Lawfare Daily: The Antitrust Implications of AI Systems with David Lawrence
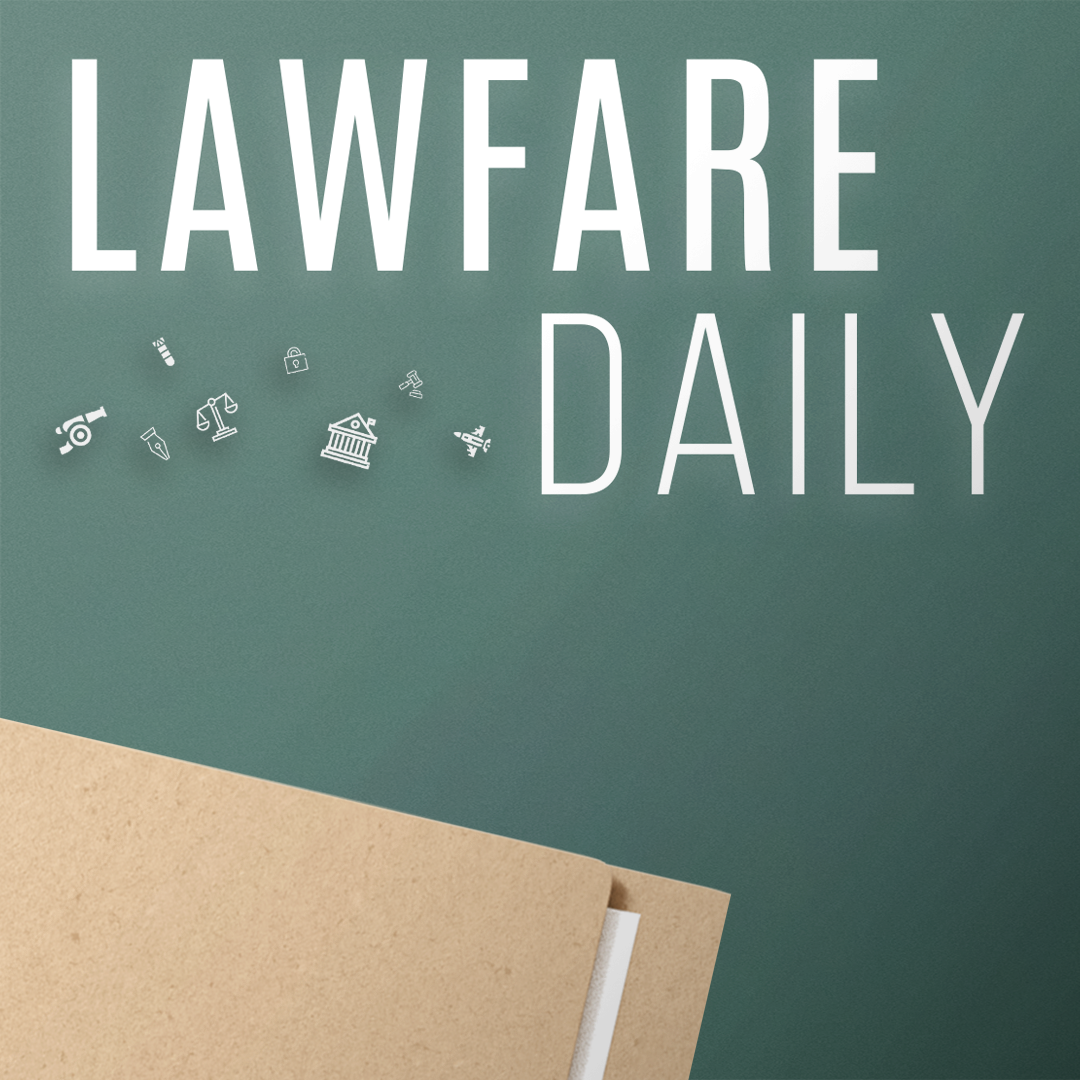
Published by The Lawfare Institute
in Cooperation With
At a recent conference co-hosted by Lawfare and the Georgetown Institute for Law and Technology, Lawfare Senior Editor Alan Rozenshtein sat down with David Lawrence, the Policy Director at the the Department of Justice's Antitrust Division, to talk about how competition law applies to the makers and users of AI models.
Click the button below to view a transcript of this podcast. Please note that the transcript was auto-generated and may contain errors.
Transcript
[Intro]
David Lawrence: So if the most important thing in competition is that we encourage dynamism and innovation, that the market's able to move quickly and people are able to get involved, open source has some attractive characteristics.
Alan Rozenshtein: It's the Lawfare Podcast. I'm Alan Rozenshtein, Associate Professor at the University of Minnesota Law School and Senior Editor at Lawfare, speaking with David Lawrence, Policy Director at the Antitrust Division of the United States Department of Justice.
David Lawrence: I absolutely reject the premise that you need monopolists to drive innovation. Innovators thrive on competition, the availability to get market access, the ability to get new partners, and to go out there and compete. So, I think that's something we really need to support.
Alan Rozenshtein: Today, we're bringing you a conversation about AI and competition law that I recorded with David at a recent conference on AI liability that Lawfare co-hosted with the Georgetown Institute for Law and Technology.
David Lawrence: Two disclaimers I have to start with: first, I'm speaking in my personal capacity. My views don't necessarily reflect those of the Department of Justice Antitrust Division. Second, the term AI can be really broadly defined. And so just like give me that breadth today, I'll be using it as a general term encompassing everything from advanced algorithmic software, machine learning, up through generative AI and LLMs.
And also, I do want to just thank the organizers again for hosting what I think is a critical conversation at a time like this. Academic engagement with the policy community, the academic role of building the blocks of knowledge is so critical to making decisions and we're in a time when I think regulators and enforcers and courts are going to be making more and more decisions.
I think of this a lot like the advent of the automobile. I'm so glad Professor Sharkey mentioned the automobile example. It's really exciting to see this new technology and what it can do. We can hardly imagine how it will change our world. Like I think people just thought it was going to be faster horses. That's kind of what we're thinking now. But we're also just imagining some of the risks.
I also think, like, we should acknowledge, like, out there in the world today, we accept a great deal of harm from automobiles. But it did also take us many years to develop regulatory protocols to minimize that harm to where it is now. So, I actually want to set the table, and don't peek at my notes here, with a trivia question for my moderator here.
Alan Rozenshtein: God-
David Lawrence: Do you know when the Model T was released?
Alan Rozenshtein: 1910s?
David Lawrence: You're so close. October 1st, 1908. Okay, another trivia question: what year did Henry Ford get his first driver's license?
Alan Rozenshtein: You're killin me here. Henry, oh, well, I assume there weren't driver's licenses for a long time, so I'm gonna say I don't know, 1942.
David Lawrence: So we actually, it was fairly quick in Michigan. In 1919, they started issuing driver's licenses. They didn't have a test, though.
Alan Rozenshtein: Did you self-certify?
David Lawrence: You just asked for a driver's license. And, you know, of course he didn't live to see the seatbelt laws, which don't come around until 1968.
Now, we don't know whether AI will wind up with license requirements for models, what an AI seatbelt is going to look like, but I do hope and I think these kinds of conversations foster that we could be a bit faster out of the gate in predicting and preventing the harms even as we make sure we harness the benefits of the new technology. And so conversations like this are really on the critical path to achieving that. Happy to be here, eager to hear your questions.
Alan Rozenshtein: Excellent. Thank you. So, let's start sort of a big picture question: what is the evidence that AI can be used to facilitate collusion? And do you think the law and specifically sort of competition laws capable of handling collusion by non-humans?
And maybe also just as part of that, maybe say just a little bit for those in the audience who are maybe not super familiar with competition law and these kind of foundational concepts, you know, can you give us a thirty-second overview of competition law, as you answer this very other complicated question?
David Lawrence: So-
Alan Rozenshtein: I gotta get you back for these trivia questions you asked me.
David Lawrence: Yes, I appreciate that. It's a great question. It's a really big question. I'll try to weave in some of the competition law concepts. When you say AI being capable of handling collusion, there's really three kinds of collusion, roughly, that the antitrust laws are often concerned about. So, you have price fixing agreements between companies, and then you have other forms of agreements that aren't quite as bad, like maybe a rule of reason information sharing, which can be problematic. And then you have the kind of collusion that happens in an oligopoly, where the competitors, they don't actually talk to each other, but prices kind of float up because they're like looking at each other and there's only a few of them.
So let me just take those one at a time. The first type of collusion, as I mentioned, is this very direct price fixing. And the antitrust laws don't like this because you're fundamentally undermining the competitive process, if two companies that purport to be competing with one another are agreeing in a smoke filled room as to the prices they're gonna charge. We treat those as criminal antitrust violations.
And in terms of evidence, we actually at the division, first came across an algorithmic price fixing case back in 2015. It's the Topkins case. So, this case involves sellers in online marketplaces. So, you all are probably familiar, when you search for a product in an online marketplace, you get like a bunch of identical products from different sellers with like slightly different prices. None of which end in a five or a zero. That's because the sellers are using algorithms behind the scenes, collecting data, and trying to sort of maximize their own position by adjusting the prices oftentimes in real time.
Well, back in 2014, sort of early days of this stuff, a couple of sellers of art posters on a major website noticed that their art posters were like coming up right after each other in the auction or in the results a lot, and so they talked to each other. And they agreed to rewrite the code of their algorithms so that they wouldn't bid against each other, which of course is price fixing, raises the prices, and so we pursued that case criminally, and the defendant pled guilty.
Now, an issue we may soon confront as AI gains capability, is what do we do if the humans weren't involved and the AIs had that idea to communicate with each other and reach an agreement? Our answer has been, and I think the answer under the law is, that if a company is delegating this price decision, price setting authority to a computer system, it needs to ensure that computer system complies with the same laws a pricing manager would.
The Supreme Court's test for what constitutes an agreement between firms in this antitrust context, it's not like contract law, offer acceptance. It’s, the phrase is concerted action and the language is actually really helpful here. So, there's a case that relates to the NFL, American Needle, and the Supreme Court said that the law is triggered by conduct that, quote, deprives the marketplace of independent centers of decision making that competition assumes and demands.
And I love that line, independent centers of decision making. And I think it is readily adaptable to the circumstance where a company delegates its decision making to a computer system, if and when that happens. You know, I think about it like this. If a regional vice president of pricing in a company, somewhere six tiers down the org chart, is delegated authority to set prices in their region, the company needs to train them up on the antitrust laws and make sure they're not talking to their competitors. Because if they do, the law will treat the entire corporation as liable for the actions of that agent.
I think by the same token, if a company delegates to an AI to be the center of decision-making on pricing, to be its pricing agent, the company needs to make sure it's training that algorithm not to cross the line into communicating and agreeing with its competitors.
Alan Rozenshtein: Yeah, so that's interesting. So, it sounds like then to think through the antitrust issue here, you have to think through the agency law issue as well. Right? And I mean, because presumably there's going to be some agent, like AI agents. This is another thing we wanted to have a paper on, but we can only have so many papers, unfortunately. Right? So there's truly some work to be done to develop what a agency law of AI is.
Like, when does your AI, I forget what the funny technical term is, go on a frolic and fancy or something. Someone call out if you've, if you know what the actual term-
Audience Member 1: Frolic and detour!
Alan Rozenshtein: Yeah, frolic and detour, thank you, right? And presumably, you know, you could say, right, I have this very complicated AI, and I tried to train it, but you know these AIs, and, you know, they went on a frolic and detour, like, what do you want from me!?
David Lawrence: So, yeah, absolutely. I think you're thinking in the right space. I would splice it a little thinner that for antitrust law purposes, it's just doctrinally separate from agency law.
Alan Rozenshtein: Okay.
David Lawrence: And it's this concept of concerted action, what qualifies as a combination or conspiracy under the Sherman Act, Section 1. So that's the first type.
Alan Rozenshtein: So, okay, so we have price fixing. I think this information sharing was next.
David Lawrence: Yeah, so information sharing. This is a second type of collusion where competitors, they aren't agreeing on the prices they're going to charge, but they're sharing a lot of competitively sensitive information with each other.
And for many, many years, the Supreme Court has recognized this can be very problematic. It's subject to an analysis where you balance the harms against the benefits, but it oftentimes illegal for companies to be, like, you think about two rivals like calling each other up and being like hey just so you know next week I'm gonna start charging this price. Like, that's a very suspect, oftentimes competitive behavior.
And one thing that's interesting about this in determining when that's problematic, historically the courts and had looked at like how much the information has been aggregated. Like, if it's specific individual competitor information, well that's really problematic, but if it's aggregated, who could ever tease out what's going on in the market or what particular competitors are doing from that information, sort of a factual now. You know who can tease it out? AI, right? And so the nature, the economic nature of what competitors can do with information exchanges is changing. And so, as we are undertaking our analysis, as we are presenting evidence to the courts, the analysis needs to just keep all of that in mind and keep the market realities in mind.
And we have a recent case, you know, talking about evidence that's in this vein, or a related vein, which is a lawsuit we filed just a few weeks ago against a company called RealPage. And I want to respect the court, so I won't go beyond the pleadings, but the basic allegation in RealPage is that the software company sells a software, advertises a service to landlords where the landlords provide their confidential pricing and apartment information to this software company. The software company runs an algorithm and then it tells the landlords how much to charge for each unit in real time. And it's doing this for, as alleged in our complaint, competing landlords. And we allege that this raises prices for rent and, you know, creates a problem. And I won't go beyond the case further than that, but you could look to the, we have a fairly lengthy complaint. You could look at some of the details.
Alan Rozenshtein: Yeah I will say because I can go further. Does RealPage not have lawyers? I mean, that sounds really bad, but fair enough. The third example he calls oligopolistic collusion.
David Lawrence: Yes. So, this is more than an oligopoly behavior. They actually have even more technical terms for it in anti-trust: parallel accommodating conduct and tacit coordination.
But what that is when there's two gas stations across the street from each other. The owner of one doesn't have to walk across the street and talk to the other one for the two of them to look at each other's signs and kind of nudge the price up. And vice versa, right? If both of them are charging 3.50 a gallon, one guy on one side of the street knows if he goes and puts it down to 3.40, the guy across the street's probably gonna do it too. But, if you're at an intersection with like 12 gas stations, that doesn't happen, right? So that's what we call an oligopoly, a market where it's fairly concentrated. There's not a lot of different competitors, and they're able to engage in this kind of coordination.
Now, the reason I use the gas station example, really easy to see each other's prices. So historically, in our merger law, right, when we were looking at what the risk if two companies are merging, the risk that it's going to result in some of this oligopoly coordination, one thing we'd look to is how transparent are the prices? How easy is it to see and adjust your pricing? Like, how much is it like the gas station example?
This is another area that AI is changing, because it's fairly common now for companies to have AI systems that are just actively determining and using the web all of their competitors’ prices. And so, you’re able to have that oligopoly pricing problem with relatively more competitors in a market.
And so one way we've reacted to this, this is something that last year we revised our merger guidelines. We have a guidance document about how we review mergers, have revised it every 10 or 15 years, put it out for public comment, and a lot of people raised this concern. So, we added some discussion in the section on this issue, that if the market, if people are using algorithmic pricing tools that we're going to be more concerned about oligopoly coordination.
Alan Rozenshtein: So, we've talked so far then about how AI tools themselves can be used to potentially cause, maybe intentionally, even maybe unintentionally, right, because you can imagine, you know, in good faith, I made this algorithmic pricing tool and the AI just was smarter than me, right, and you have to figure out how to deal with that liability.
David Lawrence: Yeah, so just to go back to my example, in good faith, I hired a sharp regional manager for Texas. But I forgot to train him not to talk to his competitors and it's actually really profitable to talk to your competitors, so if you don't train people not to do it, they're gonna.
Alan Rozenshtein: Yeah, but I want to move now to the question of potential anti-competitive issues within the AI industry itself. So, one thing that's just really remarkable about especially sort of the frontier of machine learning is how concentrated it is because compute scale and data scale is so important in that, you know, it's just hard to make progress unless you have a market capitalization of 100 billion dollars and there's aren't that many companies that do this.
So given that concentration, how do you think about the potential antitrust problems, right, generally? And then speaking of mergers, when it comes to the acquisition of, let's say, AI startups by the large incumbent players. We've seen a lot of that as, you know, a lot of the really promising AI startups that popped up in the last couple of years, you know, they get out of the gate, they make a really impressive product, and then they want to scale to that next level. And they just don't have the money, they don't have the cloud compute, so, you know, they end up being bought by Microsoft or Google or Meta or something like that.
David Lawrence: So let me, sort of weaving in the Antitrust 101 pieces here, let me start with why the benefits of competition are so important in this industry. So this is 101, but competition is the foundation of our economy. It's how it works. We have a capitalist democracy, and the way that works is when rivals compete, whether that be in foundation models or chips or app, they deliver people that they do business with more of what those people want and less of what those people don't want.
So, my boss, Assistant Attorney General Kantor, he likes to say that competition is beautifully democratic in that way. I very much agree with that. When we talk about being a capitalist democracy, it's because those two concepts are kindred spirits, right? The people have the choice in our economy.
In contrast, when dominant firms control markets and engage in exclusionary behavior, markets become less responsive to the things that people want. And the exclusionary behavior can stifle innovation and entrepreneurship. So, if there's a dominant gatekeeper keeping small firms out of the market, that's one of the things we're focused on in the monopolization case, you're less likely to invest in a startup in the first place. Because you perceive that threat and you know you're not going to be able to enter, right? And that competitive dynamic is so important for much more than the price of gasoline or other services.
Because, you know, AI is a great example of this: I don't know, like sitting here in D.C., as a bureaucrat, what consumers will want to see in their AI products. I can guess they will want them to be safe, secure, accurate. Those things we think about, regulation may be addressing. But the very best regulator to ensure that people are getting what they want is going to be that competitive process out there in the free market, driving companies to respond to consumer demands for safety and quality of products.
And just to go back to my automobile example, there are many lives saved every year because of competition between auto manufacturers over and above the regulatory floor. You think about differentiated companies like a Volvo that are like advertising the safety, right? But we need competition to have that dynamic playing out in this ecosystem.
And there's also, I think, in AI and in a lot of industries, areas where there are market failures. And you find regulation necessary beyond relying on the competitive process. Experience has proven that the more competitive markets with multiple firms are easier and more effective to regulate because monopolists tend to capture and sort of pretty readily defy the will of their regulators. We went through this with AT&T when it has a, had a telephone monopoly throughout the United States.
Okay, so that didn't answer your question, but that's sort of the backdrop. It's an important backdrop because you know, when we look at dominant players in any ecosystem, we are very focused on ensuring they don't leverage the dominance they have today to exclude competition tomorrow.
And you see that principle in a number, we now have a number of monopolization cases pending, several of them in the Big Tech space. There's a case in federal court right now against Google. We just won a monopolization case against Google related to search. We have cases against Visa, Ticketmaster, and Apple now. And you'll sort of see across those, a concern with how dominant firms have an incentive to exclude competition, and build moats around their castles. But when they do that, they frustrate the competitive process, they make the markets less dynamic, and that's problematic in the long run. So that's what we're looking for and looking to try to prevent.
Alan Rozenshtein: Relatedly to this concern about monopolization, because of the need to scale compute, is this question of data, right? So data is, and the after compute or with compute, is sort of the most important resource here. And so how do you view data as sort of a special part of the antitrust analysis here, you know, whether it's data accumulation or data sharing?
David Lawrence: So I think this really just, it goes back to what I was just saying, and I'm going to keep hitting the gas stations for you. It doesn't matter how many gas stations are on the street. If one of them bought like all of the gasoline in the world. They would be able to just shut down all the competition locally, right?
And so one thing we're looking for in markets, and I'm not in a position to say, it's a fact inquiry, like how data exactly will play in specific AI cases, but what we'll be looking for is the extent to which it's an important input to competition among all the rivals, and then whether and how it's being used or abused to exclude competition in the way that we think is problematic.
Alan Rozenshtein: So, another aspect of potentially anti-competitive conduct is vertical integration, right? So, you have horizontal integration, right? You have, there's only one gas station, right? Or someone controls all the gas stations in an area, but then you have vertical integration, and they control the gas station, and they control the refinery, and they control the oil well, etc., etc.
We're beginning to potentially see some of that in AI, right, whether it's NVIDIA, which obviously makes all of, you know, most of the high end chips, maybe releasing its own models. Sam Altman, right, head of OpenAI, going around at least reporting suggests and trying to, you know, raise literally trillions of dollars for chips. Honestly, you know, with the news that Microsoft is going to buy all the energy from the Three Mile Island reactor, maybe these companies are also going to become fusion companies as well to power their stuff.
So, you're seeing potential for vertical integration. You know, is, does that, you know, again, without commenting on any sort of specific situation, does that raise its own special set of potential antitrust concerns, right? Apart entirely from, kind of, the horizontal integration.
David Lawrence: So the analysis is different from the horizontal integration analysis. I'll be careful to just stay away from any particular firms. Our merger guidelines, we just updated, talk about this. And I actually, you know, I'm going to start with the example you gave, is a company using its own R&D and investment building a vertically related product or service, right?
So the gas station owner, like, bought some land down the street and built a refinery. And what our merger guidelines say is that tends not to be problematic. We want companies to invest and innovate, right? There's a different analysis if it is purchased through acquisition, as opposed to investment. And that's where, I alluded to this earlier, then you're going to analyze whether that merger, you know, it may have some benefits related to the vertical integration. But there's also potentially relationships with your rivals that the company you're buying already has. And that's where we're going to look for sort of the competitive effects.
And I'd contrast that again, if a company is just building its own new product or service, we're not going to be concerned that rivals are like reliant on that product or service. It didn't exist yesterday. Right? On the other hand, if you're buying a product or service, the phrasing in the merger guidelines is products your rivals use to compete. So if yesterday they're using that refinery, now you're buying it through acquisition, now we're going to need to worry about whether the merger is going to harm competition in that way.
And then a similar analysis can sort of play out with contracts. So, you know, it's not uncommon for there to be vertical contracts that are locking up key inputs or distribution channels.
I think I mentioned a minute ago this Google search case. So, we just won a liability judgment in DDC where the court agreed with the United States that Google is a monopolist in search, and that one of the ways it maintained this monopoly was by paying a bunch of phone manufacturers so that it has the default status on the phone. So, I think one of the one of the findings of the court was the payment to Apple from Google to be the sort of lead search is in many millions of dollars, right? And in those situations, the competitive concern arises that you're limiting opportunities for competitors to maintain your dominance.
Alan Rozenshtein: One of the major, I don't know, fault lines is the right word, but one of the major kind of buckets of the big frontier models is whether they're closed or open, right? And there are lots of definitions of what this means, right? But let's say kind of a first approximation. You have models like the GPT family of models of OpenAI, the Claude models from Anthropic, Gemini models from Google. They're closed in the sense that we don't know the data that went into them. We don't know the algorithms. We don't know the weights and biases, right, the specific parameters of all this stuff.
And then you have, on the other hand, open-source model. You have a bunch of them. Obviously, the most important one probably is Meta's Llama model, which is always like, you know, two months behind the frontier, but sort of pretty clear that, at this point, you know, you can have open source models that are basically as as powerful. And I think maybe Meta, I think earlier this week, even released some updates to that.
So, I guess my intuition would be that open-source models present fewer antitrust concerns, but at the same time, who knows why, and again, abstracting from the specific case, right? But you know, who knows why a company like Meta, right, would do an open-source model? What's in it for them? So maybe open-source models aren't better for antitrust. I find myself sort of quickly getting into knots about this open source. You know, I like open source for my own ideological reasons, so I want open source to always be the answer. But I'm curious what you think about how the opensourceness of a model would play into the analysis of competition.
Now of course, I should also clarify, if you use the open-source model, to your self, it doesn't matter, right? You included the closed source, open source, to help you. When you're thinking about competitive, anti-competitive issues within the AI industry itself, you know, is open source better?
David Lawrence: So, you know, I want to caution, our cases are fact specific, and you get into the details on each one. But maybe I'll mention, you know, some features of open source that are attractive from a competition standpoint. So, if the most important thing in competition is that we encourage dynamism and innovation, that the market's able to move quickly and people are able to get involved, open source has some attractive characteristics.
You know, one thing I'll mention, this is an economic concept, but a lot of these markets are characterized by scale economies and network effects. And there's probably some aspects of the AI stack, some important ones, where we'll see those economic features. You know, network effects is like, the larger the network gets, the better it is for every participant.You see this in like, apps and things like this.
Network effects can play out on a firm level, or they can play out on a market level. Alright, think about the network effects in email. Right? Lots of different providers, but we have market level network effects because you have interoperability, and it's just an open connection between email, and the web could have developed differently. Like, we could have seen somebody shut the walls of email and just taken it over, because they deprive rivals of the network effects.
So open source is a great model to try to capture some of those broader network effects. I also think that open source, it can be attractive for some of the other features that a lot of folks are focused on around the development of AI, especially safety and security. Because open source, we had a conference a few months ago. We went out to Stanford and co-hosted with them a sort of AI workshop. And the terrific thing about that is we got VCs and founders who would never fly to Washington D.C. to talk to us, to like, take the quick cab in and talk to us for 45 minutes, right.
And we actually heard from a couple of people who were developing, they're not developing models themselves, but they're hoping to be the player, who's the third party who helps facilitate in an open source ecosystem, safety and security for the various players in that system, right? And so, the same thing in the academic community: the more open these models are, the more capable academics are going to be to get in there, look for vulnerabilities, and try to expose them.
Now, of course, you then get, you do get into the, on the safety and security side, like, who are the users, right? And so, one of the conversations that's playing out up there, and to get back to my trivia question, how long did it take Henry Ford to get a driver's license? Is, you could see, and I think the conversation will play out, over whether open source, when it's significantly powerful models, sufficiently powerful weights, whatever it might be, that there's a sort of licensing requirement for those developers who access it, and then maybe the apps the users develop don't need those licenses. You know, far be it for me to say, but it's a conversation that I think it's gonna be really interesting to watch play out.
Alan Rozenshtein: So, since you mentioned sort of the internet and you mentioned the Model T trivia questions. But let me ask about sort of the history here a little bit: are there any lessons from past technological revolutions that you're thinking about, when DOJ is thinking about generally, when trying to apply the antitrust law to AI?
Let me give you a more concrete example. So, the internet was a big deal and it developed, and obviously it's not as if antitrust cases weren't brought. I mean the big Microsoft case was specifically about Internet Explorer and all that sort of stuff. But there, I think, is a perception, and I think it's reasonably fair, that it was pretty light-touch. If you look, sort of, in aggregate of the government's regulation of the internet, whether through antitrust or other ways, it was pretty light touch.
Now, you can think that was a really good thing, or you can think that's a really bad thing, right? But putting that aside, I'm curious if, you know, how you're thinking about whether it should be, or will be, or can be similarly light touch when it comes to AI or to, you know, going back to your kind of trivia questions, whether you think it would be a bad thing if we just let this develop in its own way for a decade or two and then see where we are.
David Lawrence: I think that one of the lessons of the evolution of digital markets, where I think it was fairly light-touch early on. It was light-touch early on in the hopes that competitive forces would come in and bring new competition to some of the dominant players as they were emerging. And, you know, I mentioned the network effects. There's some really powerful economic principles at play in some of these markets that proved us wrong. And that led dominant firms to be able to maintain their dominance, and we saw some durableness to it.
And so, I do think that one of the takeaways from that, and you hear this from law enforcers, from state attorneys general, is that doesn't mean we should just go bring cases in any particular area, but there had been a philosophy in the past that, oh, we should just sort of intentionally stand by, even if the law is technically violated. And I think the philosophy now is much more, we should fairly examine the facts, examine the law, and bring those cases without fear or favor whenever they appear.
Alan Rozenshtein: And in doing that, how do you think about balancing the need to enforce the, you know, competition laws with the desire to keep this industry innovating, right? Because, you know, you could imagine, you know, folks in the industry being worried about what they would view as over aggressive antitrust enforcement because they might say, hey, look, this is a unique market in which scale is everything. Like it's all about scale. So, you know, we appreciate the competition concerns, but like, you just can't build GPT-7 unless you have a market cap of trillions of dollars. And that means they're going to be only very few of them these companies.
Or, you know, you we need to make it very easy for these companies to buy startups, because that's just only going to be the way that, you know, a startup comes up with a good idea. Then they need to scale that up, right? The only way that's going to happen is if Meta buys them. So, if you guys are really stingy, right, let's say with your merger acquisition rules, you know, that's great: we're going to have a very competitive market of not great models, right? So how do you balance that?
David Lawrence: So maybe I'll just-
Alan Rozenshtein: And maybe it's a false dichotomy, right? So feel free to push back against the framing.
David Lawrence: Quickly push back against the last framing. So if you're an entrepreneur looking for exit, and you have multiple billion dollar valuations, There's going to be more than just firms that already have dominant monopoly power in a local market who are interested in buying you, for the most part, right? And maybe that second place bid, because the company doesn't have monopoly power to preserve, is 10 percent lower. But you're going to continue to see, I think, those exits taking place.
Maybe going back to your broader question, historical examples, I think, are really useful in times like this. And so, I have a paper in the Network Law Review focusing on the development of the telecommunications industry and the sort of interactions there and what we can learn. And one great, great example is the AT&T breakup in the early 1980s, which I think ushered in enormous innovation in and around telephony, both long distance, and then the rise of the cellular networks.
I wanna ask you a trivia question about the first cell phone. The first cell phone was the Carter phone. It was this rancher, his name was Carter, and he, not Jimmy, and he wanted to be able to be out on his ranch and still take phone calls. And so, he essentially, this is like in the ‘60s, he essentially strapped like a walkie-talkie to his phone at home and then he like did some ‘60s era circuitry and he made this device so he could be out on the ranch, right?
And it was really a great invention, right? Like cell phones are super good. So, he wanted to bring it to market. AT&T had rules. And AT&T had rules that nobody could make a device that attaches to the AT&T network without AT&T's approval. So, they shut that thing down and, you know, he litigated, he went to the FCC and it took, I think, 10 or 15 years for a government order to say like, oh, AT&T, you have to let the Carter phone happen.
Meanwhile, 1983, the government breaks up the AT&T monopoly. You wind up with local telephone companies and this is key: each of those local telephone companies winds up building its own cellular network and you have multiple cellular competitors in the United States. And the guy who, like, more brilliant, probably, than Carter, Steve Jobs, comes up with an even new great type of cellphone, right, the iPhone, and we all know that origin story. He, like, talked to cellphone men, cell phone companies.
And AT&T, no longer a monopolist, but facing competition from three other cell phone providers in the United States, gave him, like, one of the biggest sweetheart deals ever given to a phone manufacturer. Rebuilt parts of its network, remade the data plans so that it could advertise, compete, by saying, come to our network, you can get the iPhone, right? What a beautiful innovation story.
And so, I bring up that historical example because I absolutely reject the premise that you need monopolists to drive innovation. Innovators thrive on competition, the availability to get market access, the ability to get new partners and to go out there and compete. And so, I think that's something we really need to support.
Alan Rozenshtein: So, so far we've largely. It's in the domestic context, which makes sense right? That's, you know, competition law is mostly domestic. Most of what you do is domestic, but not in all of what you do is domestic, and you are the head, in addition, of policy and appellate, the international side of the House.
And so I'm just curious, how much of this is an international story? It is true that, most of the frontier labs are in the United States, but certainly not all of them are. Mistral is kind of the French lab and they're very close behind. Obviously, you have a lot of stuff happening in China. It's a little delayed because of export restrictions and stuff like that. And then of course even the U.S. labs have their international outposts, a lot of the Google stuff happens in England. So, I'm just curious, you know, to what extent is there an international component, and what is it, and sort of how do you think about that for AI antitrust?
David Lawrence: Sure. Great question. There, I think there's a significant international component to the policy dialogue around AI. And just for background, international antitrust enforcers, like international enforcers who have like overlapping jurisdiction in a lot of areas, get together for policy discussions, like next week the G7 competition summit is happening. So, the senior competition folks from the G7 countries will get together.
The two days of that summit are focused on AI. And it's in part just because everybody's doing what we're doing at this conference, which is trying to learn and get smart. And you know, as a policy worker in an enforcement agency, and I think you know this, you have policy experience, you want to be learning from every, like you're reading the academic articles, you're reading the white paper articles.
Other law enforcers who are thinking really hard about it have a uniquely valuable perspective. There's something so useful about, just like, stepping out of your own day to day and you have a different country but some of the same issues interacting. And just being able to, like, think about it from another angle, like, you come back home and I think you, you approach it a little bit smarter.
Alan Rozenshtein: So I want to finish, I'm going to ask the last couple of questions before we go to Q&A so folks should just start thinking about their questions.
David Lawrence: Do you have trivia for me?
Alan Rozenshtein: I don't. I'll leave it to the audience. I want to end by asking a couple of questions about the more nuts and bolts of DOJ's role here and sort of the capacity that you have to do this work. And one question is as you're thinking about these, as you're bringing cases, do you feel like you have the legal tools to do the kind of work that you need to do here. So, this is a law and tech conference, so I am legally and contractually obligated to mention the Frank, Judge Frank Easterbrook's Law of the Horse article from the mid-1990s, right?
This is this question that is sort of ever present in tech law circles about whether this is all completely new and we need to, like, utterly come up with new legal concepts and legal rules and statutes and stuff like this for some new technology. Or if this is interesting, right, but we have background principles of whether it's products liability or negligence or IP or antitrust that are perfectly good here and just like you wouldn't have a law of the horse, right, you just have law of normal stuff that you apply to horses.
And so you might say, look, we have the Sherman antitrust law, right? We have these statutes. It's kind of nice that they're incredibly short because we can interpret them capaciously. And that's fine for AI. Or you might think: we just need new tools, right? We need to go to Congress and we need new statutes because there's some unique challenges here. So I'm just kind of curious if you could riff on that that idea.
David Lawrence: Sure. I would distinguish maybe between the tool, whether the tools in the law are important and whether they're sufficient. So, you know, I think maybe this conversation is underscored, and this is my day job, there are very important respects in which the existing antitrust laws engage with the emerging AI ecosystem.
And it's really important, and case-specific adjudication in the existing cases are going to engage importantly. And we just need to take those one at a time. There's enough to do there for me that I don't have to sort of take on the question that we leave to Congress of, are they sufficient, or we leave to state legislators, are there gaps beyond that in the law? I kind of focus on the law enforcement day jobs, so I don't have to worry about it.
Alan Rozenshtein: Fair enough. A big part of bringing these cases is having the technical expertise to know what is going on with these models. And that technical expertise is very hard to come by. I mean, it's hard enough for the frontier labs to hire, you know, machine learning engineers. I imagine for a number of reasons, and this is a kind of a standard problem in government, hiring really good subject matter experts in technology is difficult. What is antitrust's needs in having this kind of in house expertise, and how has that process been going to, to get that?
David Lawrence: So, we, it's such a great and important question. I mentioned the international community. This is going on all over the world. The Competition and Markets Authority in the UK, like, they built their own, like, digital analysis. Let's bring on technologist unit, and then hosted a conference for other agencies to talk about, like, how to do it.
We've come a really long way. So, take you back about five years. I was actually in another agency. Quick story, but kind of funny, the first time I encountered, like, a really big machine learning piece of evidence. So, a white paper comes in from the party trying to establish, like, a proposition that is relevant, based on, they had, like, millions of cell phone data pings. And they put them all into a giant model, and things occurred, and then out popped like a number favorable to their client.
And so, you know, my job as a regulator is like, I was like, well, can you give me the black box? So they did. They sent the hard drives. And there was an evening at that agency where everybody lost access to email for about 15 minutes because they tried to put those hard drives into the system. So we wound up having to hire a consultant and figure out a way. And it took us months to just peer inside that black box and kind of fumble around a little bit.
Alan Rozenshtein: This reminds me of that scene in Zoolander, the movie, right, where Derek goes the answer is inside the computer. I can just imagine someone sending you a hard drive.
David Lawrence: Oh yeah, you know-
Alan Rozenshtein: That's the model, that’s the black box. Enjoy!
David Lawrence: You're talking to the like, yeah, the consultant come in and they're like, oh, trust the black box. Number comes out good. Believe us. Yeah. So, we need to be able to examine these things. Right? At the same time, we can't be afraid of the fact that some of these exercises can be really helpful to answer questions that are important that we didn't used to have good answers to.
So we, AAG Kanter, the day he arrived, when he was talking through key elements of his vision, one of them was, we need to bring on experts beyond lawyers and economists. So we previously were staffed with just lawyers and economists. He changed the name of our economic analysis team to the expert analysis team and started hiring data scientists, machine learning experts, technologists, people who would help us with these issues. And I don't mean to suggest we've, like, solved it, but in the various cases I've just mentioned, like those people were kind of critical to at least start to peer inside of the black boxes.
Alan Rozenshtein: And the last question before we go to Q&A. So, we've been focusing for obvious reasons given that we were very lucky to have you come here on DOJ's role in competition and antitrust, but of course DOJ is not the only player here. At the federal level antitrust enforcement is split, in interesting ways, between DOJ and FTC.
And then of course, we haven't talked at all about the states which play an extremely important role in antitrust, often on their own initiative, often in collaboration with the federal government. And there's, you know, my home state of Minnesota, right, has I think one of the more aggressive, sort of state antitrust practices, which I think is kind of neat. So how do you think about DOJ's role? How is DOJ thinking about its role in relation to, you know, other partners or, I don't know, frenemies maybe? I don't, that's, I'm sure it's very complicated, all this jurisdiction negotiating.
David Lawrence: No, but I'll pick between those words. I mean, it's partners, right? You have multiple law enforcers. First of all, we're all very resource-constrained. And so having more hands working on issues, having situations where you can divide up even pieces of a case or something, so we work closely with our state attorneys general partners, like, jointly, and actually each of the cases I mentioned today had multiple state attorneys general, in most cases bipartisan, signed on and helping us as part of the investigative team.
So they're really helpful just from a, like, spreading the resources perspective, and the same with the FTC, right? So they take a significant portion of the cases, we take a significant portion of the cases. Then I also mentioned like this critical exercise, especially in emerging markets, of getting smart, being thoughtful. The kind of debate we can have with an enforcement partner you can be, you know, we'll have a confidentiality waiver in place. You can really talk issues through. And leverage each other's expertise in a way that I think is just really helpful and is good government.
Alan Rozenshtein: So, I invite folks from the audience to ask questions!
Audience Member 2: Hi, my name is Skylar. I'm a 1L at Georgetown Law.
David Lawrence: Hi, nice to meet you.
Audience Member 2: My question is about retail platforms and how their algorithm plays into the test of collusion. Because I was thinking they're kind of between oligopoly and information sharing. But on the other hand, they're not explicitly sharing their algorithmic recommendations to the sellers, but in a way they're kind of participating in this one system. And the delegation, they're not really, the sellers aren't really delegating their price choices to retail platforms either but they get so much information from the other sellers that are on the platform.
David Lawrence: Really great question. So the question is about retail platforms and the way they use their algorithms, as I'm gonna give the answer I've given a few times, which is these things are really specific. I wouldn't want to just give, like, a general answer. Talking about partnerships the FTC has a ongoing, it's in court now, lawsuit against Amazon that discusses a lot of very specific behaviors with the operation of the platform, so you might sort of look to that for more detail. Thank you.
Audience Member 3: Hi. So, I just want to go back a little bit on open source and because, if I understood you correctly, like open source is encouraging pro-competitiveness, in one side. And then we also have the risk of safety, as you also mentioned. And I want to know like your thoughts on, like, I don't know, like this type of tactics that might be going on about like maybe open source first and then closed later, you know, like I'm bringing, how that brings, or shrinks the market.
And in, in also in that same line, your thoughts on like how maybe having regulation are, also shrinking the market. If you closed you know, like if you put regulation on top of this type of industry where you are, in some sense, shrinking the market for open source type of business models in AI.
David Lawrence: Yeah, so a couple pieces there. Great question. I think that when we think about constructing regulation to the extent regulation is necessary. It's critical that regulatory agencies be thinking about competition and that they not be regulating in a way that erects barriers to entry and limits the competitive dynamic.
Actually, in this administration, President Biden put out an executive order early on calling for a whole of government approach to competition, and creating a competition council at which we and FTC and various federal agencies that have regulatory authorities, like, get together and focus on the competition side of this. Relatedly, actually, OIRA, this is getting into the regulatory weeds, but they have a circular that requires cost benefit analysis. And they added an additional, I forget if it's called a supplement or a report, specifically instructing agencies how they can examine the competitive implications of regulations.
This is incredibly important to do, because as long as there have been monopolies, there have been monopolies advocating to government to create regulations that would create barriers to entry and preserve their monopolies. And so, regulators just need to be on, really on the ball when they're examining regulatory frameworks to make sure that they're achieving necessary ends in the least anti-competitive way, in the way that sort of least protects just the dominant firms.
Alan Rozenshtein: I'm going to use moderator’s privilege to actually ask a follow up, because I'm going to ask the kind of converse or contrapositive, I always forget which of those words is correct, of your point that everyone has to think about competition. And my question is, what other than competition does antitrust have to think about?
So, one thing I liked about this question was that the student asked, so thank you for it, was this point that, you know, maybe open source is really good, and I'm, let's just hypothesize for a second, that open source is really good for competition, but on margin, it's not so good for AI safety, right? I'll be very clear, I'm not sure that's true, but let's just assume it's a plausible first approximation.
When antitrust thinks about, okay, what's it going to do? Does it say, okay, look, our job is competition. That's what we do, right? It's not our job in the first instance to think about safety. Like, there's an AI safety institute at NIST in Commerce. That's their job. Or, does antitrust, when it thinks about these issues, have to say, yeah, we do competition, but at the end of the day, we have to kind of do, we have to think of all of these considerations?
David Lawrence: Yeah. So, really great and important question, I'm going to get back into like antitrust doctrine. So, in most of our cases, like these monopolization cases, first we show why it's harmful to competition.
And at that point, all we're thinking about is competition. And then the defendants can try to establish that there are sort of legitimate business justifications for the conduct.
Alan Rozenshtein: Or maybe social justifications. So maybe it has to be closed because it's safer or something.
David Lawrence: So, if safety is legitimately part of the justification, I would think something like safety is really, you know, in many cases is going to be part of the competitive analysis too.
The question though is whether there are legitimate justifications or whether they’re protextual. And so, you know, that's a very fact bound analysis. I'll, you know, bring up our old friend, the AT&T divestiture. In the years leading up to that, you can look up the papers, monopolist AT&T advocated loudly and vociferously on the Hill, at the White House, and in the public sphere, that them maintaining their telephone monopoly was critical to maintaining our nation's security against Russia.
And that if this breakup happened, the Cold War was threatened. Now in that particular case, the Cold War went our way and the breakup did happen. But in the other cases, we're just going to look at the facts and really get into it, in order to determine whether the justifications are legitimate.
Alan Rozenshtein: I did not know, but that's great. I'm gonna go reread those. That must be fun to read in retrospect. Please.
Audience Member 4: I think one of the more frustrating sort of like setups that we see in terms of privacy and consumer protection is when a consumer is using a product not because they chose it but because like they work at a company or they go to school and like that's the email that you're supposed to use or like whatever product that they're supposed to use and they don't have a choice in it.
And so, a lot of times I think like the competition in that space can be misaligned in terms of like, it might, I don't know, like an email company might be competing based on like what the employer wants to do, which might not be privacy protective. And so like as an employee or like as a consumer, you don't get a choice in it. And you don't feel like the companies are competing to protect your privacy. And so I'm just wondering, like, what are the kind of, like, are there antitrust like frameworks that could help, or are there other solutions where, I don't know, do people have to get together and be like, this email sucks?
David Lawrence: There can be antitrust implications when these third parties are involved, and particularly if they're being paid to make the decision. So I mentioned like the Google search case. Where the, you know, the default search engine on everybody's phones is that Google is paying the phone company to make that happen, right? In, in terms of, you know, your specific email, I'm not sure, but these issues can arise. Thank you.
Audience Member 5: Hi. I wanted to go back to some of the discussion you're having on vertical integration in the AI supply chain. I think a lot of the discussion has focused on, like, the need for cloud computing and kind of all of these different inputs that are required to build foundation models in these kinds of AI systems.
I'm curious though, if you guys have been thinking about kind of like the other side of it, the distribution side. Because a lot of these, it seems like a lot of these companies that are, you know, very well resourced on the computing side also have a lot of other, you know, software products that, you know, these models could potentially be integrated into, and there seems to be a lot of opportunities for self-preferencing and that sort of thing. So I'd just be curious, like, from your perspective what sorts of behaviors are you guys looking out for?
David Lawrence: Outstanding question, and absolutely. So, I'm sure there's been discussion. Today, you have multiple layers in this AI stack, and all else equal, each layer would be trying to encourage competition in the corresponding layers because if there's a dominant firm in a corresponding layer, they're going to be like sapping the rents on either side.
But you can have contracts or mergers and acquisitions that sort of like pass the dominant firm's incentives to the other layers to sort of restrain that sort of competitive force and to limit competition. So, we would be looking to anywhere in the stack that somebody is operating as a gatekeeper that they have significant market power, monopoly power, and then asking the question of whether there are specific behaviors that are leveraging or maintaining it anti-competitive.
Audience Member 6: Hey, this has been so interesting! I litigate cases against Big Tech for product liability and distributor negligence. And I'm really interested in the idea of data sets that are not open source, but are actually obtained through the discovery process of litigation, and then rich resource parties then try to swoop in and get it. And what the regulation should be and the privacy issue should be around that.
I happen to be in possession of, like, 60,000 items, like, from a shuttered website that I litigated against. It's called Omegle. And like, a third party has tried to, like, has offered to purchase that. And it's a third party that's interested in child welfare for Big Tech. And they don't share with each other because they're competitors with each other but think that a data set should, would help all of them if it comes from a third party. But I think it's kind of unethical and there’re privacy implications because data sets have names and addresses and emails and trauma in these types of reports. So what do you think?
David Lawrence: So, in, in my world, because we're the federal government, we have a lot of restrictions on the sorts of things. So I don't, one answer is I don't have to think a lot about that because we are very heavily restricted in that way. I, my instinct is like a case management order or the orders of the court might have something to say about when and whether that's happening, but the, I guess the details could be case-specific. Thank you.
Audience Member 7: I'm with Encode Justice. We're a youth advocacy organization focused on AI. One of our student volunteers is actually writing a brief on RealPages. So, I was sitting there editing it, and then you started talking about it. So I was like, oh, this is cool.
David Lawrence: Oh, wonderful. Nice to meet you.
Audience Member 7: And I think the crux of that case is that there's basically a perfect intermediary that's getting all of the information from all of the different parties. And so, you know, X party and Y party aren't necessarily seeing each other's private information, but this intermediary is. It's like, if all of the 10 gas stations had a guy named Bob that was like getting all of their rental information and their insurance, is there, you know, existing context for that with, you know, an individual like Bob that's an actual human in the past, or is this a completely novel thing, not just because it's machines, but because we haven't had an intermediary in that way?
David Lawrence: So, there are, I don't know if they were named Bob. But there have been a number of cases over the years, like if multiple competitors agree to have somebody else, like, set the prices market-wide, that ringleader and the agreeing parties could be held responsible.
And actually, perhaps an analogous example relates to e-book pricing. So the department brought a case when the iPad launched. Apple worked with the, we alleged, and the court agreed, that Apple worked with a number of publishers to set a new pricing protocol market-wide for the sale of e-books that was less favorable for consumers than what was already happening off of the iPad, and so we challenged that and with all of the participants around the conspiracy as well.
Audience Member 7: Interesting. Thanks.
David Lawrence: Thank you.
Alan Rozenshtein: Well, I think this is a good place to end it. I hope you'll all join me by thanking David for an extremely interesting conversation.
David Lawrence: Thank you.
Alan Rozenshtein: The Lawfare Podcast is produced in cooperation with the Brookings Institution. You can get ad-free versions of this and other Lawfare podcasts by becoming a Lawfare material supporter through our website, lawfaremedia.org/support. You'll also get access to special events and other content available only to our supporters. Please rate and review us wherever you get your podcasts. Look out for our other podcasts including Rational Security, Chatter, Allies, and the Aftermath, our latest Lawfare Presents podcast series on the government's response to January 6th. Check out our written work at lawfaremedia.org. The podcast is edited by Jen Patja, and your audio engineers this episode were the good people at the Georgetown Institute for Law and Technology. Our theme song is from Alibi Music. As always, thank you for listening.