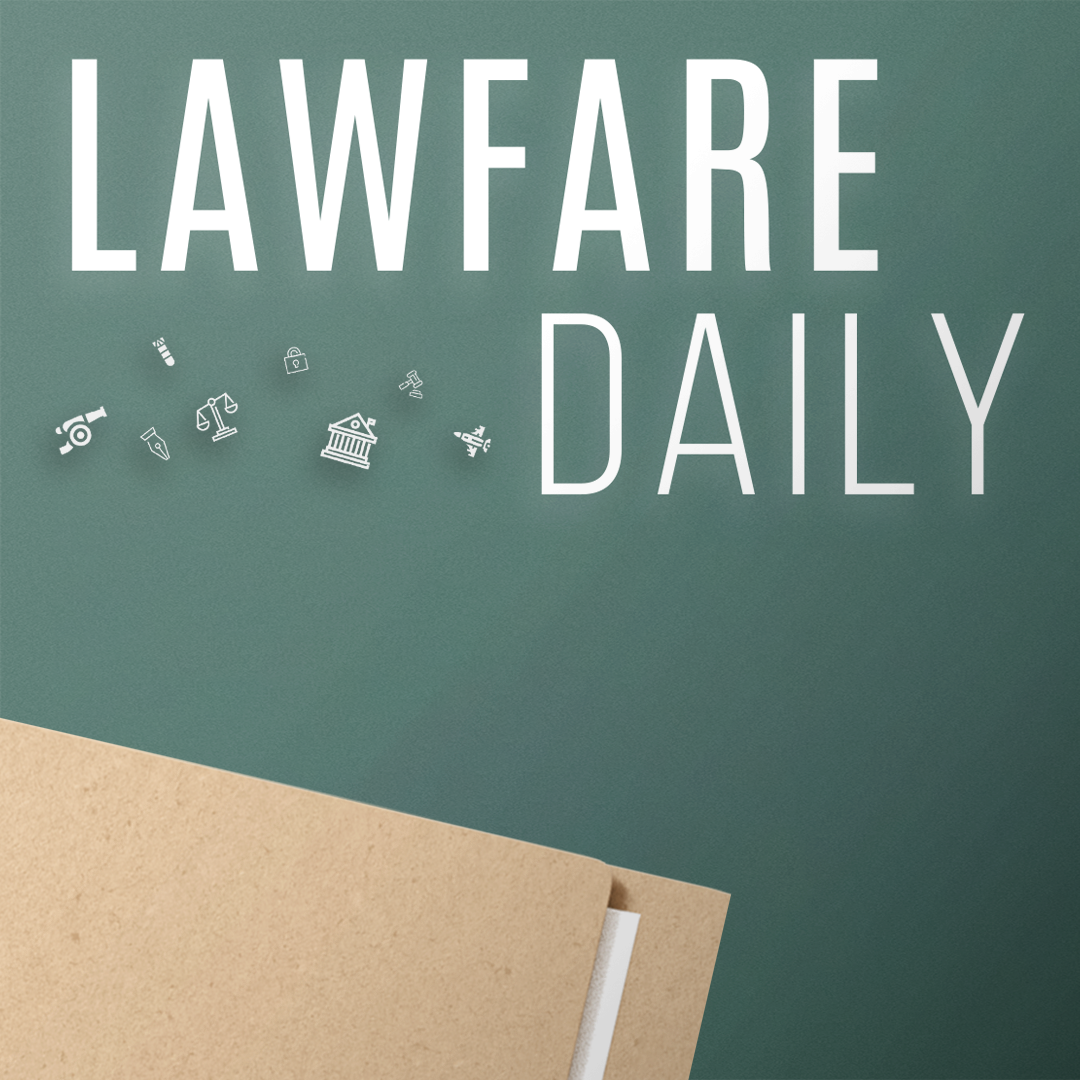
Published by The Lawfare Institute
in Cooperation With
Associate Professor at the University of Minnesota Law School and Lawfare Senior Editor Alan Rozenshtein sits down with Kevin Frazier, Assistant Professor of Law at St. Thomas University College of Law, Co-Director of the Center for Law and AI Risk, and a Tarbell Fellow at Lawfare. They discuss a new paper that Kevin has published as part of Lawfare’s ongoing Digital Social Contract paper series titled “Prioritizing International AI Research, Not Regulations.”
Frazier sheds light on the current state of AI regulation, noting that it's still in its early stages and is often under-theorized and under-enforced. He underscores the need for more targeted research to better understand the specific risks associated with AI models. Drawing parallels to risk research in the automobile industry, Frazier also explores the potential role of international institutions in consolidating expertise and establishing legitimacy in AI risk research and regulation.
To receive ad-free podcasts, become a Lawfare Material Supporter at www.patreon.com/lawfare. You can also support Lawfare by making a one-time donation at https://givebutter.com/c/
Click the button below to view a transcript of this podcast. Please note that the transcript was auto-generated and may contain errors.
Transcript
[Introduction]
Kevin Frazier: You need to get the order right. You need to do the robust research first, so that you can go to regulators with specific interventions that can then further be tested and refined through subsequent research.
Alan Rozenshtein: It's the Lawfare Podcast. I'm Alan Rozenshtein, Associate Professor at the University of Minnesota Law School and Senior Editor at Lawfare with Kevin Frazier, Assistant Professor of Law at St. Thomas University College of Law and co-director of the Center for Law and AI Risk.
Kevin Frazier: If we're able to consolidate both expertise and financial resources at an international level, well now it becomes far more likely that we're going to produce the quality and quantity of research that we really need to respond to the challenge at hand.
Alan Rozenshtein: Today we're talking about a new paper that Kevin has published as part of Lawfare's ongoing Digital Social Contract series, titled, Prioritizing International AI Research, Not Regulation.
[Main Podcast]
Kevin, let's start by getting a high altitude lay of the land. And I'd like you to talk about sort of three things and compare where they are. One is the development of AI systems, the systems themselves and their capabilities. The second is government attempts to regulate those systems for whatever reason. And then the third is the sort of research into the systems that we would need to do good regulation. Where are we on all three of those, especially as compared to each other?
Kevin Frazier: So, focusing on the first in terms of the AI capacity and development, I think we're pretty dang far along the spectrum in terms of where we thought we may be at this point in 2024. If you had asked anyone in 2022, before the release of ChatGPT, and you had told them about Llama 3.1, for example, I think people would have lost their pants or whatever undergarments they were wearing at that time.
So just to keep that in perspective, I think it's important to say, yes, I know we don't have ChatGPT-5. I know people were hoping for it yesterday and it still hasn't come and all that. But putting this into perspective, it is wildly impressive just how far we've come in a matter of two years. So on the development side, we continue to see pretty robust models being released at a relatively fast clip. How long that's going to continue into the future is to some subject to a lot of debate, right.
There's some people talking about a shortage of data. There's some people talking about a shortage of compute. And so for a lot of reasons, we could be hitting some degree of a slower innovation rate. But on the whole, I think folks should rightfully anticipate that we're going to see AI become a larger and larger part of our lives. In particular, because we're seeing this development of AI agents, AI models that will be able to act on your behalf without regularly prompting them to take certain actions. And so, as we see AI agents proliferate, for example, that's going to pose a, even broader set of regulatory issues and concerns. So that's the first bucket, is it may not be as fast as people thought, but we've still got a lot of regulatory headaches ahead, even if we see things slow down tremendously.
On the second bucket, when it comes to regulation, I'd say we're giving it a B effort. Right? So we see regulators around the world acknowledging that AI needs some degree of regulation. But for right now, I'd say a lot of that regulation is perhaps under theorized and minimally going to probably be under enforced. So let's start with the EU AI Act, for example. So the EU AI Act, which is coming online, is very much focused on setting AI systems into various risk buckets. And depending on the bucket, it's subject to additional regulation, which in theory will lead to more oversight and more enforcement. We've yet to see whether or not the EU has all the tools required to make sure that enforcement is robust, but I don't think anyone thinks the EU AI Act is going to meaningfully decrease AI risk across the board, whether you're concerned with algorithmic discrimination or something as broad as existential risks, such as the release of bioweapons. From what I've read, there are a few folks who are sleeping easier to the extent they are very concerned about either of those risks because of the EU AI act.
And on the U.S. context, I think maybe we'd, we'd instead go with a, a C. We know a lot of folks are concerned about AI. But if you just look at the past year, from Majority Leader Schumer saying things like, ah, we've really got to think about existential risk, catastrophic risk, mentioning things like a P(doom), inviting a lot of members of the AI safety community to the hill to most recently when he was releasing his bipartisan AI Senate working group roadmap in which he labeled innovation as the north star of AI, I think we can see the federal government is going through some wild pendulum swings about how, when, and whether to regulate AI. Generally at the state level, I think we see some increased attention to various risks posed by AI. The regulation that's catching the most headlines or the proposal that's catching the most headlines is Senate Bill 1047 in California, which wants to regulate models and prevent them from propagating critical harms across the U.S. and the world. So that's an important bill. Whether or not it's going to pass is very much an open question.
And given that kind of landscape. I think we can all say that given the risks we've acknowledged about AI, whether you're, again, focused more on those algorithmic discrimination type harms or existential harms, regulation isn't quite there yet, especially from a U.S. context. So we may have the seeds of meaningful regulation, but whether or not those seeds germinate into some robust regulatory regime is to be determined.
On the research side, and by research here, what I really want to focus on is this idea of risk research. Research that's focused not on identifying the best use cases for AI necessarily, research that's not necessarily benchmarking AI, but research that's really grounded in what are the tangible, specific harms presented by different AI models. With respect to that sort of risk research, we are woefully behind. So, I want to put this in context of some other examples of risk research that I'm talking about. But for now, I think it's important to see that when we talk about the level of investment going on with respect to this sort of research, the labs are spending billions, if not in a few years, trillions on compute data and expertise to understand AI and to advance AI.
Looking to the public side of the ledger, we see that the EU, the European commission is debating right now whether or not they should spend a hundred billion dollars over seven years to fund this kind of research. So this is very much a apples to, I don't know, an apple planet, right? Imagine just a planet imagine a planetary apple, right? It's just not going to equate. We've got a lot of resources on one side looking at how to really drive that AI development. And on the other side of the equation, the scale of resources required to do this sort of research is just not being meaningfully discussed at the public level.
Alan Rozenshtein: Why not just rely on the labs themselves to do as much research as they feel is necessary to do? I mean, presumably, there is some liability that they might experience that would cause them to want to do research in the way that a normal company that bakes a toaster wants to do research on if the toaster will explode because they might be held accountable. One might even think that some of these labs for kind of ideological reasons or philosophical reasons, however you want to call it, OpenAI, Anthropic, the people that are there might care a lot about these issues.
Why, to you, isn't it enough to just say, look they're, they're gonna do a bunch of research on capabilities but also on risks and, and like that's, that's, that's enough.
Kevin Frazier: So like all good lawyers and professors, the only thing I can turn to is analogies. And here the best analogy that I see is looking to risk research, as I've called it, in the development of the automobile industry. So if we look back to history of how did we treat the introduction of the automobile, think back to the Model T, Ford, all those good times, whatever, good vibes. Not a good vibe if you were the passenger of a Model T because for decades we just let cars drive all over the road without any sort of meaningful safety mechanisms within those cars. There were no airbags, there were no seatbelts, and so you had folks just going through windshields, folks getting punctured by different parts of the car upon a crash, and where was the risk research then? Did we just count on automobile manufacturers to share their results? They saw these crashes. They realized what was happening to drivers and passengers of these vehicles. But we didn't see that robust research going on, and then sharing that research with the requisite authorities.
Instead, we had to wait decades until Ralph Nader and the insurers raised attention about the harms caused by these vehicles and started to really study and thoroughly document what exactly were these risks. That's when insurers went to accident sites, looked at cars, looked at the exact dynamics of a crash, studied how the car bent and folded, studied where the people flew and started to thoroughly analyze what could be done to make these vehicles safer. And so I'd like to shorten that cycle between the introduction of the Model T and when we get the seatbelt in the 1970s and make that a much shorter time horizon in the context of AI. And so even though maybe OpenAI and Anthropic or pick your lab is a little more safety oriented than let's say Ford was, the profit motive for me will always be overriding with respect to these corporations. And that may change. And I'd love to be proven wrong, but I'm not going to bet my meager salary on that.
Alan Rozenshtein: Or the future of the world, if you're concerned-
Kevin Frazier: Or the future of the world.
Alan Rozenshtein: about existential high risk. Yeah, so I think the highway safety analogy is a great one, and you use it to great effect in your, in your paper to motivate the sort of thing you're talking about. And I just want to stay on this for a little bit because the history of it is really interesting and kind of under, underappreciated by a lot of folks. So just, just talk, talk me through a little bit of the history of the main institution here that, that did all of this research. This is the Insurance Institute for Highway Safety, and then also the National Highway Safety Traffic Administration. So how do you, how do, how do they come to be? And, and what, do you, were the, the main reasons why these institutions really were effective in, I think if Nader's if I remember the title of Nader's book, taking something that was unsafe at any speed and making wat are today actually remarkably safe vehicles?
Kevin Frazier: Yeah, so the Insurance Institute for Highway Safety or IIHS, it really rolls off the tongue, got started when they realized that a lot of insurance payments were being made to folks who were the subjects of horrible vehicle crashes. And if you're an insurer, ideally, you're not paying out those claims. And so their thought was, how can we help better understand who's responsible for these risks? Who's responsible for these harms that we're seeing in fatal crashes occurring across the country? And so a group of insurers got together and initially just developed data collection mechanisms. So it was all about, okay, we saw this crash happen at the intersection of 8th Street and B Avenue. What speed were the cars traveling? What kind of car was it? Where did the passengers go? They collected all this information. They consolidated all of this information, and then they began to share it even with other insurers. They realized that it was incredibly valuable for all of them to be sharing and collecting this information.
Then they went a step further, and they realized that they could begin to emulate these crashes in a practice setting, right? In a research facility, taking cars driving them into walls or driving them into different obstacles and analyzing whether or not that car was going to protect the driver and any passengers. And so it was this slow development of more and more robust mechanisms to understand what is it that these vehicles can actually do that help them see mechanisms for regulation, right? So, if you understand the risks posed by these cars, then you can go to policy makers and begin to say, this is an intervention that we've studied, that we've seen empirically repeated, in repeated instances that we think should be the focus of regulation. And here's a specific piece of regulation that we actually think would make a meaningful difference, and here's why. So that was the development of the IIHS.
In turn, we saw the development of the National Highway Safety Traffic Administration, and that administration more or less relied on IIHS to conduct Car crashes and safety testing, and then use those tests to come up with new standards and enforce those standards. So, what we see is what I like to call this research-regulation cycle. So you do the robust research, you share that with the requisite regulators, and then it results in some new policy, some new safety standard. And you repeat that cycle over and over and over again. But you need to get the order right. You need to do the robust research first so that you can go to regulators with specific interventions that can then further be tested and refined through subsequent research. So I think this cycle is really important to regulating emerging technologies, but you've got to get that order right.
Alan Rozenshtein: And in terms of the attributes that made in particular IIHS so successful, what are the main lessons you take away from that? And because if you know, if you're going to want to replicate this kind of institution, what do you want to make sure it has in?
Kevin Frazier: So I think when you look at risk research institutions, and you could put IIHS, and as I'm sure we'll discuss soon, CERN, and to some extent the IPCC in these categories, there are a couple attributes that really distinguish institutions that are able to do this risk research. The first is just expertise. So I was fortunate to be able to talk to some folks at IIHS and they are a small but mighty organization. They recruit some of the best safety engineers from around the U.S., and frankly around the world, and they consult with experts around the world as well to continuously refine and improve their crash test system.
The second is having close relationships with regulators. So, IIHS, because they've been in this game for so long, because they have established that robust expertise when they speak, they speak with authority. So they're able to go to the National Highway Safety Traffic Administration and say, look, we have this new finding. You all may want to issue a responsive regulation or policy statement or something of a similar effect. They speak with authority when they do that. And so we see this cycle start to advance because they have that research that is meaningful and robust, and then it's shared with the requisite regulators.
The other attributes of the IIHS and similar organizations is they're doing very transparent research. So you can go right now and see some of these crash tests. You can look at the exact specs of how they run their tests. What speed is the car going? What are the dimensions of the crash test dummies they're using? How are they thinking about the different configurations of these tests? And that way they're perfectly replicable by other organizations. And that sort of transparency heightens the authority of the organization even further.
Alan Rozenshtein: So, one of the big arguments you make in your paper is that not only do we need some sort of institution to do this research and regulation cycle or to get the research and regulation cycle going, but that it specifically should be an international organization. And so I want to spend some time talking about why you think that's important. Because of course it's hard enough to build anything, you know, domestic. You want to build an international version of it, it's a hundred times harder just because of the nature of international cooperation. So you clearly think that the benefits of an international institution outweigh those costs. And so just say, say why.
Kevin Frazier: So I will start with a preface that this is very much an idealized paper or a theoretical paper. If we were in a vacuum, this is how we should approach international risk research with respect to AI. And when we look at lessons of how risk research has been done in prior context, what's key is that you have all of the best experts available and that you have the affected parties feel like they are a part of that risk research.
So let's start with CERN as an example. So, CERN is a research entity based in Europe made up originally of just 11 European nations and those nations work together to ram particles together at incredibly high speeds to learn about the basics of the universe. Well, what's unique about their governance structure is they're allowed to have guest researchers, come and participate in those experiments. So, you have the leading particle physicists from around the world coming to CERN, to observe, inform, and improve whatever collisions they're doing in their incredible Hadron Collider. So that's a reason why we need that sort of international approach to begin with, with respect to AI.
AI expertise is not in the U.S. alone. There are AI experts around the world, and although they're probably disproportionately in the U.S., we have a higher proportion of AI expertise than perhaps other countries. The more we can bring those experts together, the more we're going to see robust results. So, in an ideal world, it would be excellent if U.S. AI researchers were working with those in the U.K., which in some instances they are, thanks to AI safety institutes, but also Chinese experts on AI. If we saw experts from Russia, from India, from you name the country, the more we can consolidate expertise in a single location or working on a single endeavor, the more robust results we're going to see because we're just bringing those different perspectives together.
The other thing that's really important about that international focus or reach of the risk research institution would be the legitimacy of its results. So right now, if the U.S. comes out with certain risk research, and it's only informed by U.S. experts, done in some opaque fashion, out of public view, and only with U.S. citizens, it's going to be really hard for people who perhaps are less, less risk averse and maybe for a myriad of reasons, skeptical of U.S. geopolitical priorities to accept that research. If instead we have an international body of experts participating in that process, well now it becomes far more likely that other countries are going to accept those results, act on those results, and further support that research going forward.
Alan Rozenshtein: I want to make this these points more concrete and to do that I want you to kind of specify what you think the benefits of the international research initiative would be to some proposals that have been made in the United States, actually for United States specific research. So, Senator Wiener has proposed some, some research facilities specifically in California. Stanford has proposed what it would call the national AI research resource. Obviously, the federal government has historically done a lot of basic scientific research in, in, you know, Brookhaven labs and sort of other places. You know, you, you talk about all of those in your paper and you sort of find them all wanting. And so I think it'd be helpful to articulate why specifically.
Kevin Frazier: Yeah, so let's just look at the instance of CalCompute, which is the proposal from Senator Wiener, which would involve predominantly working with California researchers, California research institutions, and state funds from California and any private donors who want to contribute to this CalCompute endeavor. And while I think it's really admirable to create something that will allow for public entities in California to do research as well as to explore different innovative uses of AI. The limitations are quite pronounced.
So separating some things into explicit buckets. First, if we look to that expertise category, we're going to see severe limitations of who is actually allowed to use that CalCompute, who can assist with that research. From my read of the law, it would generally be just California citizens, or in some cases, U.S. citizens who apply to use that resource. And that's somewhat understandable, right, if it's a California Research Institute, there's a reason why you would want to prioritize American and Californian users. That means we're missing out on a whole lot of perspectives. We're missing out on experts from around the world who could bring different insights to that research process. So, number one is just the, the shortcomings from an expertise point of view.
Number two is the insufficiency of resources. So CalCompute alone, assuming that California was able to muster, let's say a couple billion or even a tens of billions of dollars towards CalCompute. Again, that is just, it's an order of magnitude, if not two orders of magnitude, smaller than the resources that some of these private labs are going to be spending on compute. So the research that a single entity in California, or a single entity even in the U.S., would be able to procure is quite small. And as a result, the quality and the quantity of research coming from that institute would be quite small in contrast to the amount of research that should be going on. If we're able to consolidate both expertise and financial resources, at an international level, well now it becomes far more likely that we're going to produce the quality and quantity of research that we really need to respond to the challenge at hand.
Alan Rozenshtein: So, one of the international kind of models you look to is CERN, which we've talked about. But the other, and you argue actually perhaps more, more appropriate model for this issue is the, the IPCC, the Intergovernmental Panel on Climate Change. So what is the IPCC and what does it do and how is it different in terms of its role in research from something like CERN?
Kevin Frazier: So I want to say first, and not to brag, sorry listeners, I do feel a little bit validated by my favoring of the IPCC over CERN. For all those who aren't reading their European news on a regular basis, the president of the European Commission recently proposed a hundred-billion-dollar CERN for AI in Europe. This was in June. Weeks later, it's gone nowhere. No one knows what proposals she's looking at, what the specs are, who's going to be involved, where the money's coming from. So, although I would love a CERN for AI to be created, the odds of getting all of these countries who have repeatedly expressed a desire to be first in AI or the AI innovators to consolidate all those resources are just quite low.
And so what I think is a more feasible approach for at least some international risk research to be done is this IPCC. So the IPCC, as it works in its climate context, is an aggregation of the latest research on climate change from around the world. So, you get all of the research that's been done over about the past five to seven years. Then you get this incredible diversity of experts from around the world to come together, review all of that research, and share what is the current understanding of climate change. How have we changed our perspective or understanding on climate change since we issued our last IPCC report? So we've had numerous reports that often go on to inform some of these critical climate treaties and negotiations because the IPCC process requires that experts, when they're writing these reports, reach consensus on their findings.
And so this process, number one, gathering all the latest research. Number two, vetting that research with international expertise. And number three, reaching consensus on those findings as a result of that process can really shape the narrative as to what the right regulatory response will be for climate change going forward. And so the difference between a CERN for AI and an IPCC for AI is that the CERN for AI is essentially conducting the research. It's digging into the models. It's digging into different accident reports, for example, or what have you. It's generating research. The IPCC for AI, instead, is consolidating and verifying research that's done elsewhere. So, we might not have quite as robust of risk research done under an IPCC regime, but what we get is consensus about the risks that need regulation sooner rather than later and by specific actors. So that's the key distinguishing factor between the two.
Alan Rozenshtein: So I think a nice place to end our conversation is actually where you end the paper, which is a couple of lessons that, that you've drawn from your analysis and that you think it's important to get out there. So why don't we finish up with that? What, what are the big takeaways from you having, having done all this really interesting work on what a model for AI research and regulation cycle might look like?
Kevin Frazier: Yeah, so I think one of the first things that I just want to specify, perhaps at a more meta level, is there are a lot of suggestions going around about an IAEA for AI, a CERN for AI, an IPCC for AI, and while I think that's really good work and folks have the right motives, the crux of my research, I'd say here, is that we really need to be honest that international efforts at any level are, are quite difficult. And they're getting more difficult as a result of geopolitical tensions and the scale of research that's required in the AI context. And so what I want people to do is to just be a little bit more nuanced and humble when it comes to suggesting some of these organizations as models. Because when you dive into the weeds of a CERN or an IPCC, you begin to see that these are not just happy historical accidents. They didn't somehow emerge from the ether but are instead very carefully crafted institutions. And so that's really my first lesson is just that independent research isn't by accident. And so when you discuss the need for a research institution or a regulatory authority, be very specific about the sort of structure, culture, and governance that you want to see in that research body. And then ask yourself whether it's feasible to, to realize those aims in our current setting. And, unfortunately in some cases the answer is just going to be no. And if it is no, then you need to ask what needs to change to make that research feasible.
The second lesson is that a lot of this is just going to boil down to money. Unfortunately, resource intensive research requires resources. And so, we need to be really clear about where the money's coming from and how long it's going to be around. That's probably the biggest reason why CERN has become such an institution. It has reliable funding from a lot of different stable economies. And to the extent they're unstable, it has some nice fallback mechanisms to ensure that funding remains relatively stable. So thinking about that money question also has to be at the fore.
And finally, I would just say that we need global expertise on these questions. If we're not going to replicate the past and just have our international institutions reflect western values and global north perspectives, then we need to really bring other stakeholders to the table. And when you look at organizations like CERN, even though it is grounded in Europe, it brings in international expertise. And when you look at organizations like the IPCC, it doesn't settle for the perspective of experts from elite institutions in the West, but instead intentionally reaches a global set of expertise. And that's what we need with respect to AI, because the risks that are concerning Americans are definitely not the risks that are concerning other communities. And so we need to be attentive to that and shape our research and regulation to those global concerns.
Alan Rozenshtein: I think this is a good place to end things. Thank you, Kevin, for first writing this great paper and also then for coming on the podcast to talk about it.
Kevin Frazier: Always a pleasure, sir.
Alan Rozenshtein: The Lawfare Podcast is produced in cooperation with the Brookings Institution. You can get ad-free versions of this and other Lawfare podcasts by becoming a Lawfare material supporter through our website, lawfaremedia.org/support. You'll also get access to special events and other content available only to our supporters.
Please rate and review us wherever you get your podcasts. Look out for our other podcasts, including Rational Security, Chatter, Allies, and the Aftermath, our latest Lawfare Presents podcast on the government's response to January 6th. Check out our written work at lawfaremedia.org. The podcast is edited by Jen Patja and your audio engineer for this episode was Noam Osband of Goat Rodeo. Our theme song is from Alibi Music. As always, thank you for listening.