How Policymakers Can Build a Better Doomsday Clock
Probabilistic forecasting is a powerful tool for anticipating global catastrophic risks.
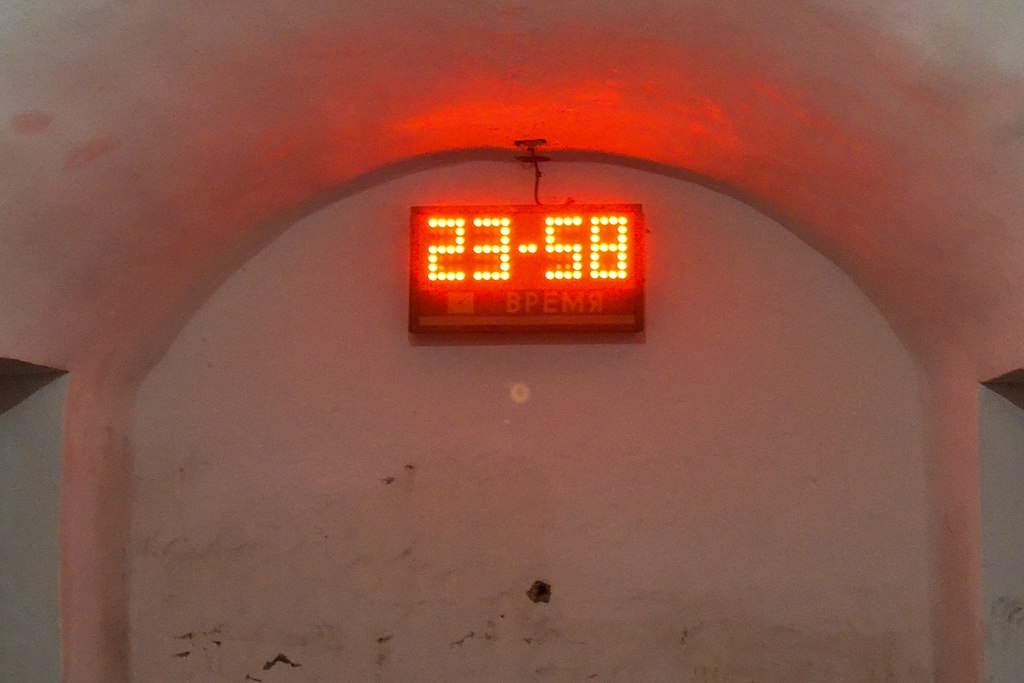
Published by The Lawfare Institute
in Cooperation With
Vladimir Putin’s nuclear saber-rattling during the invasion of Ukraine has many people wondering: How likely is this crisis to escalate to nuclear war? The Bulletin of the Atomic Scientists’s famous Doomsday Clock has a simple and frightening answer: Humanity is mere seconds away from “midnight,” and the risk of nuclear war is unacceptably high.
But how high is it, exactly?
The Doomsday Clock can’t give an answer to that—seconds don’t translate well to probabilities—and it’s not designed to, as a public communications and awareness-raising tool. Perhaps the question is fundamentally unanswerable. But new techniques in the nascent science of forecasting suggest that better tools can be built that give early-warning indicators of global catastrophic risks. If policymakers, researchers and funders embrace the possibilities of crowdsourced probabilistic forecasting as a new kind of Doomsday Clock, it might be possible to better see—and avert—the next crisis on the horizon.
***
Expert judgments about such critical issues, as explained in Lawfare last year, often rely on vague, unquantified and untestable predictions that make it impossible to assess their accuracy. Probabilistic forecasting can help. By “keeping score” of quantified expressions of belief about the future, forecasters can understand their own performance more clearly and see, for example, whether things they say are “80 percent likely” do, in fact, occur 80 percent of the time.
These techniques on probabilistic crowdsourced forecasting took off with the research of Philip Tetlock, Barbara Mellers and others in tournaments organized by the Intelligence Advanced Research Projects Activity (IARPA). By identifying and training teams of “superforecasters,” the researchers of the now-famous Good Judgment Project were able to make stunningly accurate predictions about geopolitical events, and to show that the ability to see the future can be trained and improved.
To do this, superforecasters often start by asking basic questions like “how often do countries usually invade one another?” This gives them the base rate of a broad class of events. From there, they will update their beliefs based on additional information, frequently adjust their forecasts up or down, and—when the time comes to score their performance—honestly assess what went wrong. Training in these simple techniques, putting top forecasters in teams and using aggregation algorithms to optimize the “wisdom of the crowds,” are powerful techniques, as these experiments have shown. In the IARPA tournaments, forecasters—many of them not subject-matter experts—even outperformed seasoned intelligence analysts with access to classified information.
For a while, the U.S. intelligence community took note and launched efforts to incorporate crowdsourced forecasting tools into the analytic and policy processes. Unfortunately, these efforts stalled during the Trump years, and it seemed that government forecasting was doomed.
Then, the U.K. launched Cosmic Bazaar, a crowdsourced forecasting tool that recruits participants from across the British civil service, not just the intelligence community, to make forecasts on events around the world. The initiative has had some success. For example, it helped senior policymakers pay greater attention to a growing terrorist threat in Mozambique; had the forecasters not pointed to the threat, the government might have missed it.
Similarly, as the coronavirus pandemic showed the urgent need for better disease surveillance and forecasting, others advocated for the U.S. to reimplement geopolitical forecasting. (Full disclosure: I was involved with organizing a conference and a working group and publishing a report and articles related to forecasting implementation.)
Recently, the U.S. government has shown renewed interest in crowdsourced forecasting. Last fall, U.S. Deputy Director of National Intelligence Morgan Muir announced, “We are incorporating some of the insights that we’ve gotten ... into a new crowdsourced forecasting tool designed to be inclusive, open, and responsive to both analyst and policymaker desire for transparency and relevance.” Early this year, Foretell, a technology forecasting platform developed by Georgetown’s Center for Security and Emerging Technologies, moved to the Applied Research Laboratory for Intelligence and Security and “is now part of a larger forecasting program to support U.S. government policy decisions called INFER.”
Despite this momentum, however, much work remains to be done. Policymakers struggle to make sense of the outputs of forecasting platforms when they are expressed only as numerical probabilities. Moreover, the kinds of questions that are well suited to traditional probabilistic forecasting are narrow, relatively near term and precisely resolvable. By contrast, the kinds of questions that matter to policymakers—and the kinds of questions that would inform a forecasting “Doomsday Clock”—are broad, often long term and without clear resolution criteria.
The stakes are immense. For example, better forecasting techniques would allow policymakers to predict likely troop movements in Ukraine and other global hot spots; the probability of escalation from conventional war to nuclear exchange; the probability of yet another global pandemic; and the probabilities of the effects of potentially catastrophic climate change on human conflict, migration and resource use. Billions of dollars and lives are at stake.
Of course, not all of these questions may turn out to be answerable. Long-term forecasting is difficult and may even be impossible given the uncertainty of a world in which the flap of a butterfly’s wings in Brazil might set off a tornado in Texas. And traditional forecasting techniques decline in accuracy once the questions go several years into the future. Moreover, when events are rare or even unprecedented, creating base rates for forecasts can be impossible.
But it’s worth trying to improve these techniques, even marginally. Recent research by Tetlock and his collaborators Pavel Atanasov and Ezra Karger on improving judgments of existential risk outlines the key methodological challenges that need to be overcome, and describes possible solutions for a new discipline of “second-generation” forecasting. (Full disclosure: This work was funded in part by members of Founders Pledge, my current employer, and we continue to recommend the research as a high-impact funding opportunity.) Second-generation forecasting builds on the foundation of the first-generation forecasting work of the IARPA tournaments and the Good Judgment Project by using intersubjective, rather than objective, resolution criteria, allowing forecasters to tackle difficult “big questions,” including questions about global catastrophic risks.
Many of these new techniques rely on intersubjective measures of accuracy, such as using superforecasters to engage in “reciprocal scoring” of key metrics. This helps to provide immediate feedback on questions where resolution would otherwise be slow or impossible, like questions about the probability of nuclear war. For example, Tetlock and his co-authors show how reciprocal scoring can help assess the usefulness of “conditional trees,” clusters of interrelated questions that can help approximate big picture answers. By having panels of superforecasters assess the questions at each branch of the tree, researchers can calculate an evidence ratio to score the incisiveness of each question.
In other words, these second-generation forecasting tournaments may begin to open up the Doomsday Clock and help policymakers better understand how the many interlocking gears of geopolitics affect the risk of disaster. Only a fraction of the clock’s complex movement may be visible, and the empirical evidence on long-term forecasting remains scant. Nonetheless, given the stakes, even small improvements in near-term early-warning indicators could have immense benefits. As Tetlock and his co-authors write, “Even if cumulative information gains prove modest and are confined to a 1-to-5 year planning horizon, the expected value of lives saved would be massive.”
***
It will be months, if not years, before evidence from this research on second-generation forecasting will enable updates. Still, steps taken toward better evidence-based policy and risk mitigation through forecasting can be taken. Governments should continue the promising initial efforts on probabilistic forecasting, and those states that have not done so should consider the benefits of these techniques. International entities such as the World Health Organization ought to adopt the methods as well, to better anticipate transnational threats. A more accurate Doomsday Clock might be on its way. Everyone would be better off if policymakers are prepared to adopt it.